An Algorithm for Crack Detection, Segmentation, and Fractal Dimension Estimation in Low-Light Environments by Fusing FFT and Convolutional Neural Network
FRACTAL AND FRACTIONAL(2023)
摘要
The segmentation of crack detection and severity assessment in low-light environments presents a formidable challenge. To address this, we propose a novel dual encoder structure, denoted as DSD-Net, which integrates fast Fourier transform with a convolutional neural network. In this framework, we incorporate an information extraction module and an attention feature fusion module to effectively capture contextual global information and extract pertinent local features. Furthermore, we introduce a fractal dimension estimation method into the network, seamlessly integrated as an end-to-end task, augmenting the proficiency of professionals in detecting crack pathology within low-light settings. Subsequently, we curate a specialized dataset comprising instances of crack pathology in low-light conditions to facilitate the training and evaluation of the DSD-Net algorithm. Comparative experimentation attests to the commendable performance of DSD-Net in low-light environments, exhibiting superlative precision (88.5%), recall (85.3%), and F1 score (86.9%) in the detection task. Notably, DSD-Net exhibits a diminutive Model Size (35.3 MB) and elevated Frame Per Second (80.4 f/s), thereby endowing it with the potential to be seamlessly integrated into edge detection devices, thus amplifying its practical utility.
更多查看译文
关键词
crack detection,crack segmentation,deep learning,fractal dimension estimation,low-light environment
AI 理解论文
溯源树
样例
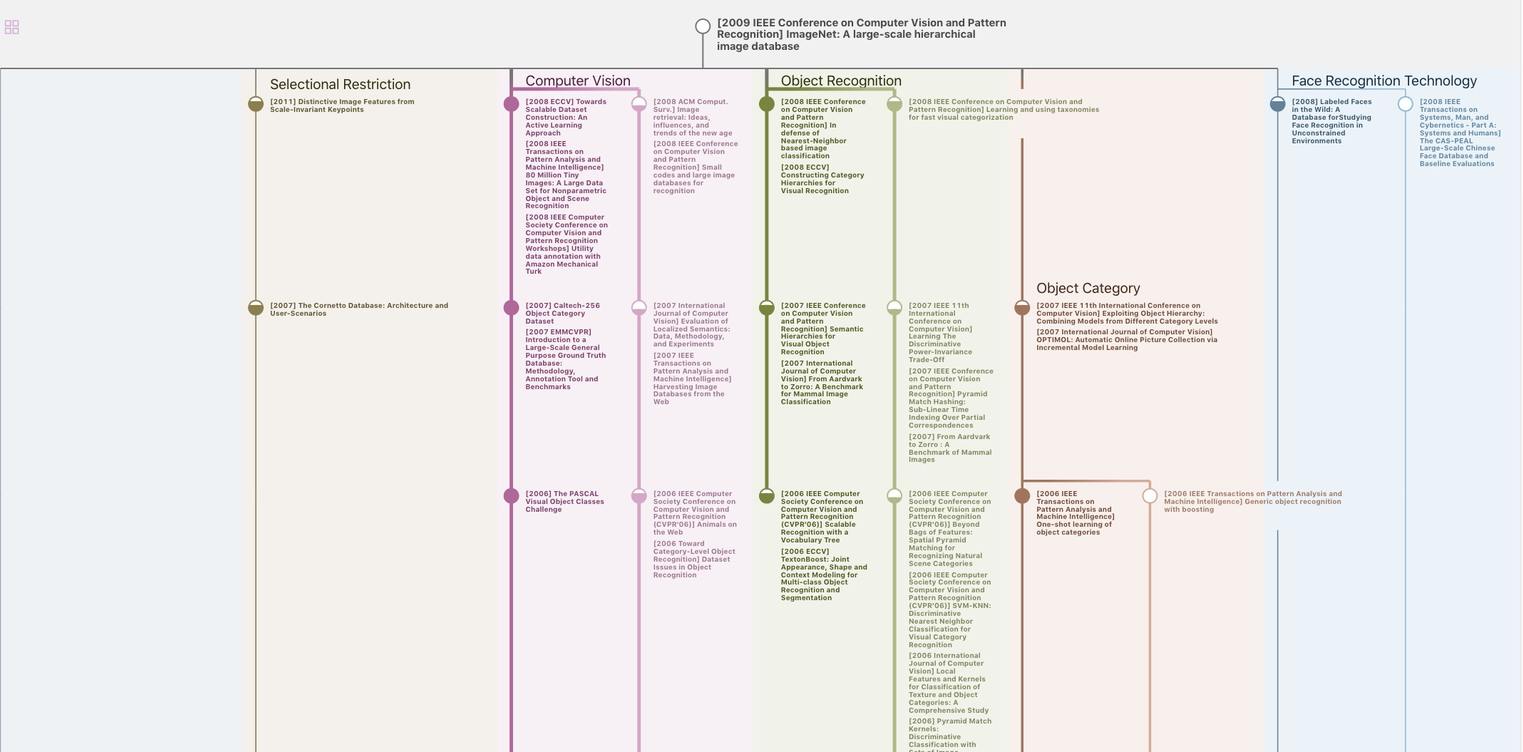
生成溯源树,研究论文发展脉络
Chat Paper
正在生成论文摘要