Assessment and machine learning prediction of heavy metals fate in mining farmland assisted by Positive Matrix Factorization
JOURNAL OF ENVIRONMENTAL MANAGEMENT(2024)
摘要
The accurate pollutant prediction by Machine Learning (ML) is significant to efficient environmental monitoring and risk assessment. However, application of ML in soil is under studied. In this study, a Positive Matrix Factorization (PMF) assisted prediction method was developed with Support Vector Machine (SVM) and Random Forest (RF) for heavy metals (HMs) prediction in mining farmland. Principal Component Analysis (PCA) and Redundancy Analysis (RDA) were selected to pretreat data. Experiment results illustrated Cd was the main pollutant with heavy risks in the study area and Pb was easy to migrate. The method effects of HMs total concentration predicting were PMF > Simple > PCA > PCA - PMF, and RF predicted better than SVM. Data pretreatment by RDA prior inspection improved the model results. Characteristic HMs Tessier fractions prediction received good effects with average R value as 0.86. Risk classification prediction performed good in Cd, Cu, Ni and Zn, however, Pb showed weak effect by simple model. The best classifier method for Pb was PMF - RF method with relatively good effect (Area under ROC Curve = 0.896). Overall, our study suggested the combination between PMF and ML can assist the prediction of HMs in soil. Spatial weighted attribute of HMs can be provided by PMF.
更多查看译文
关键词
Machine learning,Positive Matrix Factorization,Soil heavy metals
AI 理解论文
溯源树
样例
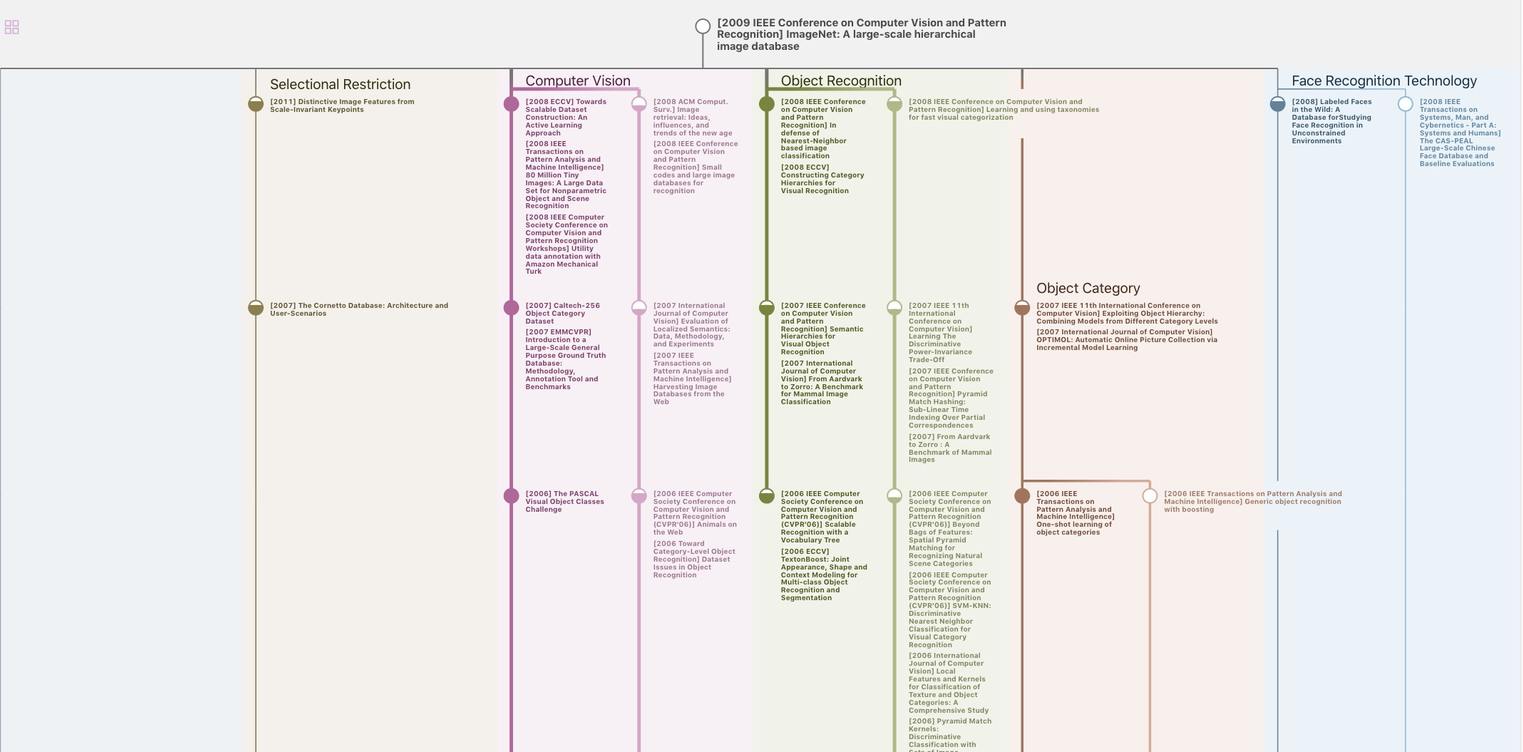
生成溯源树,研究论文发展脉络
Chat Paper
正在生成论文摘要