Lane Detection based on Generative Adversarial Networks and Attention Mechanism
2023 5th International Conference on Industrial Artificial Intelligence (IAI)(2023)
摘要
Lane detection plays a crucial role in the visual navigation system of intelligent vehicles. In lane detection, intelligent algorithms are capable of effectively processing images to accurately identify the desired objects. However, due to the complexity of real-world scenarios, objects to be detected in road images face challenges such as shape distortion, uneven illumination, shadow occlusion, and low resolution. Effectively extracting road features has become a research challenge. This study proposes a lane detection method based on generative adversarial networks (GANs) and attention mechanisms to address the severe imbalance between daytime and nighttime images in existing datasets. The method utilizes GANs for style transfer to augment the training dataset and designs a Coordinated Attention Detection Network (CADNet). By incorporating attention mechanisms into the residual modules of the backbone network, the model learns the correlation between feature channels, automatically calibrates attention in the channel dimension, and enhances the model’s robustness and generalization ability. Experimental evaluations on mainstream datasets and comparisons with state-of-the-art algorithms demonstrate that the proposed method effectively mitigates the impact of lighting and shadows on image detection, enabling more accurate lane detection in complex scenarios involving occlusion and low light conditions. The method exhibits higher accuracy, lower false detection rate, and missing detection rate, while significantly improving feature extraction performance.
更多查看译文
关键词
feature extraction,lane detection,information fusion,image enhancement,attention mechanism
AI 理解论文
溯源树
样例
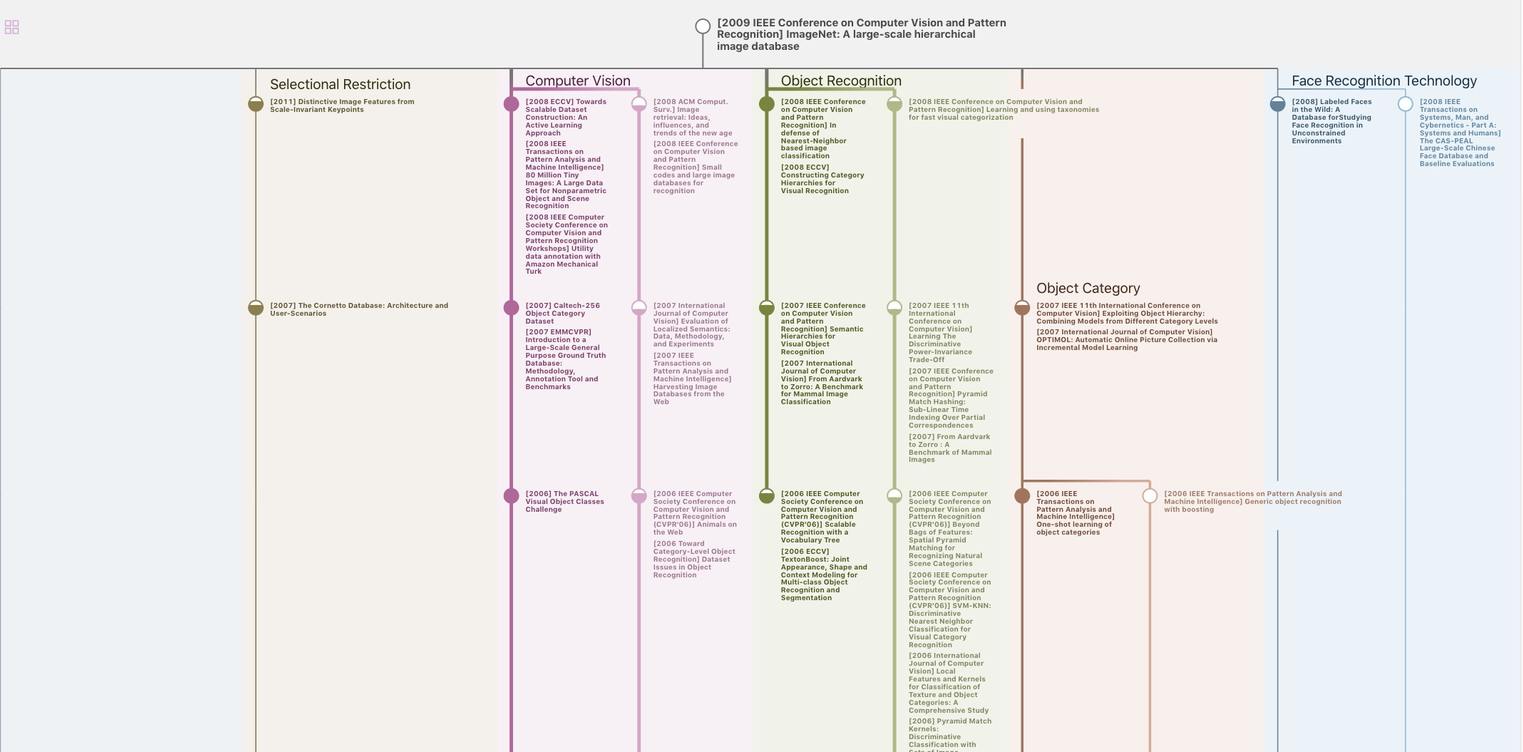
生成溯源树,研究论文发展脉络
Chat Paper
正在生成论文摘要