Federated Learning Assisted Distributed Energy Optimization.
CoRR(2023)
Abstract
The increased penetration of distributed energy resources and the adoption of
sensing and control technologies are driving the transition from our current
centralized electric grid to a distributed system controlled by multiple
entities (agents). The Transactive Energy Community (TEC) serves as an
established example of this transition. Distributed energy management
approaches can effectively address the scalability, resilience, and privacy
requirements of the evolving grid. In this context, the accuracy of agents'
estimations becomes crucial for the performance of distributed and multi-agent
decision-making paradigms. This paper specifically focuses on integrating
Federated Learning (FL) with the multi-agent energy management procedure. FL is
utilized to forecast agents' local energy generation and demand, aiming to
accelerate the convergence of the distributed decision-making process. To
enhance energy aggregation in TECs, we propose an FL-assisted distributed
Consensus + Innovations approach. The results demonstrate that employing FL
significantly reduces errors in predicting net power demand. The improved
forecast accuracy, in turn, introduces less error in the distributed
optimization process, thereby enhancing its convergence behavior.
MoreTranslated text
AI Read Science
Must-Reading Tree
Example
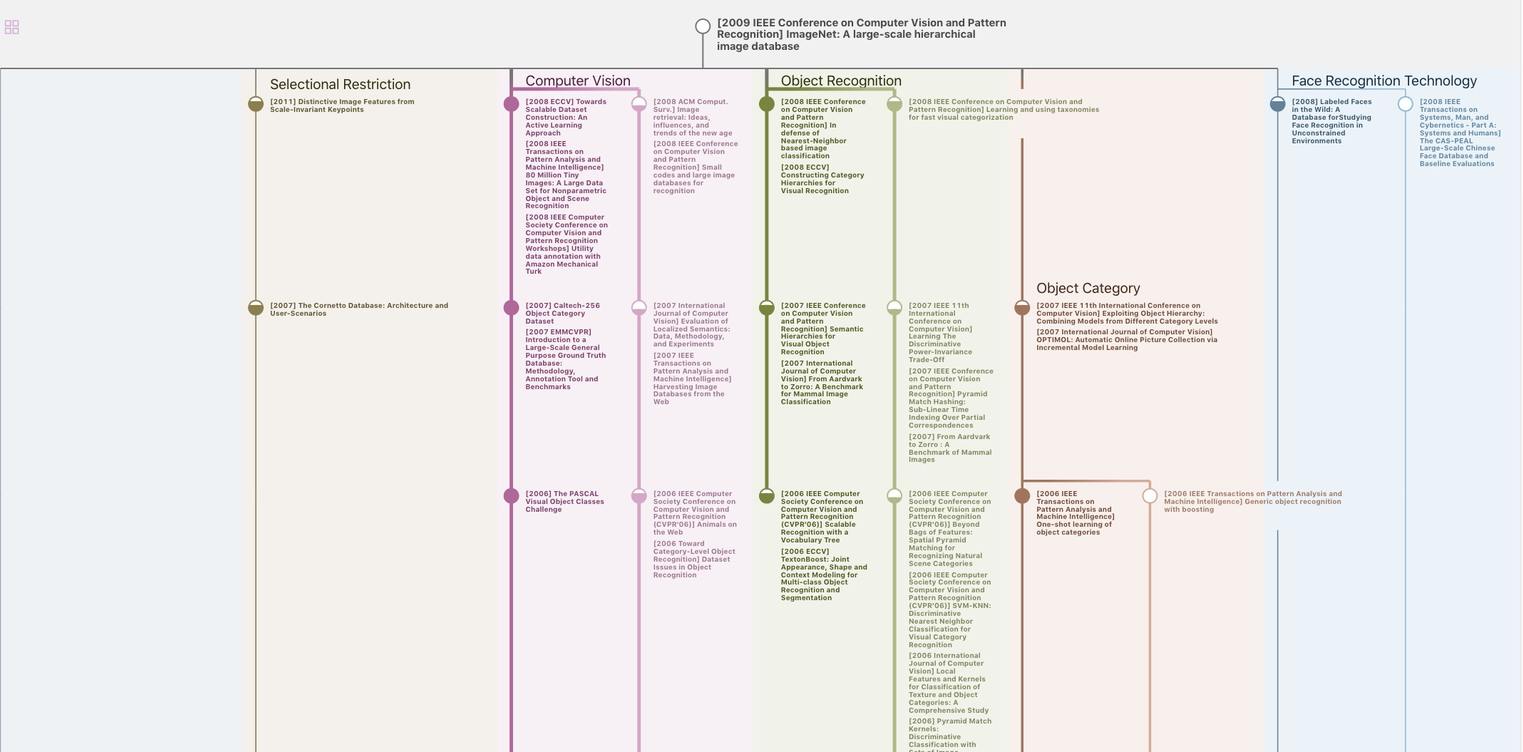
Generate MRT to find the research sequence of this paper
Chat Paper
Summary is being generated by the instructions you defined