Incorporating Syntactic Cognitive in Multi-granularity Data Augmentation for Chinese Grammatical Error Correction
NEURAL INFORMATION PROCESSING, ICONIP 2023, PT VI(2024)
摘要
Chinese grammatical error correction (CGEC) has recently attracted a lot of attention due to its real-world value. The current mainstream approaches are all data-driven, but the following flaws still exist. First, there is less high-quality training data with complexity and a variety of errors, and data-driven approaches frequently fail to significantly increase performance due to the lack of data. Second, the existing data augmentation methods for CGEC mainly focus on word-level augmentation and ignore syntactic-level information. Third, the current data augmentation methods are strongly randomized, and fewer can fit the cognition pattern of students on syntactic errors. In this paper, we propose a novel multi-granularity data augmentation method for CGEC, and construct a syntactic error knowledge base for error type Missing and Redundant Components, and syntactic conversion rules for error type Improper Word Order based on a finely labeled syntactic structure tree-bank. Additionally, we compile a knowledge base of character and word errors from actual student essays. Then, a data augmentation algorithm incorporating character, word, and syntactic noise is designed to build the training set. Extensive experiments show that the F0.5 in the test set is 36.77%, which is a 6.2% improvement compared to the best model in the NLPCC Shared Task, proving the validity of our method.
更多查看译文
关键词
Grammatical error correction,Data augmentation,Multi-granularity knowledge base,Sentence structure grammar
AI 理解论文
溯源树
样例
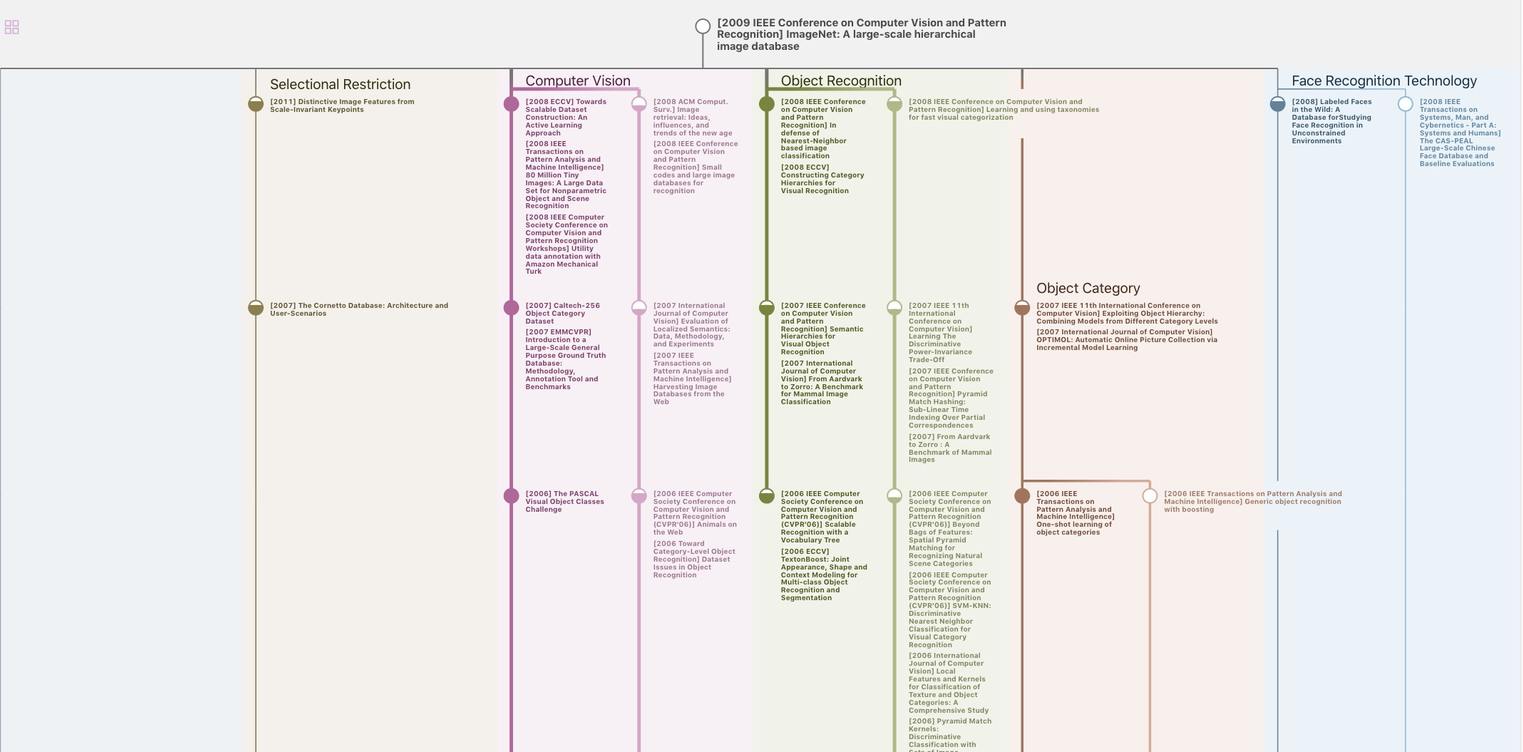
生成溯源树,研究论文发展脉络
Chat Paper
正在生成论文摘要