Effi-Seg: Rethinking EfficientNet Architecture for Real-Time Semantic Segmentation
NEURAL INFORMATION PROCESSING, ICONIP 2023, PT V(2024)
Abstract
A popular strategy for designing a semantic segmentation model is to utilize a well-established pre-trained Deep Convolutional Neural Network (DCNN) as a feature extractor and replace the classification head with a decoder to generate segmented outputs. The advantage of this strategy is the ability to obtain a ready-made backbone with additional knowledge. However, there are several disadvantages, such as a lack of architectural knowledge, a significant semantic gap among the deep feature maps, and a lack of control over architectural changes to reduce memory overhead. To overcome these issues, we first study the complete architecture of EfficientNetV1 and EfficientNetV2, analyzing the architectural and performance gaps. Based on this analysis, we develop an efficient segmentation model called Effi-Seg by implementing several architectural changes to the backbone. This approach leads to better semantic segmentation results with improved efficiency. To enhance contextualization and achieve accurate object localization in the scene, we introduce a feature refinement module (FRM) and a semantic aggregation module (SAM) in the decoder. The complete segmentation network comprises only 1.49 million parameters and 8.4 GFLOPs. We evaluate the performance of the proposed model using three popular benchmarks, and it demonstrates highly competitive results on all three datasets while maintaining excellent efficiency.
MoreTranslated text
Key words
deep learning,deep convolutional neural networks,semantic segmentation,encoder-decoder and feature aggregation,real-time applications
AI Read Science
Must-Reading Tree
Example
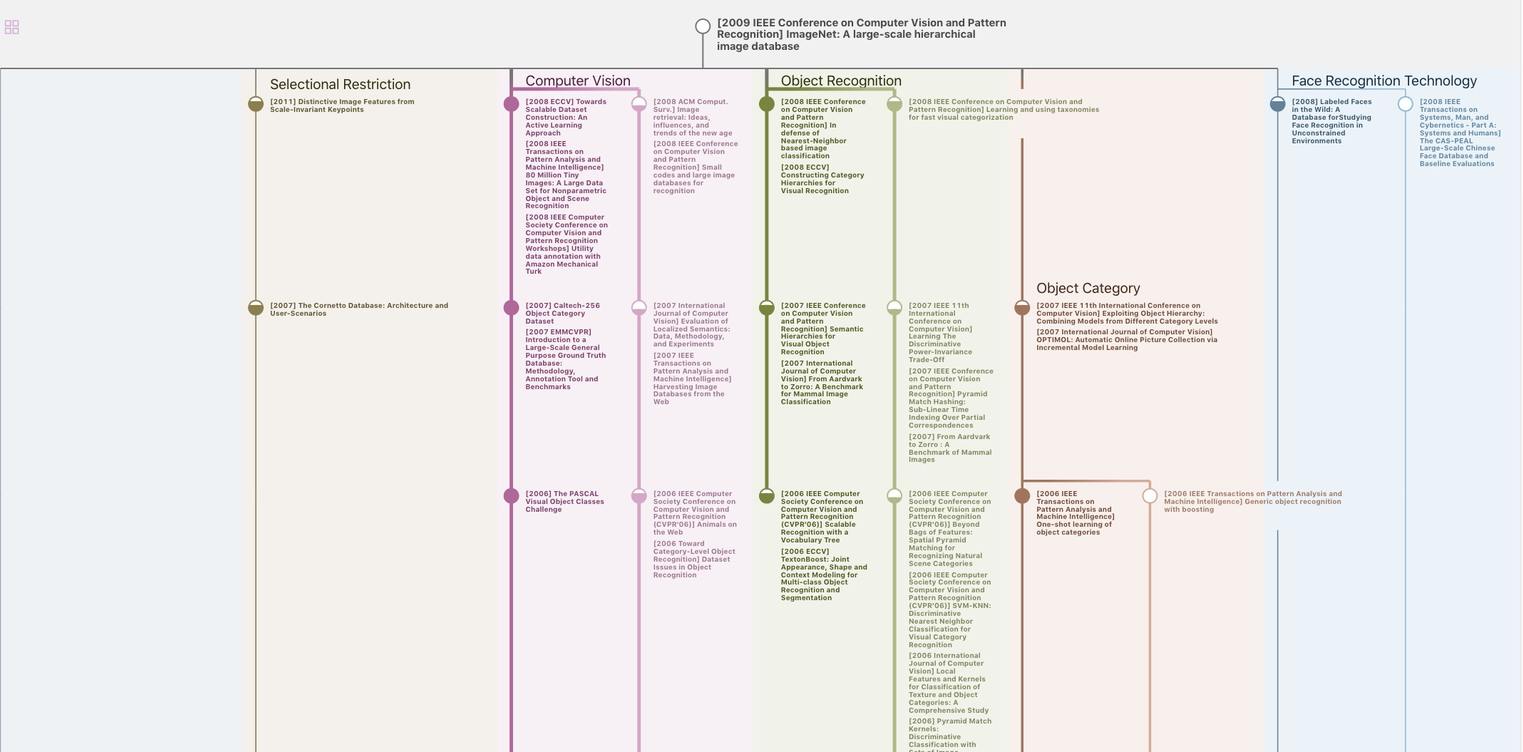
Generate MRT to find the research sequence of this paper
Chat Paper
Summary is being generated by the instructions you defined