REDN: A Recursive Encoder-Decoder Network for Edge Detection
IEEE Access(2020)
摘要
In this paper, we introduce REDN: A Recursive Encoder-Decoder Network with Skip-Connections for edge detection in natural images. The proposed network is a novel integration of a Recursive Neural Network with an Encoder-Decoder architecture. The recursive network enables iterative refinement of the edges using a single network model. Adding skip-connections between encoder and decoder helps the gradients reach all the layers of a network more easily and allows information related to finer details in the early stage of the encoder to be fully utilized in the decoder. Based on our extensive experiments on popular boundary detection datasets including BSDS500 [1], NYUD [2] and Pascal Context [3], REDN significantly advances the state-of-the-art on edge detection regarding standard evaluation metrics such as Optimal Dataset Scale (ODS) F-measure, Optimal Image Scale (OIS) F-measure, and Average Precision (AP).
更多查看译文
关键词
Edge detection,deep learning,encoder-decoder network,recursive network
AI 理解论文
溯源树
样例
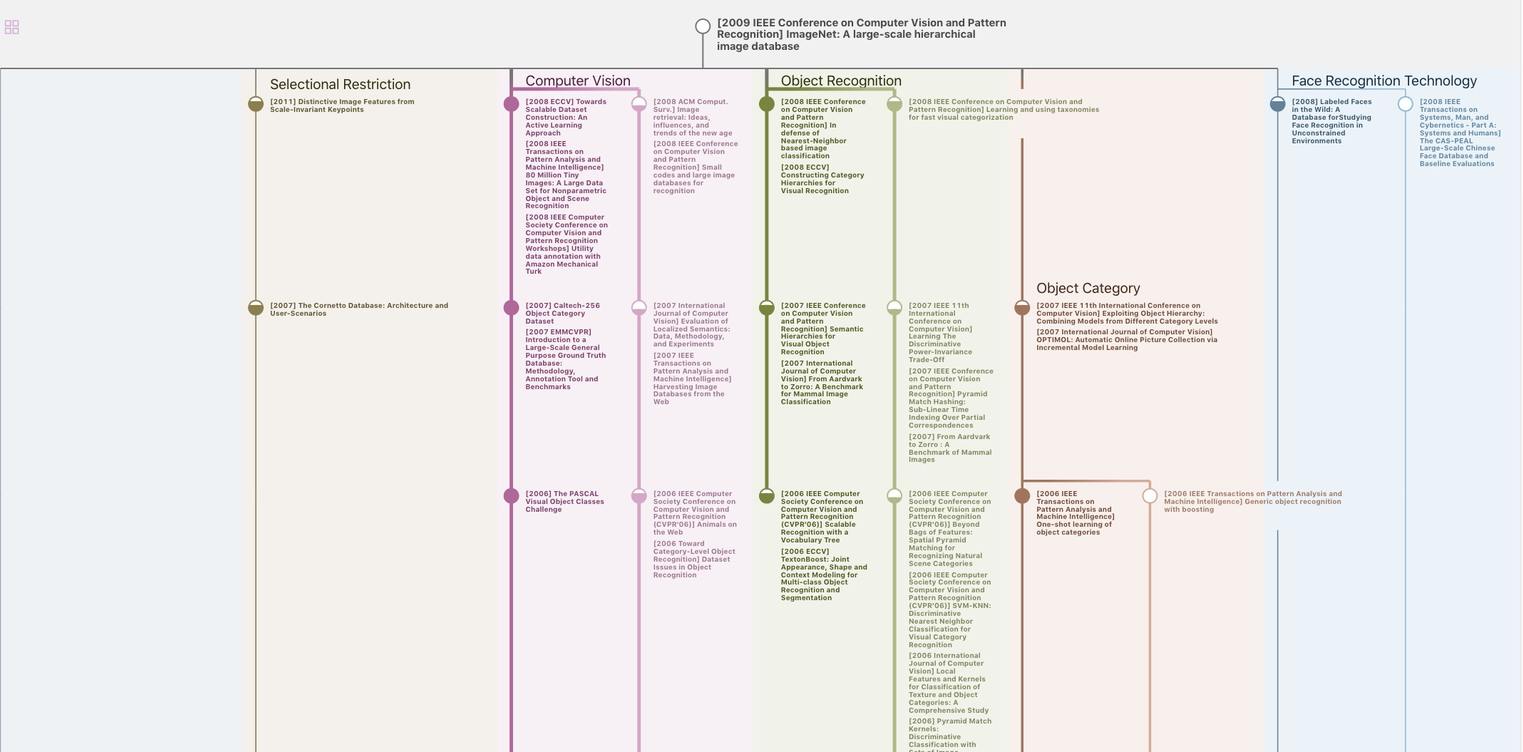
生成溯源树,研究论文发展脉络
Chat Paper
正在生成论文摘要