Extending DenseHMM with Continuous Emission
NEURAL INFORMATION PROCESSING, ICONIP 2023, PT VI(2024)
摘要
Traditional Hidden Markov Models (HMM) allow us to discover the latent structure of the observed data (both discrete and continuous). Recently proposed DenseHMM provides hidden states embedding and uses the co-occurrence-based learning schema. However, it is limited to discrete emissions, which does not meet many real-world problems. We address this shortcoming by discretizing observations and using a region-based co-occurrence matrix in the training procedure. It allows embedding hidden states for continuous emission problems and reducing the training time for large sequences. An application of the proposed approach concerns recommender systems, where we try to explain how the current interest of a given user in a given group of products (current state of the user) influences the saturation of the list of recommended products with the group of products. Computational experiments confirmed that the proposed approach outperformed regular HMMs in several benchmark problems. Although the emissions are estimated roughly, we can accurately infer the states.
更多查看译文
关键词
HMM,embedding,co-occurrence,emission discretization
AI 理解论文
溯源树
样例
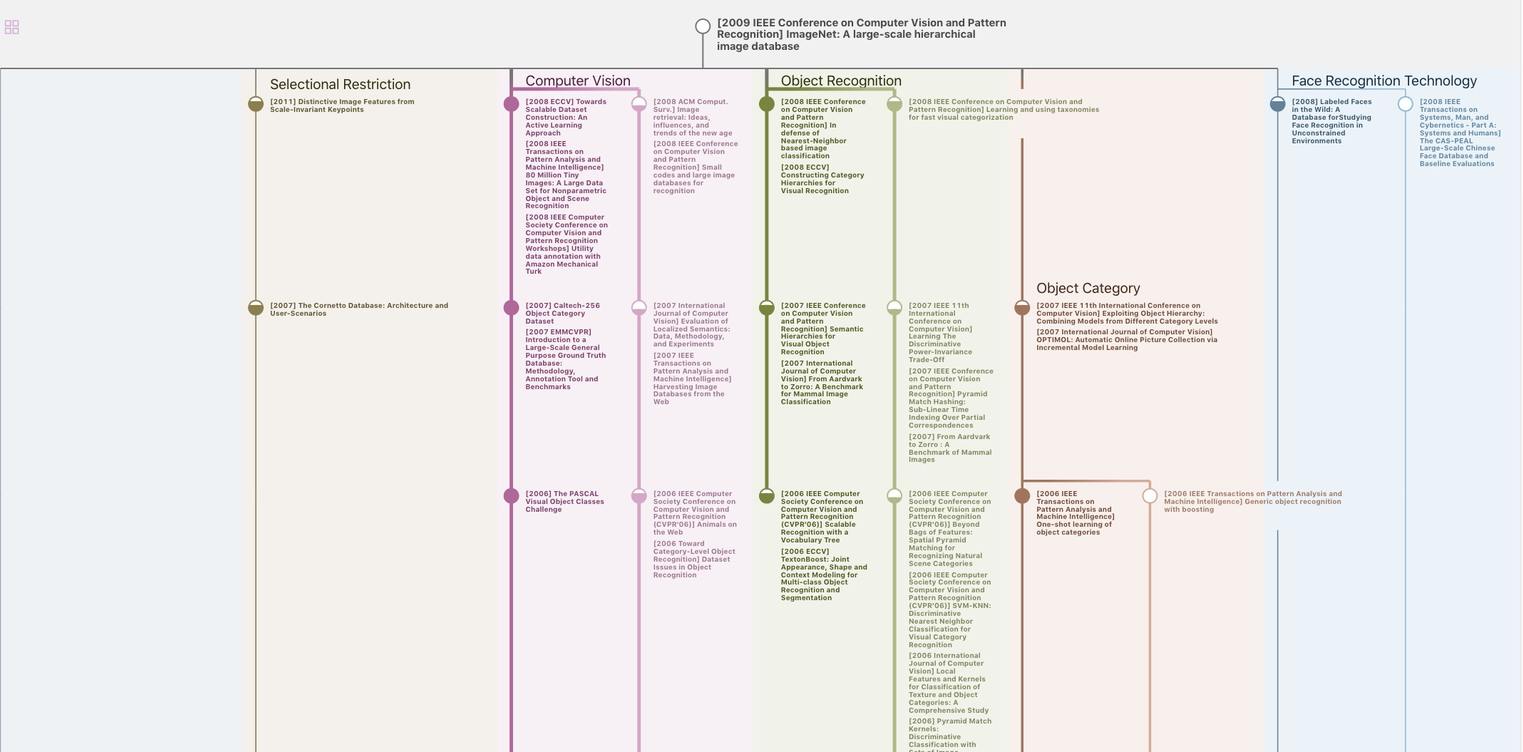
生成溯源树,研究论文发展脉络
Chat Paper
正在生成论文摘要