Convergence Analysis for Learning Orthonormal Deep Linear Neural Networks
IEEE SIGNAL PROCESSING LETTERS(2024)
摘要
Enforcing orthonormal or isometric property for the weight matrices has been shown to enhance the training of deep neural networks by mitigating gradient exploding/vanishing and increasing the robustness of the learned networks. However, despite its practical performance, the theoretical analysis of orthonormality in neural networks is still lacking; for example, how orthonormality affects the convergence of the training process. In this letter, we aim to bridge this gap by providing convergence analysis for training orthonormal deep linear neural networks. Specifically, we show that Riemannian gradient descent with an appropriate initialization converges at a linear rate for training orthonormal deep linear neural networks with a class of loss functions. Unlike existing works that enforce orthonormal weight matrices for all the layers, our approach excludes this requirement for one layer, which is crucial to establish the convergence guarantee. Our results shed light on how increasing the number of hidden layers can impact the convergence speed. Experimental results validate our theoretical analysis.
更多查看译文
关键词
Convergence analysis,deep neural networks,orthonormal structure,Riemannian optimization
AI 理解论文
溯源树
样例
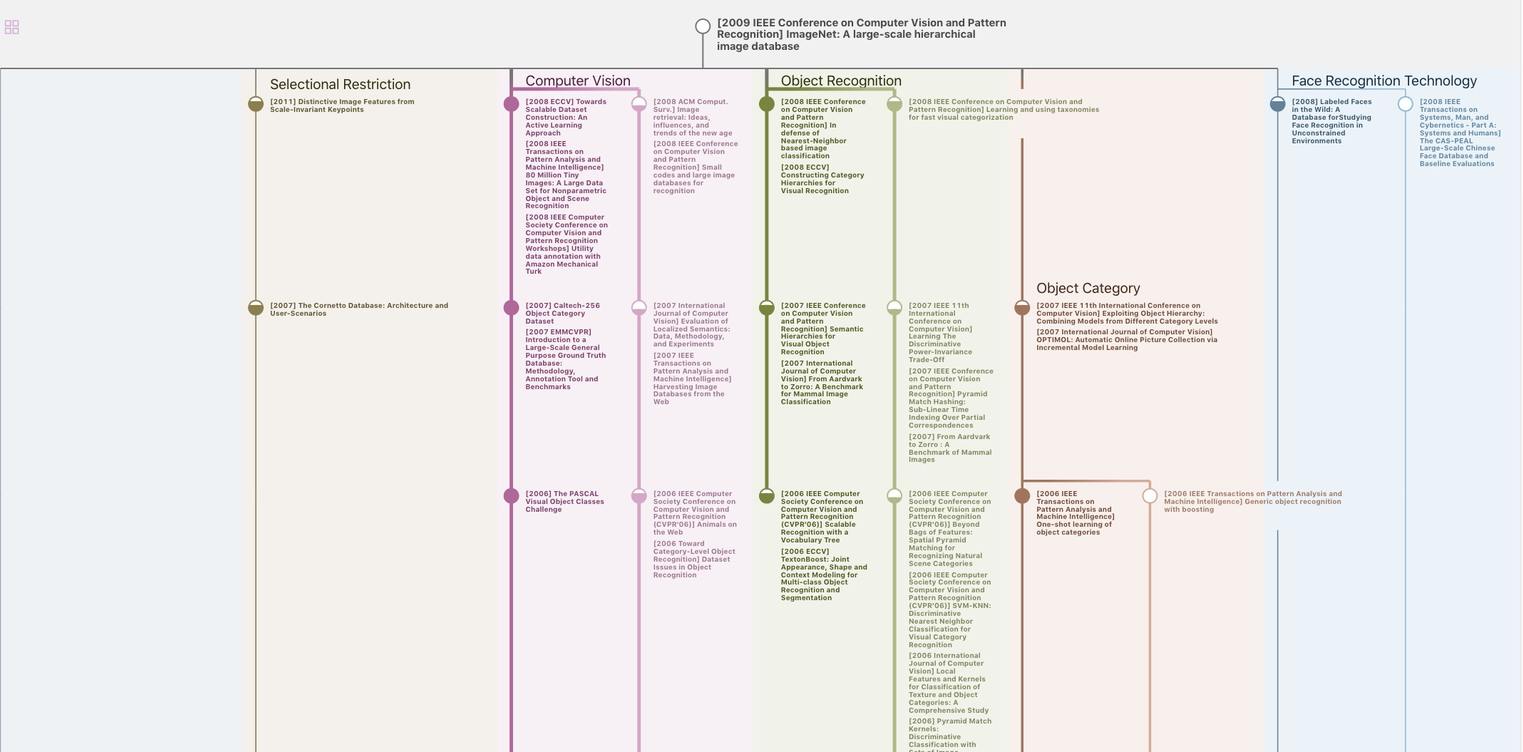
生成溯源树,研究论文发展脉络
Chat Paper
正在生成论文摘要