Deciphering and integrating invariants for neural operator learning with various physical mechanisms
NATIONAL SCIENCE REVIEW(2024)
摘要
Neural operators have been explored as surrogate models for simulating physical systems to overcome the limitations of traditional partial differential equation (PDE) solvers. However, most existing operator learning methods assume that the data originate from a single physical mechanism, limiting their applicability and performance in more realistic scenarios. To this end, we propose the physical invariant attention neural operator (PIANO) to decipher and integrate the physical invariants for operator learning from the PDE series with various physical mechanisms. PIANO employs self-supervised learning to extract physical knowledge and attention mechanisms to integrate them into dynamic convolutional layers. Compared to existing techniques, PIANO can reduce the relative error by 13.6%-82.2% on PDE forecasting tasks across varying coefficients, forces or boundary conditions. Additionally, varied downstream tasks reveal that the PI embeddings deciphered by PIANO align well with the underlying invariants in the PDE systems, verifying the physical significance of PIANO. PIANO: a new operator learning framework that deciphers and incorporates invariants from the PDE series via self-supervised learning and attention technique, achieving superior performance in scenarios with various physical mechanisms.
更多查看译文
关键词
neural operator,PDE solver,contrastive learning,physical invariants
AI 理解论文
溯源树
样例
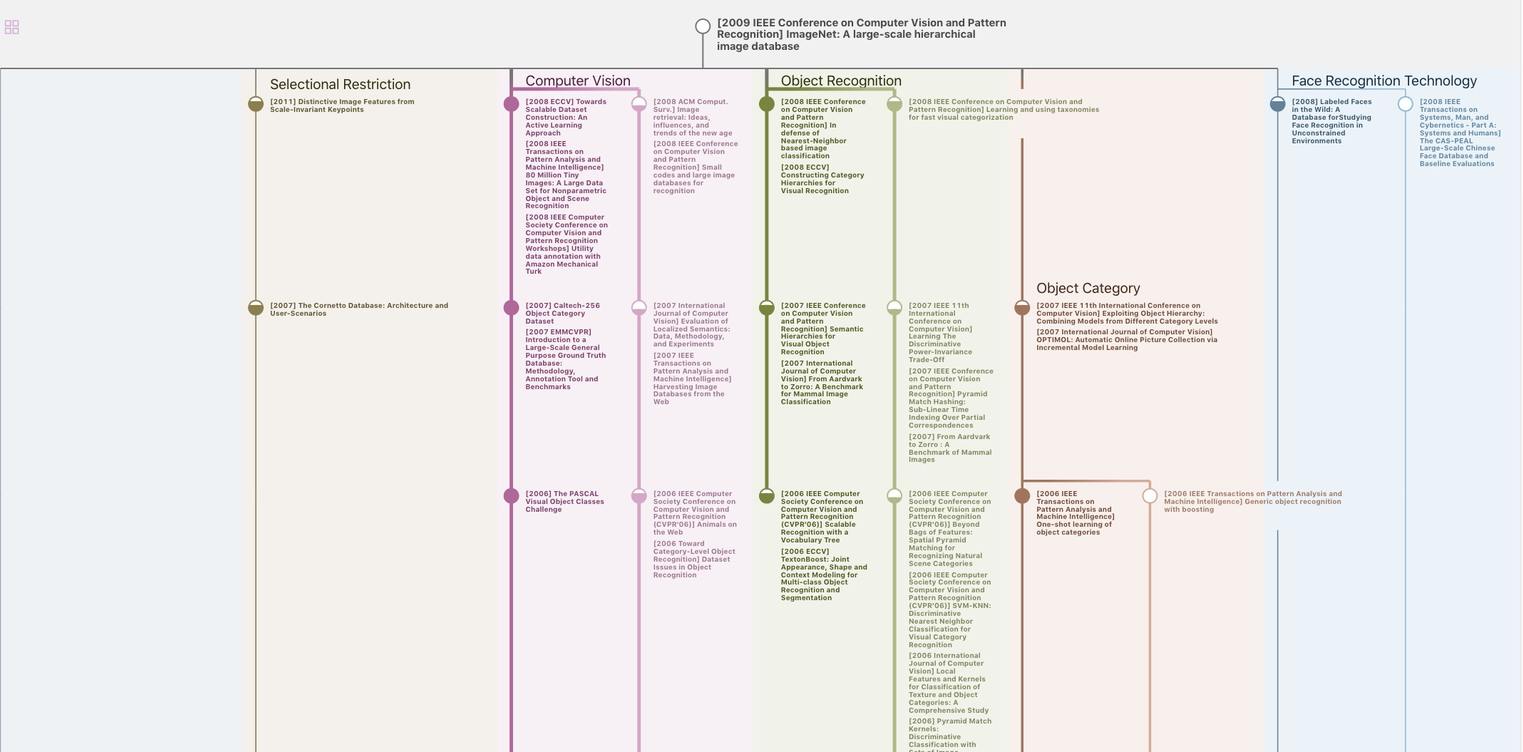
生成溯源树,研究论文发展脉络
Chat Paper
正在生成论文摘要