Degree-Aware Graph Neural Network Quantization
ENTROPY(2023)
摘要
In this paper, we investigate the problem of graph neural network quantization. Despite the great success on convolutional neural networks, directly applying current network quantization approaches to graph neural networks faces two challenges. First, the fixed-scale parameter in the current methods cannot flexibly fit diverse tasks and network architectures. Second, the variations of node degree in a graph leads to uneven responses, limiting the accuracy of the quantizer. To address these two challenges, we introduce learnable scale parameters that can be optimized jointly with the graph networks. In addition, we propose degree-aware normalization to process nodes with different degrees. Experiments on different tasks, baselines, and datasets demonstrate the superiority of our method against previous state-of-the-art ones.
更多查看译文
关键词
network quantization,graph neural network,graph data
AI 理解论文
溯源树
样例
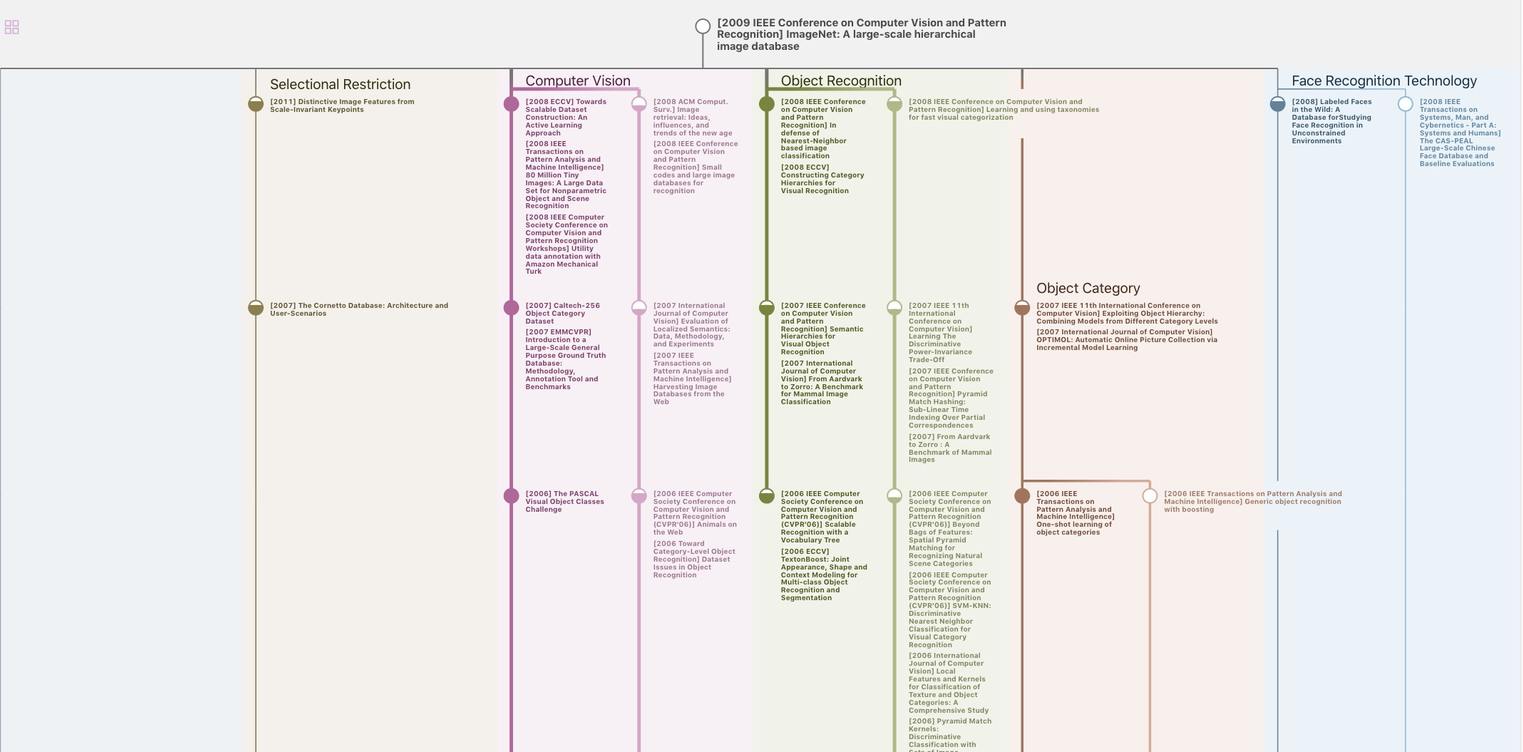
生成溯源树,研究论文发展脉络
Chat Paper
正在生成论文摘要