Exploring percolation phase transition in the three-dimensional Ising model with machine learning
arxiv(2023)
Abstract
The percolation phase transition plays a significant role in understanding the Quantum Chromodynamics (QCD) phase diagram, particularly in the study of the Quark-gluon Plasma and the critical point in high energy nuclear physics. It is expected that the QCD critical point belongs to the three-dimensional (3D) Ising universality class. In this study, we explore the percolation phase transition within the 3D cubic Ising model by employing two machine learning algorithms: Principal Component Analysis and the Domain Adversarial Neural Network. Our findings reveal the effectiveness of machine learning techniques in distinguishing different phases during the percolation transition. Through the finite-size scaling analysis on the output of the neural networks, the critical temperature and a correlation length exponent in the geometrical percolation transition are extracted and compared to those in the thermal magnetization phase transition within the 3D Ising model. These results offer valuable insights to our understanding of QCD phase transitions in relativistic heavy-ion collisions.
MoreTranslated text
AI Read Science
Must-Reading Tree
Example
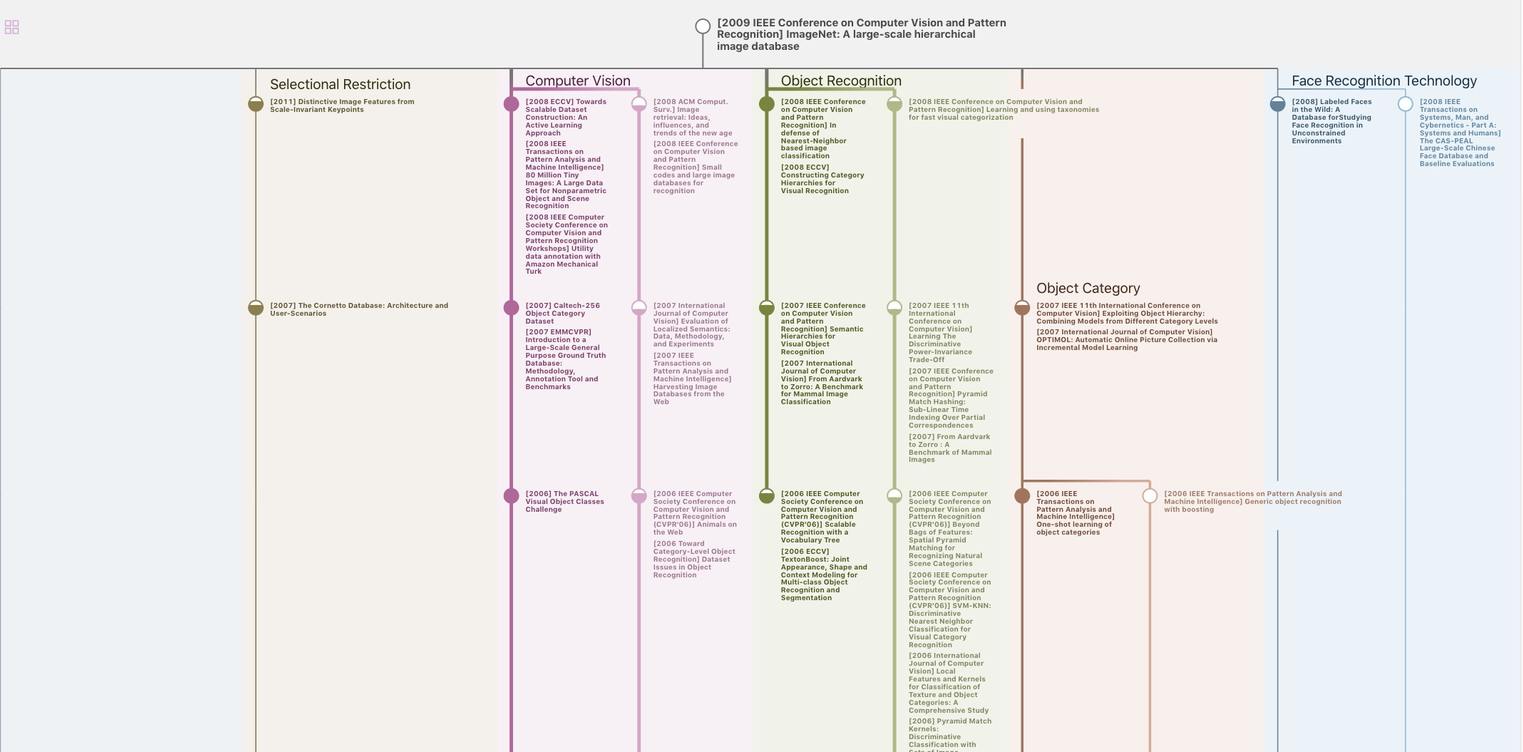
Generate MRT to find the research sequence of this paper
Chat Paper
Summary is being generated by the instructions you defined