Brain-Inspired Binaural Sound Source Localization Method Based on Liquid State Machine
NEURAL INFORMATION PROCESSING, ICONIP 2023, PT III(2024)
摘要
Binaural Sound Source Localization (BSSL) is a remarkable topic in robot design and human hearing aid. A great number of algorithms flourished due to a leap in machine learning. However, prior approaches lack the ability to make a trade-off between parameter size and accuracy, which is a primary obstacle to their further implementation on resource-constrained devices. Spiking Neural Network (SNN)-based models have also emerged due to their inherent computing superiority over sparse event processing. Liquid State Machine (LSM) is a classic Spiking Recurrent Neural Network (SRNN) which has the natural potential of processing spatiotemporal information. LSM has been proved advantageous on numerous tasks once proposed. Yet, to our best knowledge, it is the first proposed BSSL model based on LSM, and we name it BSSL-LSM. BSSL-LSM is lightweight with only 1.04M parameters, which is a considerable reduction compared to CNN (10.1M) and D-BPNN (2.23M) while maintaining comparable or even superior accuracy. Compared to SNN-IID, there is a 10% accuracy improvement for 10 degrees interval localization. To achieve better performance, we introduce Bayesian Optimization (BO) for hyperparameters searching and a novel soft label technique for better differentiating adjacent angles, which can be easily mirrored on related works. Project page: https://github.com/BSSL-LSM.
更多查看译文
关键词
Binaural sound source Localization,Spiking neural network,Liquid state machine,Bayesian optimization
AI 理解论文
溯源树
样例
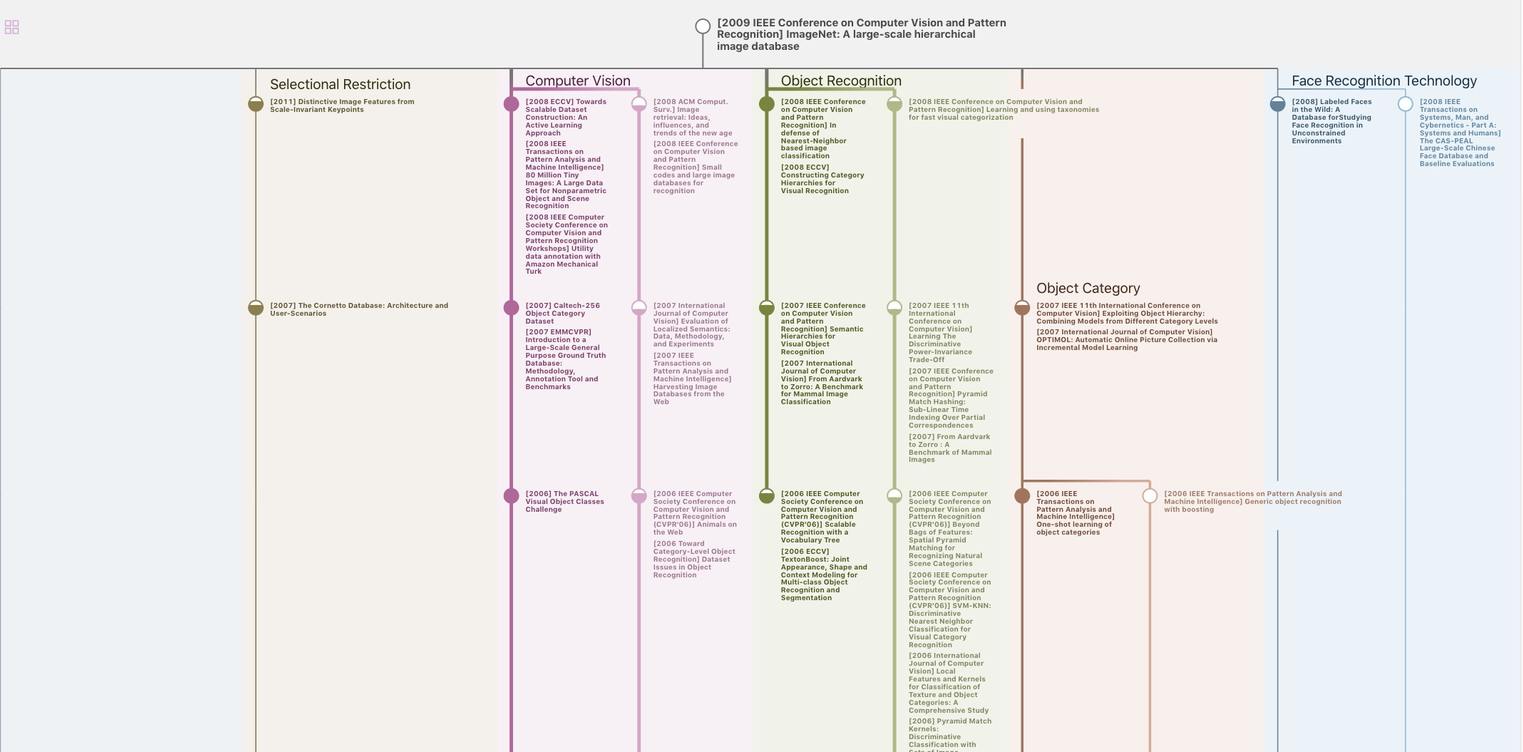
生成溯源树,研究论文发展脉络
Chat Paper
正在生成论文摘要