Predicting Perceived Visual and Cognitive Distractions of Drivers With Multimodal Features
IEEE Transactions on Intelligent Transportation Systems(2015)
摘要
A driver's behaviors can be affected by visual, cognitive, auditory, and manual distractions. While it is important to identify the patterns associated with particular secondary tasks, it is more general and useful to define distraction modes that capture the general behaviors induced by various sources of distractions. By explicitly modeling the distinction between types of distractions, we can assess the detrimental effects induced by new in-vehicle technology. This study investigates drivers' behaviors associated with visual and cognitive distractions, both separately and jointly. External observers assessed the perceived cognitive and visual distractions from real-world driving recordings, showing high interevaluator agreement in both dimensions. The scores from the perceptual evaluation are used to define regression models with elastic net regularization and binary classifiers to separately estimate the cognitive and visual distraction levels. The analysis reveals multimodal features that are discriminative of cognitive and visual distractions. Furthermore, the study proposes a novel joint visual-cognitive distraction space to characterize driver behaviors. A data-driven clustering approach identifies four distraction modes that provide insights to better understand the deviation in driving behaviors induced by secondary tasks. Binary and multiclass recognition problems demonstrate the effectiveness of the proposed multimodal features to infer these distraction modes defined in the visual-cognitive space.
更多查看译文
关键词
binary recognition problems,human evaluation,binary classification,interevaluator agreement,cognition,driver cognitive distraction,pattern clustering,driver behavior characterization,driver visual distraction,driver perceived visual distraction prediction,joint visual-cognitive distraction space,human factors,auditory distractions,elastic net regularization,regression analysis,detrimental effects,pattern classification,data-driven clustering approach,regularized regression,perceptual evaluation,in-vehicle technology,multiclass recognition problems,graphical user interfaces,explicit modeling,real-world driving recordings,driver perceived cognitive distraction prediction,driver information systems,external observers,distraction modes,binary classifiers,secondary tasks,multimodal features,pattern identification,manual distractions,visualization,measurement,classification,vision
AI 理解论文
溯源树
样例
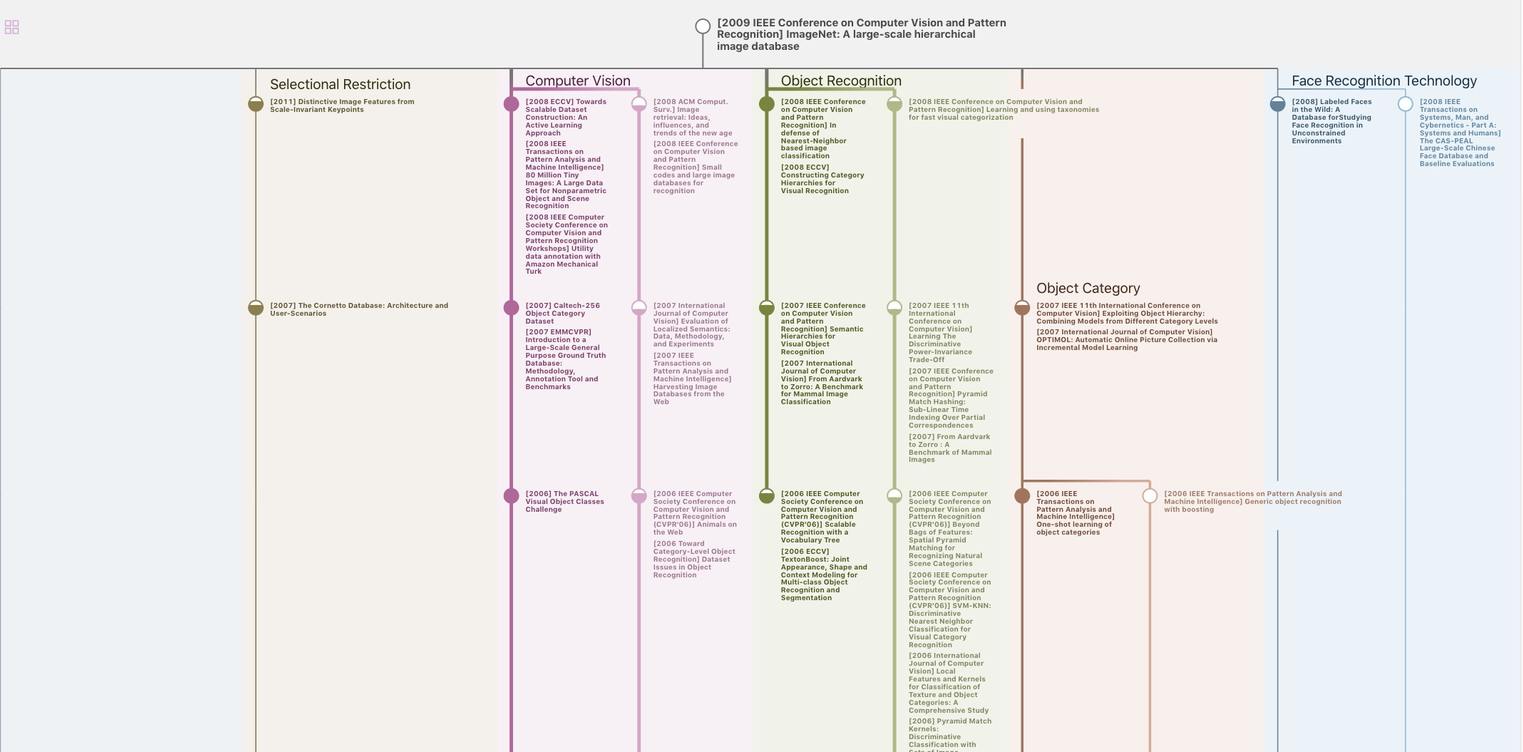
生成溯源树,研究论文发展脉络
Chat Paper
正在生成论文摘要