Towards Sequential Counterfactual Learning to Rank
ANNUAL INTERNATIONAL ACM SIGIR CONFERENCE ON RESEARCH AND DEVELOPMENT IN INFORMATION RETRIEVAL IN THE ASIA PACIFIC REGION, SIGIR-AP 2023(2023)
Abstract
Counterfactual evaluation plays a crucial role in learning-to-rank problems, as it addresses the discrepancy between the data logging policy and the policy being evaluated, due to the presence of presentation bias. Existing counterfactual methods, which are based on the empirical risk minimization framework, aim to evaluate the ability of a ranking policy to produce optimal results for a single query using implicit feedback from logged data. In real-world scenarios, however, users often reformulate their queries multiple times until they find what they are looking for and then provide a feedback signal. In such circumstances, current counterfactual approaches cannot assess a policy's effectiveness in delivering satisfactory results to the user over consecutive queries during a search session. Taking sequential search behavior into account, we propose the first counterfactual estimator for session ranking metrics under sequential position-based models and conduct preliminary experiments to shed light on further research in this direction.
MoreTranslated text
Key words
Information Retrieval,Counterfactual Learning to Rank
AI Read Science
Must-Reading Tree
Example
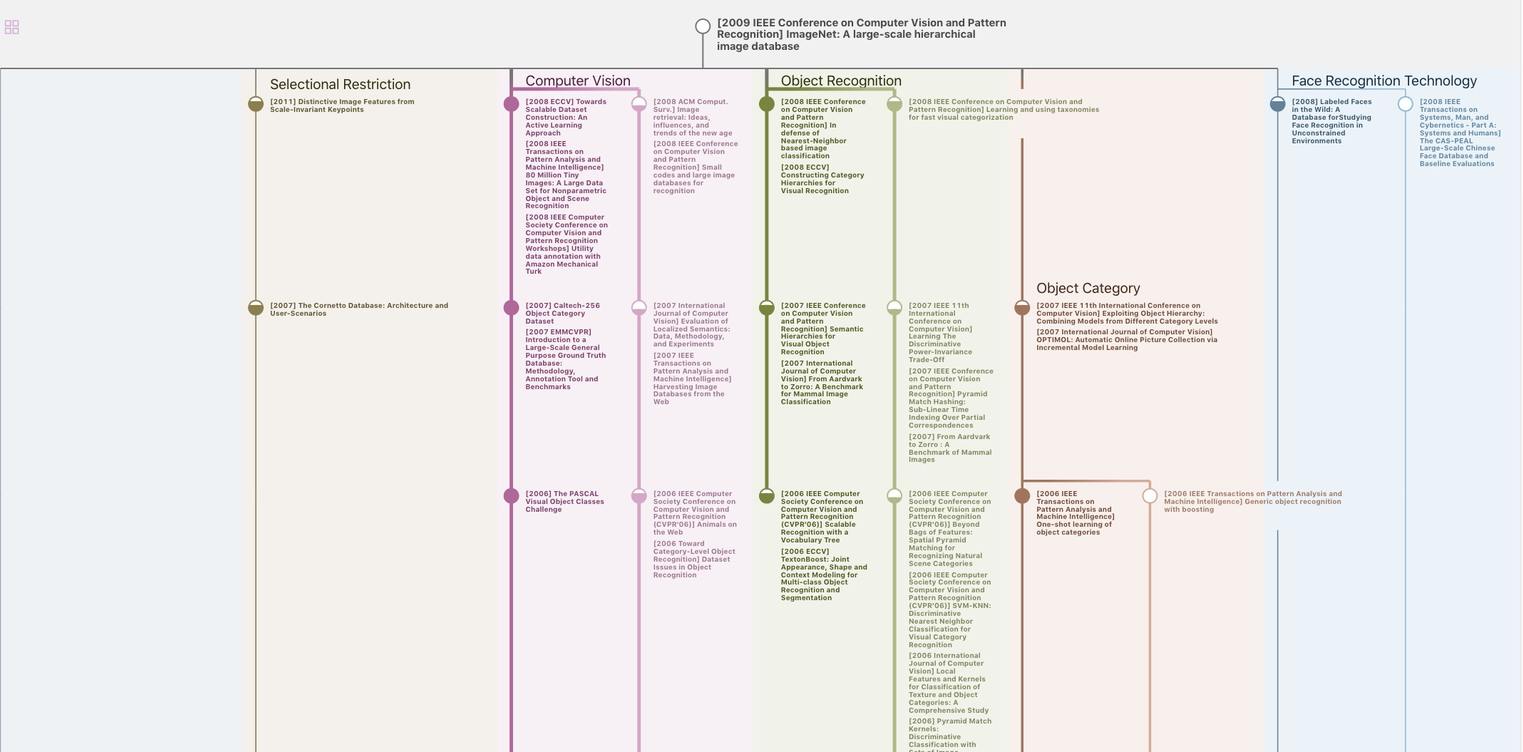
Generate MRT to find the research sequence of this paper
Chat Paper
Summary is being generated by the instructions you defined