Investigation of Physics-Informed Neural Networks to Reconstruct a Flow Field with High Resolution
JOURNAL OF MARINE SCIENCE AND ENGINEERING(2023)
Abstract
Particle image velocimetry (PIV) is a widely used experimental technique in ocean engineering, for instance, to study the vortex fields near marine risers and the wake fields behind wind turbines or ship propellers. However, the flow fields measured using PIV in water tanks or wind tunnels always have low resolution; hence, it is difficult to accurately reveal the mechanics behind the complex phenomena sometimes observed. In this paper, physics-informed neural networks (PINNs), which introduce the Navier-Stokes equations or the continuity equation into the loss function during training to reconstruct a flow field with high resolution, are investigated. The accuracy is compared with the cubic spline interpolation method and a classic neural network in a case study of reconstructing a two-dimensional flow field around a cylinder, which is obtained through direct numerical simulation. Finally, the validated PINN method is applied to reconstruct a flow field measured using PIV and shows good performance.
MoreTranslated text
Key words
physics-informed neural network,flow field reconstruction,Navier-Stokes equations,continuity equation
AI Read Science
Must-Reading Tree
Example
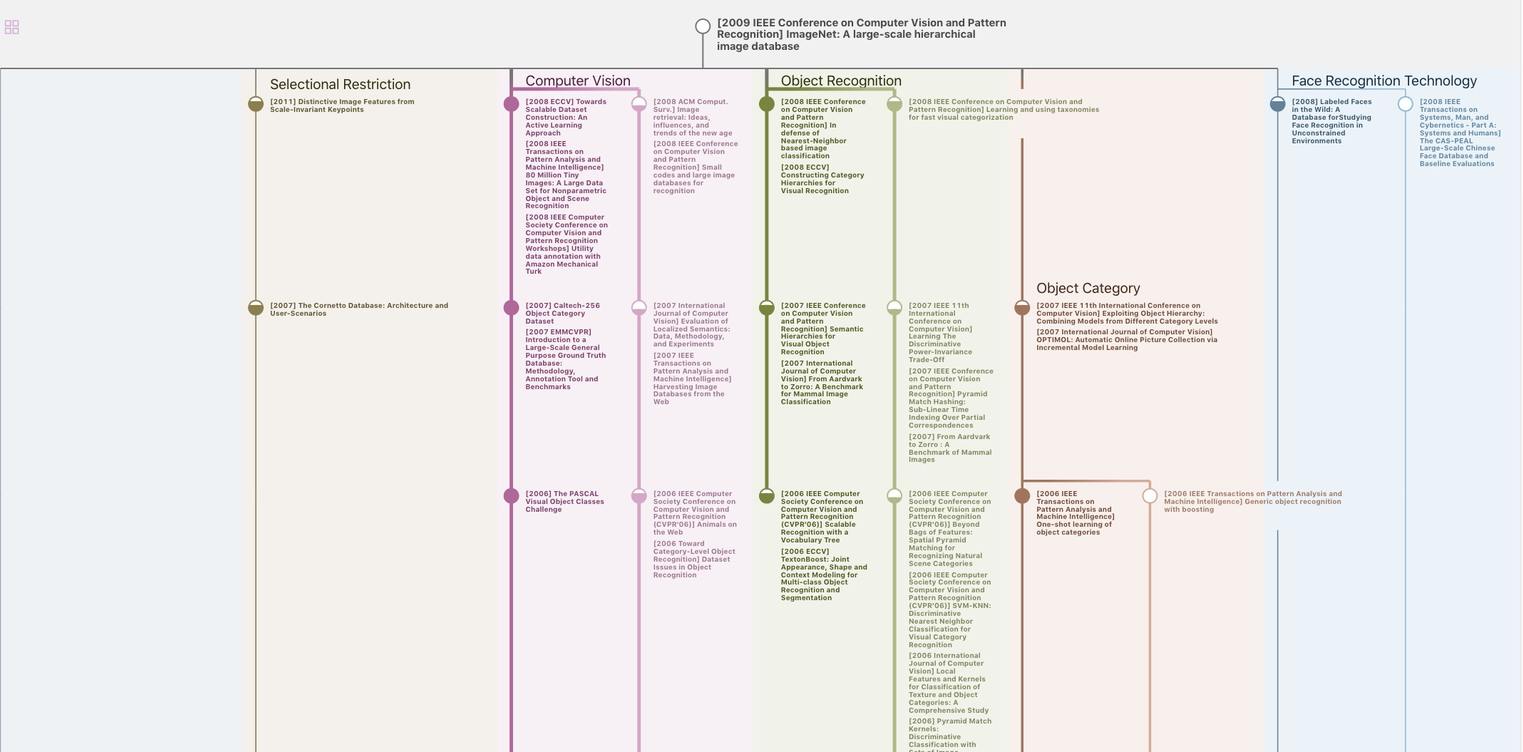
Generate MRT to find the research sequence of this paper
Chat Paper
Summary is being generated by the instructions you defined