Using a Deep Neural Network with Small Datasets to Predict the Initial Production of Tight Oil Horizontal Wells
Electronics(2023)
摘要
Due to its abundant reserves, tight oil has emerged as a significant substitute for conventional petroleum resources. It has become one of the focal points of exploration and research, and a new hot spot in global unconventional oil and gas exploration and development. This has led to a significant increase in the demand for forecasting the production capacity of tight oil horizontal wells. The deep neural network (DNN), as a mature model, has demonstrated significant advantages in many fields. However, due to the confidentiality and uniqueness of oilfield data, acquiring large datasets has become a challenge. Traditional methods using small datasets for training DNN models result in low accuracy and overfitting issues, which hinders the development of neural networks in the petroleum industry. This study aims to predict the initial production capacity of tight oil horizontal wells by using a small dataset of 650 data points through a DNN model. The research results indicate that pre-trained and fine-tuned DNNs outperform shallow neural networks, supporting vector machines, and DNN trained with traditional methods in terms of better generalization performance. Their accuracy reached 91.3%, demonstrating that it is reasonable to use a small dataset with pre-trained and fine-tuned DNN models.
更多查看译文
关键词
small datasets,DNN,tight oil prediction,initial production capacity,horizontal wells
AI 理解论文
溯源树
样例
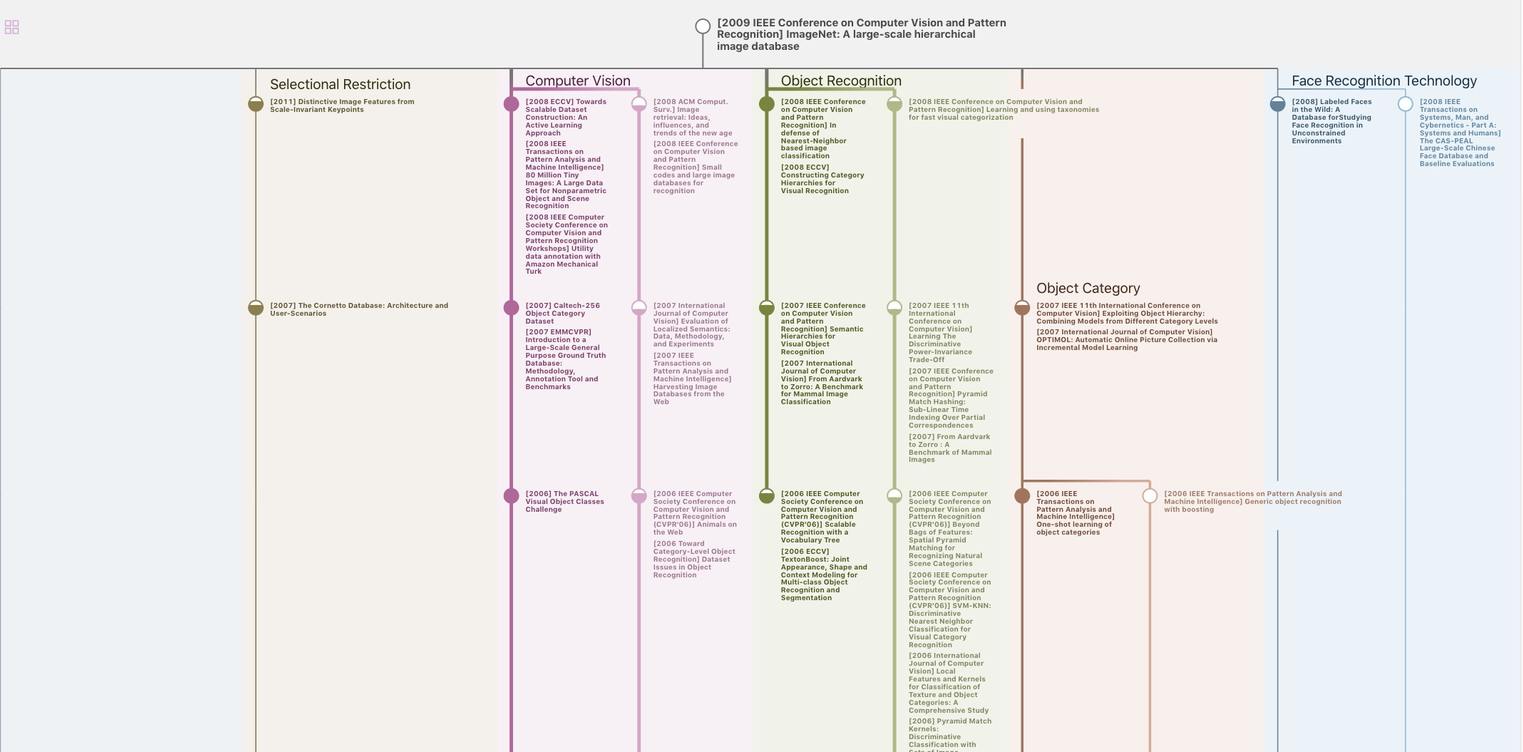
生成溯源树,研究论文发展脉络
Chat Paper
正在生成论文摘要