Deep-Neural-Network-Based Receiver Design for Downlink Non-Orthogonal Multiple-Access Underwater Acoustic Communication
JOURNAL OF MARINE SCIENCE AND ENGINEERING(2023)
Abstract
The excavation of the ocean has led to the submersion of numerous autonomous vehicles and sensors. Hence, there is a growing need for multi-user underwater acoustic communication. On the other hand, due to the limited bandwidth of the underwater acoustic channel, downlink non-orthogonal multiple access (NOMA) is one of the fundamental pieces of technology for solving the problem of limited bandwidth, and it is expected to be beneficial for many modern wireless underwater acoustic applications. NOMA downlink underwater acoustic communication (UWA) is accomplished by broadcasting data symbols from a source station to several users, which uses superimposed coding with variable power levels to enable detection through successive interference cancellation (SIC) receivers. Nevertheless, comprehensive information of the channel condition and channel state information (CSI) are both essential for SIC receivers, but they can be difficult to obtain, particularly in an underwater environment. To address this critical issue, this research proposes downlink underwater acoustic communication using a deep neural network utilizing a 1D convolution neural network (CNN). Two cases are considered for the proposed system in the first case: in the first case, two users with different power levels and distances from the transmitter employ BPSK and QPSK modulations to support multi-user communication, while, in the second case, three users employ BPSK modulation. Users far from the base station receive the most power. The base station uses superimposed coding. The BELLHOP ray-tracing algorithm is utilized to generate the training dataset with user depth and range modifications. For training the model, a composite signal passes through the samples of the UWA channel and is fed to the model along with labels. The DNN receiver learns the characteristic of the UWA channel and does not depend on CSI. The testing CIR is used to evaluate the trained model. The results are compared to the traditional SIC receiver. The DNN-based DL NOMA underwater acoustic receiver outperformed the SIC receiver in terms of BER in simulation results for all the modulation orders.
MoreTranslated text
Key words
multi-user underwater acoustic communication,non-orthogonal multiple access,successive interference cancellation,deep neural network,1D convolution neural network
AI Read Science
Must-Reading Tree
Example
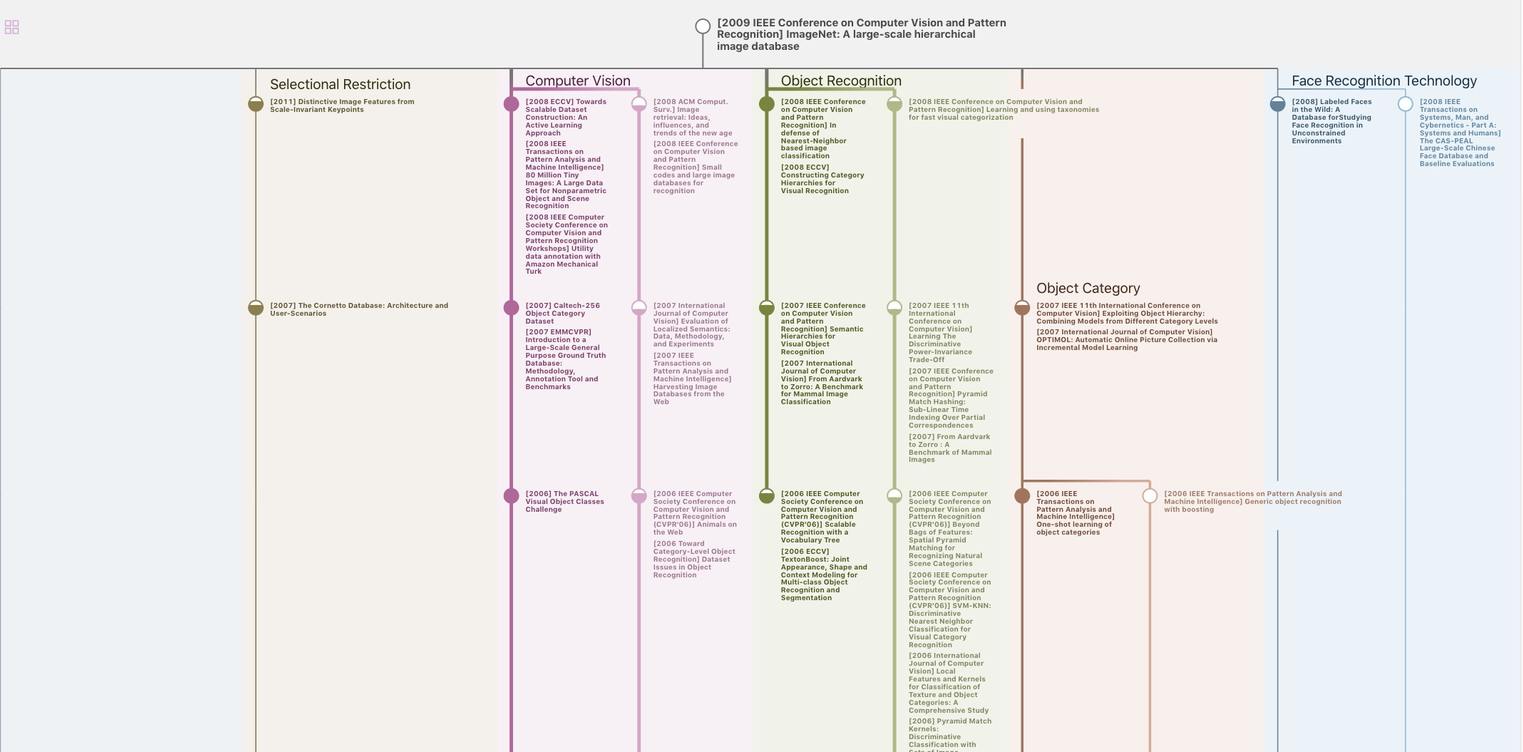
Generate MRT to find the research sequence of this paper
Chat Paper
Summary is being generated by the instructions you defined