Multi-view Contrastive Learning for Knowledge-Aware Recommendation
NEURAL INFORMATION PROCESSING, ICONIP 2023, PT V(2024)
Abstract
Knowledge-aware recommendation has attracted increasing attention due to its wide application in alleviating data-sparse and cold-start, but the real-world knowledge graph (KG) contains many noises from irrelevant entities. Recently, contrastive learning, a self-supervised learning (SSL) method, has shown excellent anti-noise performance in recommendation task. However, the inconsistency between the use of noisy embeddings in SSL tasks and the original embeddings in recommendation tasks limits the model's ability. We propose a Multi-view Contrastive learning for Knowledge-aware Recommendation framework (MCKR) to solve the above problems. To remove inconsistencies, MCKR unifies the input of SSL and recommendation tasks and learns more representations from the contrastive learning method. To alleviate the noises from irrelevant entities, MCKR preprocesses the KG triples according to the type and randomly perturbs of graph structure with different weights. Then, a novel distance-based graph convolutional network is proposed to learn more reliable entity information in KG. Extensive experiments on three popular benchmark datasets present that our approach achieves state-of-the-art. Further analysis shows that MCKR also performs well in reducing data noise.
MoreTranslated text
Key words
Recommender System,Knowledge Graph,Contrastive Learning,Self-Supervised Learning,Graph Convolutional Network
AI Read Science
Must-Reading Tree
Example
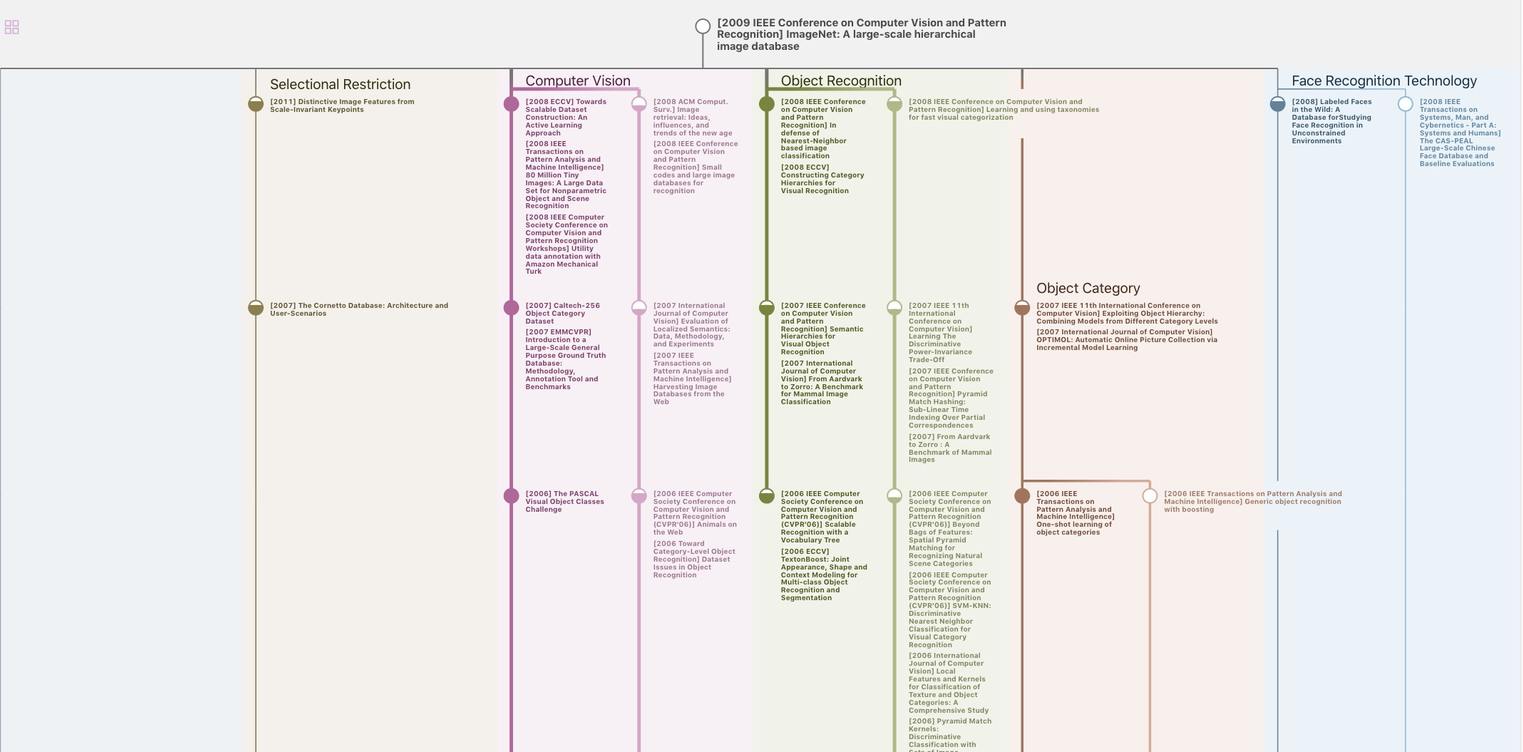
Generate MRT to find the research sequence of this paper
Chat Paper
Summary is being generated by the instructions you defined