A Latent Feature-Based Multimodality Fusion Method for Theme Classification on Web Map Service
IEEE Access(2020)
摘要
Massive maps have been shared as Web Map Service (WMS) from various providers, which could be used to facilitate people's daily lives and support space analysis and management. The theme classification of maps could help users efficiently find maps and support theme-related applications. Traditionally, metadata is usually used in analyzing maps content, few papers use maps, especially legends. In fact, people usually considers metadata, maps and legends together to understand what maps tell, however, no study has tried to exploit how to combine them. This paper proposes a method to fuse them with the purpose of classifying map themes, named latent feature based multimodality fusion for theme classification (LFMF-TC). Firstly, a multimodal dataset is created that supports the supervised classification on map themes. Secondly, textual and visual features are designed for metadata, maps, and legends using some advanced techniques. Thirdly, a latent feature based fusion method is proposed to fuse the multimodal features on the feature level. Finally, a neural network classifier is implemented using supervised learning on the multimodal dataset. In addition, a web-based collaboration platform is developed to facilitate users in labeling multimodal samples through an interactive Graphical User Interface (GUI). Extensive experiments are designed and implemented, whose results prove that LFMF-TC could significantly improve the classification accuracy. In theory, the LFMF-TC could be used for other applications with few modifications.
更多查看译文
关键词
Cartography,machine learning,multimodality fusion,theme classification,web map service
AI 理解论文
溯源树
样例
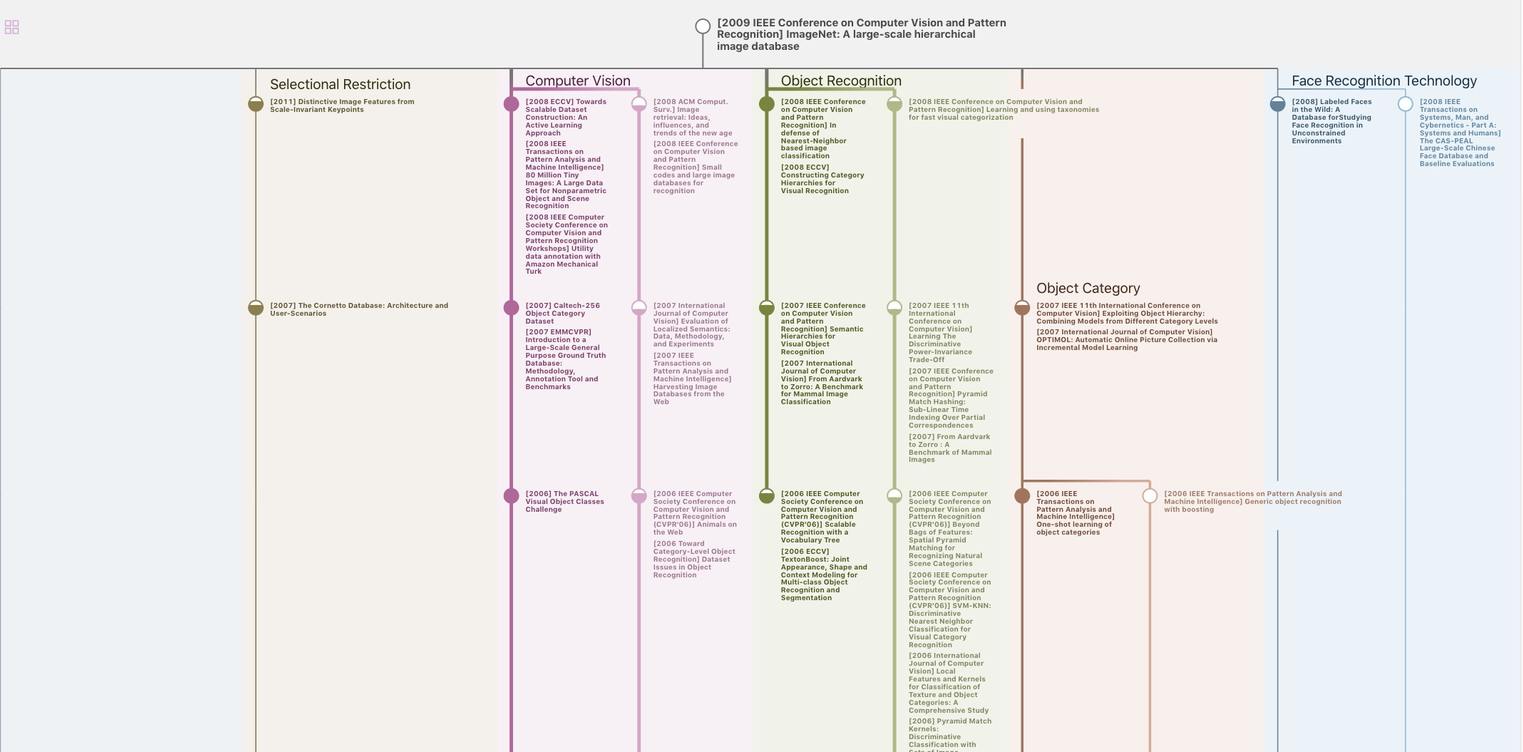
生成溯源树,研究论文发展脉络
Chat Paper
正在生成论文摘要