RPUC: Semi-supervised 3D Biomedical Image Segmentation Through Rectified Pyramid Unsupervised Consistency
NEURAL INFORMATION PROCESSING, ICONIP 2023, PT III(2024)
摘要
Deep learning models have demonstrated remarkable performance in various biomedical image segmentation tasks. However, their reliance on a large amount of labeled data for training poses challenges as acquiring well-annotated data is expensive and time-consuming. To address this issue, semi-supervised learning (SSL) has emerged as a potential solution to leverage abundant unlabeled data. In this paper, we propose a simple yet effective consistency regularization scheme called Rectified Pyramid Unsupervised Consistency (RPUC) for semi-supervised 3D biomedical image segmentation. Our RPUC adopts a pyramid-like structure by incorporating three segmentation networks. To fully exploit the available unlabeled data, we introduce a novel pyramid unsupervised consistency (PUC) loss, which enforces consistency among the outputs of the three segmentation models and facilitates the transfer of cyclic knowledge. Additionally, we perturb the inputs of the three networks with varying ratios of Gaussian noise to enhance the consistency of unlabeled data outputs. Furthermore, three pseudo labels are generated from the outputs of the three segmentation networks, providing additional supervision during training. Experimental results demonstrate that our proposed RPUC achieves state-of-the-art performance in semi-supervised segmentation on two publicly available 3D biomedical image datasets.
更多查看译文
关键词
Semi-supervised learning,Rectified pyramid consistency
AI 理解论文
溯源树
样例
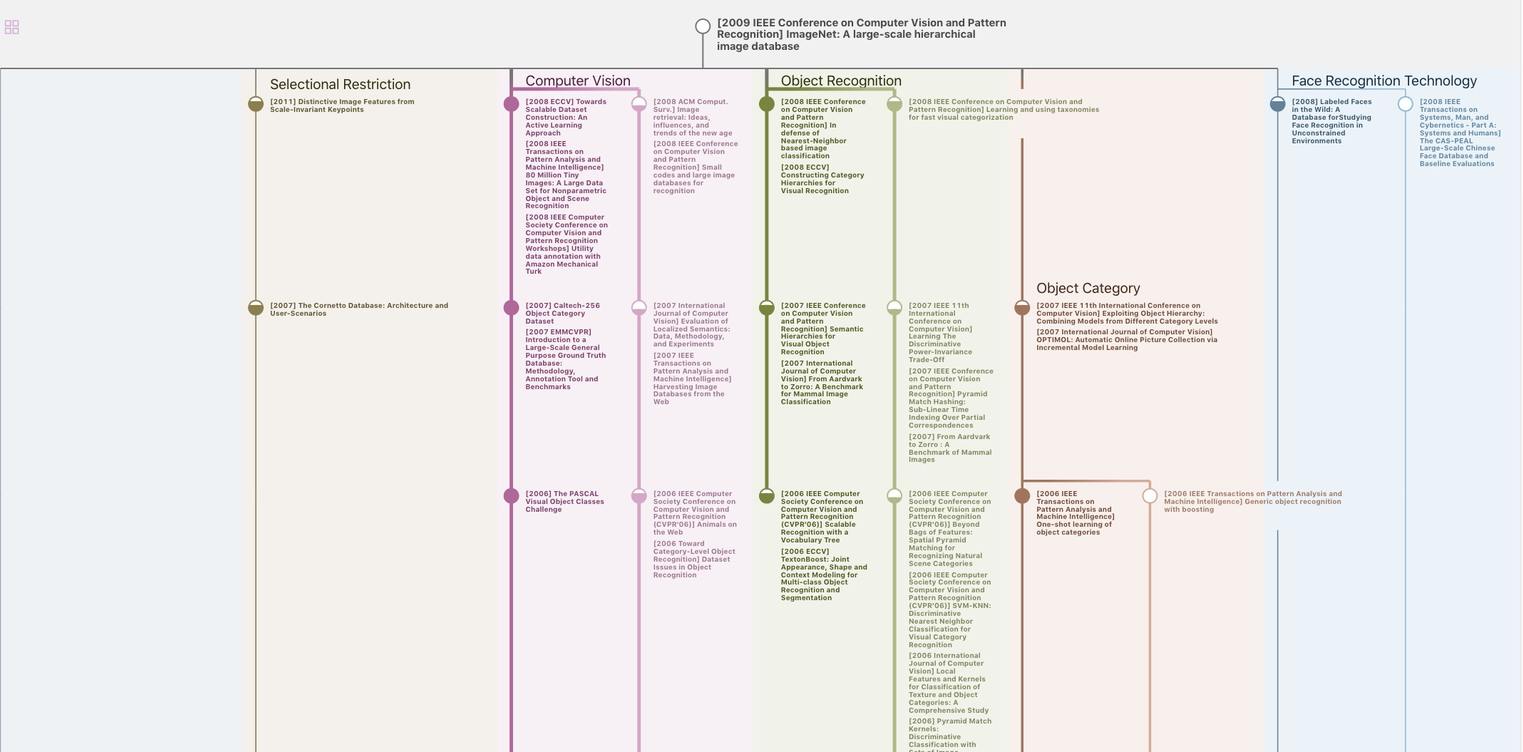
生成溯源树,研究论文发展脉络
Chat Paper
正在生成论文摘要