Learning Primitive-Aware Discriminative Representations for Few-Shot Learning
NEURAL INFORMATION PROCESSING, ICONIP 2023, PT II(2024)
摘要
Few-shot Learning (FSL) aims to learn a classifier that can be easily adapted to recognize novel classes with only a few labeled examples. Recently, some works about FSL have yielded promising classification performance, where the image-level feature is used to calculate the similarity among samples for classification. However, the image-level feature ignores abundant fine-grained and structural information of objects that could be transferable and consistent between seen and unseen classes. How can humans easily identify novel classes with several samples? Some studies from cognitive science argue that humans recognize novel categories based on primitives. Although base and novel categories are non-overlapping, they share some primitives in common. Inspired by above research, we propose a Primitive Mining andReasoning Network (PMRN) to learn primitive-aware representations based onmetric-based FSL model. Concretely, we first add Self-supervision Jigsaw task (SSJ) for feature extractor parallelly, guiding the model encoding visual pattern corresponding to object parts into feature channels. Moreover, to mine discriminative representations, an Adaptive Channel Grouping (ACG) method is applied to cluster and weight spatially and semantically related visual patterns to generate a set of visual primitives. To further enhance the discriminability and transferability of primitives, we propose a visual primitive Correlation Reasoning Network (CRN) based on Graph Convolutional network to learn abundant structural information and internal correlation among primitives. Finally, a primitive-level metric is conducted for classification in a meta-task based on episodic training strategy. Extensive experiments show that our method achieves state-of-the-art results on miniImageNet and Caltech-UCSD Birds.
更多查看译文
关键词
Few-shot Learning,Visual Primitive,Graph Convolution,Episodic Training
AI 理解论文
溯源树
样例
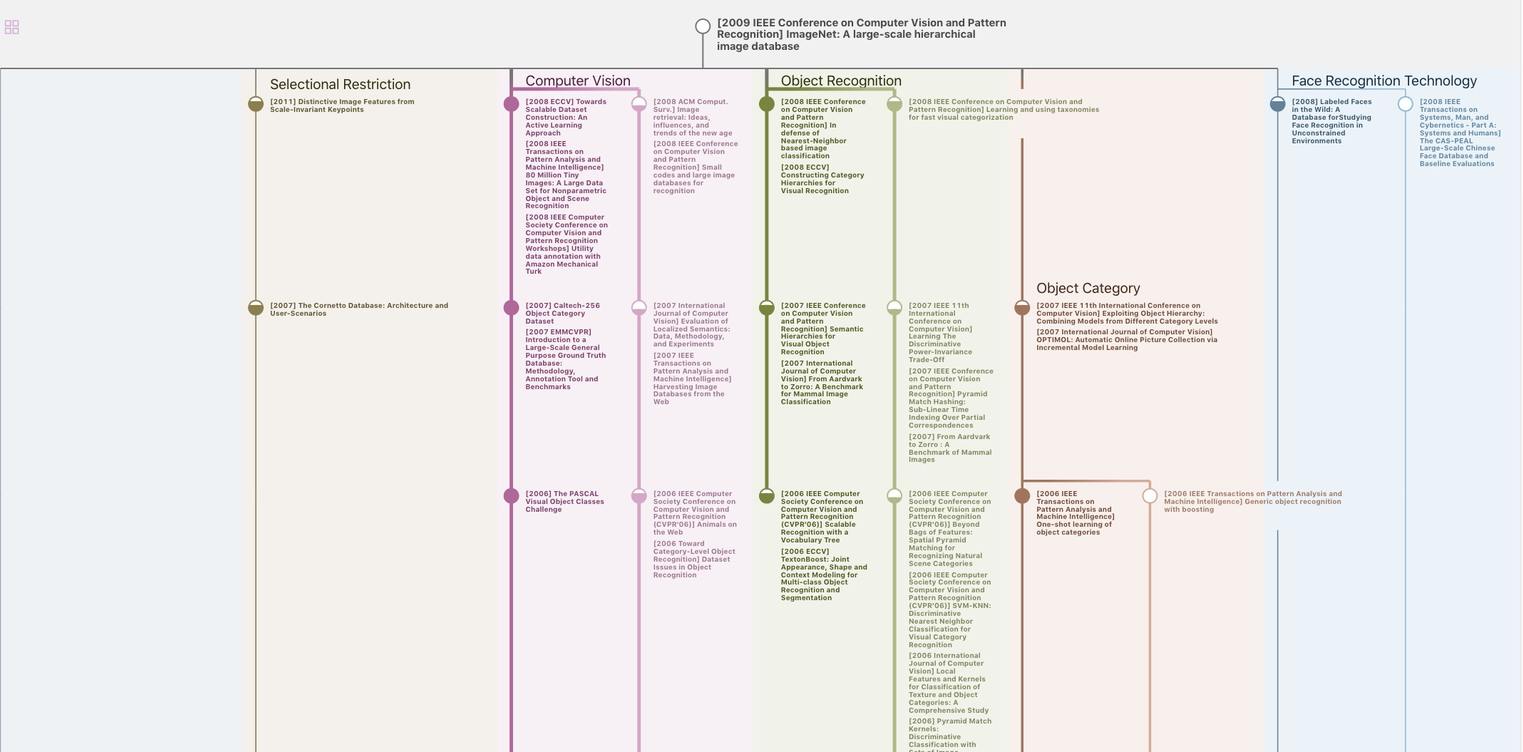
生成溯源树,研究论文发展脉络
Chat Paper
正在生成论文摘要