Systematic Bias Correction in Ocean Mesoscale Forecasting Using Machine Learning
Journal of Advances in Modeling Earth Systems(2023)
摘要
The ocean circulation is modulated by meandering currents and eddies. Forecasting their evolution is a key target of operational models, but their forecast skill remains limited. We propose a machine learning approach that improves the output of an ocean circulation model by learning and predicting its systematic biases. This method can be applied a priori to any region, and is tested in the Gulf of Mexico, where the Loop Current (LC) and the large anticyclonic eddies that detach from it are major forecasting targets. The LC dynamics are recurrent and lie on a low-dimensional dynamical attractor. Building upon the information gained analyzing this low dimensional attractor, we improve the representation of sea surface anomalies in model outputs through information from satellite altimeter data using a Sequence-to-Sequence model, which is a special class of Recurrent Neural Network. Building upon the HYCOM-NCODA analysis system, we deliver a correction to the forecast at the observation resolution. For at least 15 days the proposed method learns to forecast the systematic bias in the HYCOM-NCODA, outperforming persistence, and improving the forecast. This data-driven approach is fast and can be implemented as an added step to any dynamical hindcasting or forecasting model. It offers an interesting avenue for further developing hybrid modeling tools. In these tools, fundamental physical conservations are preserved through the integration of partial differential equations which obey them. In addition, the method highlights specific deficiencies of the hindcast system that deserve further investigation in the future.
更多查看译文
关键词
bias correction,machine learning,mesoscale forecast,Gulf of Mexico
AI 理解论文
溯源树
样例
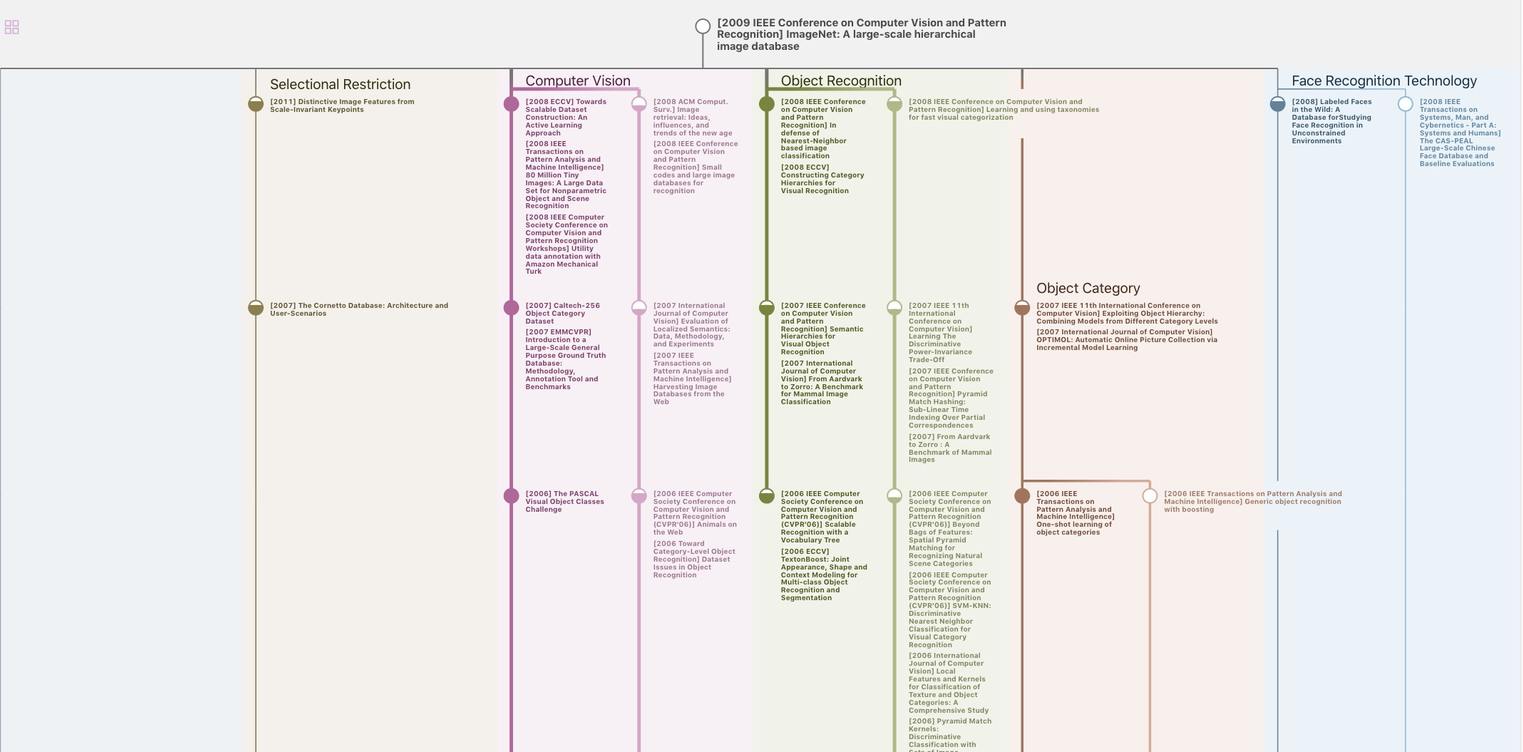
生成溯源树,研究论文发展脉络
Chat Paper
正在生成论文摘要