Machine learning-assisted determination of material chemical compositions: a study case on Ni-base superalloy
Science and Technology of Advanced Materials: Methods(2023)
摘要
ABSTRACTThe determination of chemical compositions of materials plays a paramount role in materials design and discovery. Optimization of such compositions can be a very expensive trial-and-error task, specially when the desired properties are very sensitive to the composition variations. As the number of elements and the variations of the possible composition values increase, the number of possible candidate materials increases exponentially. In this work, we present an efficient machine learning-assisted method to optimize the chemical compositions of materials for desired mechanical properties. The method utilizes a hybrid approach combining Monte Carlo tree search (MCTS) and an expansion policy neural network. The efficiency of this method was demonstrated by optimizing chemical compositions of a seven-element Ni-base superalloy (Al, Co, Cr, Mo, Nb, Ti, and Ni) to avoid the precipitation of the gamma-prime ([Formula: see text]) phase during cooling in the 3D additive manufacturing process. We were able to find Ni-base superalloys that could not be found by trial-and-error search or by using human experience.
更多查看译文
关键词
Chemical composition optimization,machine learning,Monte Carlo tree search,neural network,Ni-base superalloy
AI 理解论文
溯源树
样例
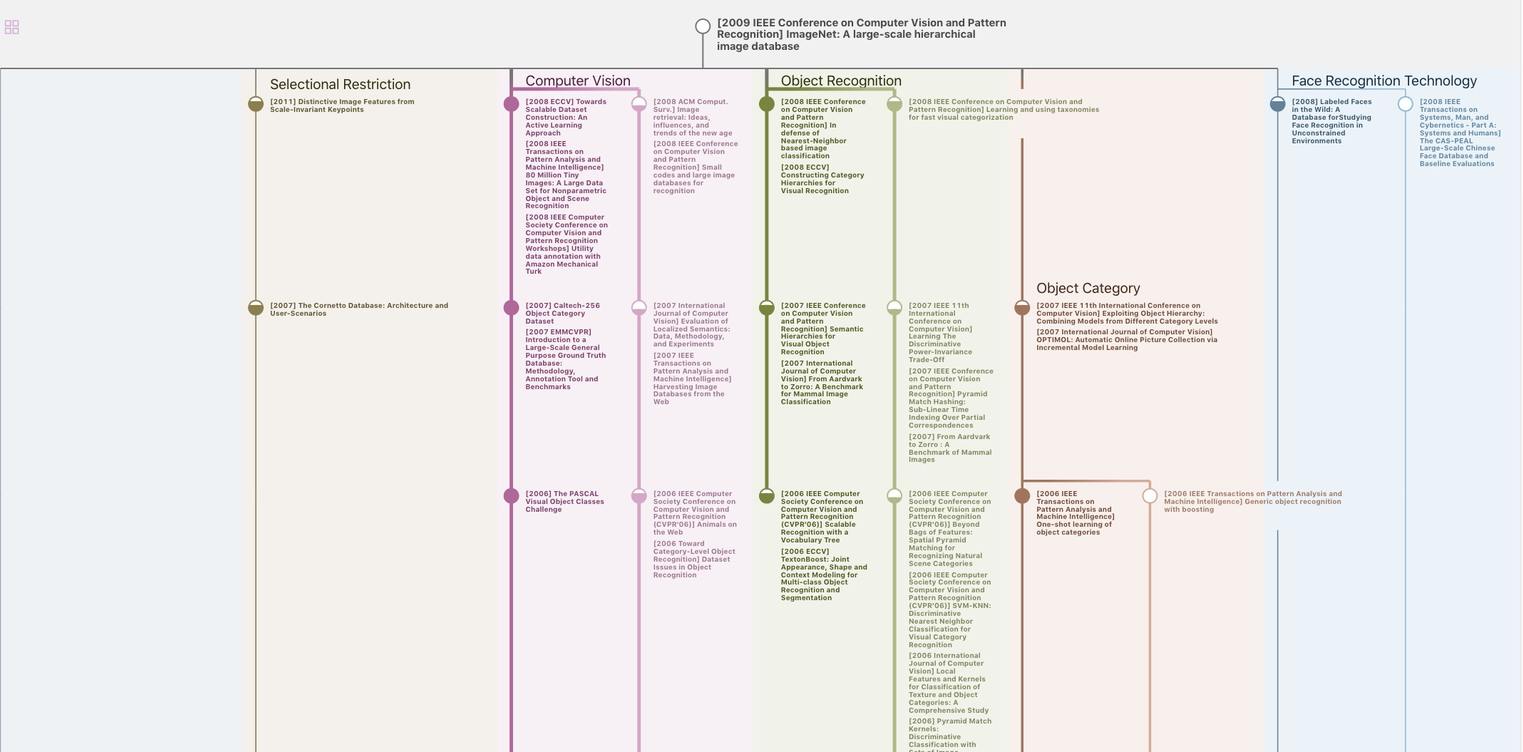
生成溯源树,研究论文发展脉络
Chat Paper
正在生成论文摘要