Exploring Non-isometric Alignment Inference for Representation Learning of Irregular Sequences
NEURAL INFORMATION PROCESSING, ICONIP 2023, PT VI(2024)
摘要
The development of Internet of Things (IoT) technology has led to increasingly diverse and complex data collection methods. This unstable sampling environment has resulted in the generation of a large number of irregular monitoring data streams, posing significant challenges for related data analysis tasks. We have observed that irregular sequence sampling densities are uneven, containing randomly occurring dense and sparse intervals. This data imbalance tendency often leads to overfitting in the dense regions and underfitting in the sparse regions, ultimately impeding the representation performance of models. Conversely, the irregularity at the data level has limited impact on the deep semantics of sequences. Based on this observation, we propose a novel Non-isometric Alignment Inference Architecture (NAIA), which utilizes a multi-level semantic continuous representation structure based on inter-interval segmentation to learn representations of irregular sequences. This architecture efficiently extracts the latent features of irregular sequences. We evaluate the performance of NAIA on multiple datasets for downstream tasks and compare it with recent benchmark methods, demonstrating NAIA's state-of-the-art performance results.
更多查看译文
关键词
Representation learning,Non-isometric Alignment Inference,Irregular sequences,Continuous latent representation
AI 理解论文
溯源树
样例
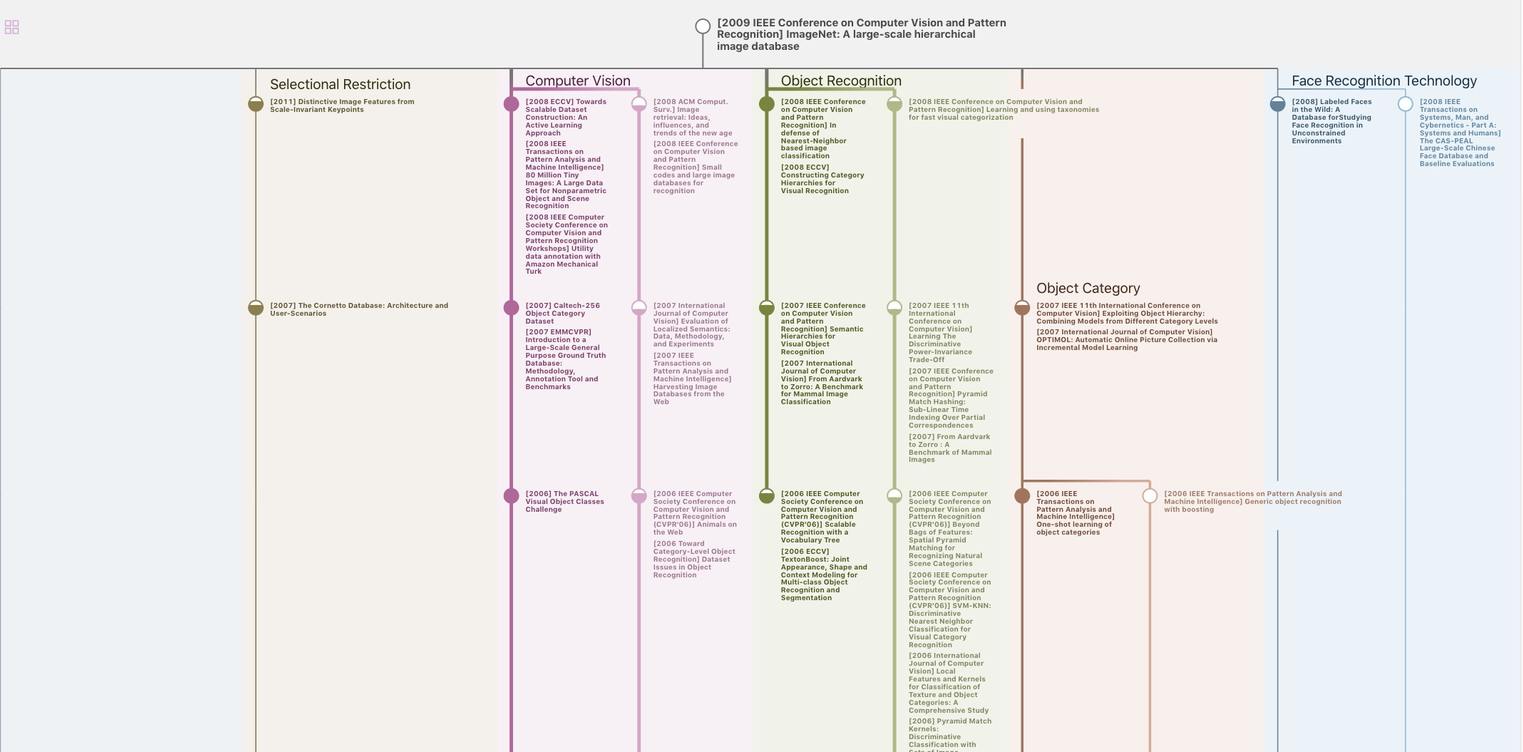
生成溯源树,研究论文发展脉络
Chat Paper
正在生成论文摘要