VEPL-Net: A Deep Learning Ensemble for Automatic Segmentation of Vegetation Encroachment in Power Line Corridors Using UAV Imagery
ISPRS INTERNATIONAL JOURNAL OF GEO-INFORMATION(2023)
摘要
Vegetation encroachment in power line corridors remains a major challenge for modern energy-dependent societies, as it can cause power outages and lead to significant financial losses. Unmanned Aerial Vehicles (UAVs) have emerged as a promising solution for monitoring infrastructure, owing to their ability to acquire high-resolution overhead images of these areas quickly and affordably. However, accurate segmentation of the vegetation encroachment in this imagery is a challenging task, due to the complexity of the scene and the high pixel imbalance between the power lines, the vegetation and the background classes. In this paper, we propose a deep learning-based approach to tackle this problem caused by the original and different geometry of the objects. Specifically, we use DeepLabV3, U-Net and a modified version of the U-Net architecture with VGG-16 weights to train two separate models. One of them segments the dominant classes, the vegetation from the background, achieving an IoU of 0.77. The other one segments power line corridors from the background, obtaining an IoU of 0.64. Finally, ensembling both models into one creates an "encroachment" zone, where power lines and vegetation are intersected. We train our models using the Vegetation Encroachment in Power Line Corridors dataset (VEPL), which includes RGB orthomosaics and multi-label masks for segmentation. Experimental results demonstrate that our approach outperforms individual networks and original prominent architectures when applied to this specific problem. This approach has the potential to significantly improve the efficiency and accuracy of vegetation encroachment monitoring using UAV, thus helping to ensure the reliability and sustainability of power supply.
更多查看译文
关键词
deep learning,vegetation encroachment monitoring,semantic segmentation,power line corridors,UAV,GeoAI
AI 理解论文
溯源树
样例
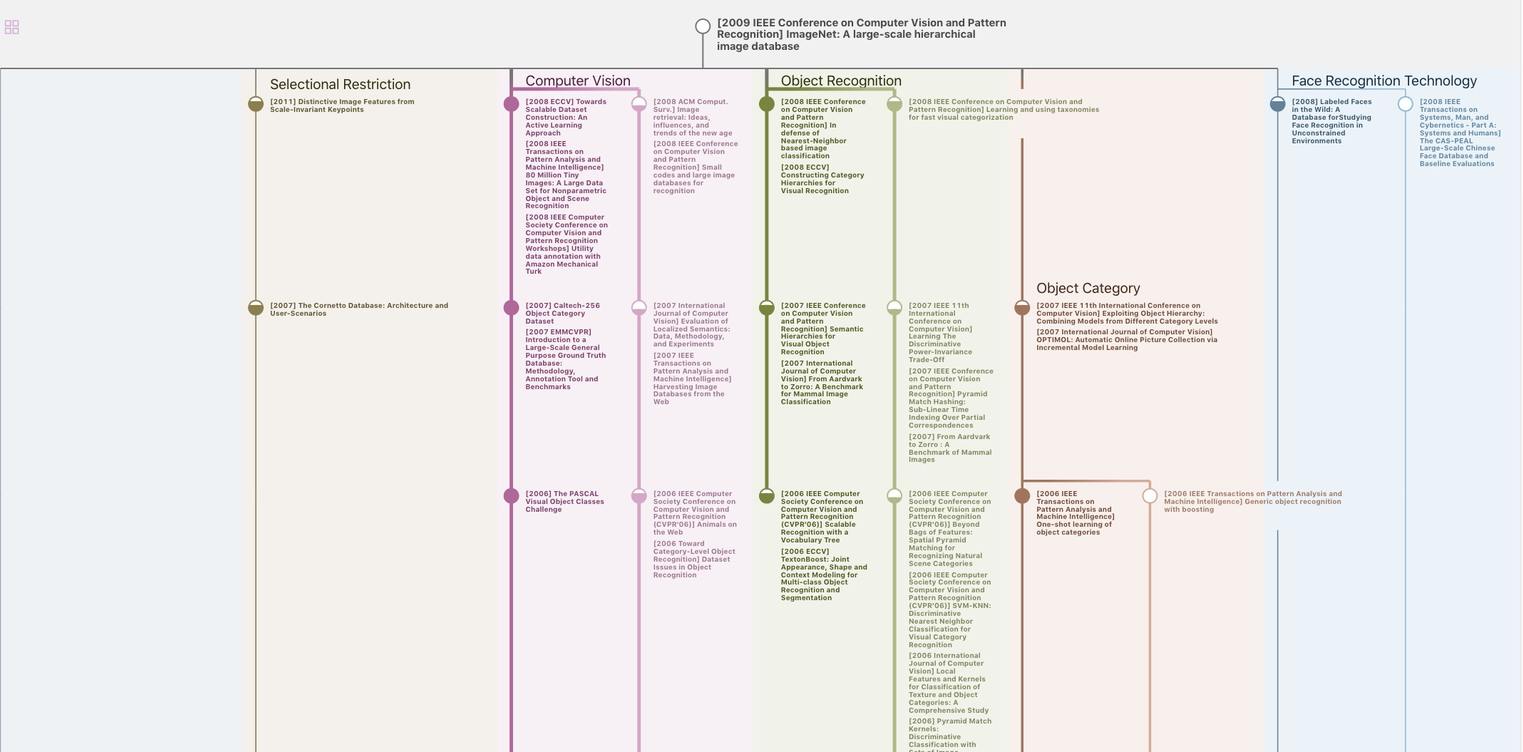
生成溯源树,研究论文发展脉络
Chat Paper
正在生成论文摘要