Prediction of Spatial Distribution of Soil Organic Carbon in Helan Farmland Based on Different Prediction Models
LAND(2023)
摘要
Soil organic carbon (SOC) is widely recognized as an essential indicator of the quality of arable soils and the health of ecosystems. In addition, an accurate understanding of the spatial distribution of soil organic carbon content for precision digital agriculture is important. In this study, the spatial distribution of organic carbon in topsoil was determined using four common machine learning methods, namely the back-propagation neural network model (BPNN), random forest algorithm model (RF), geographically weighted regression model (GWR), and ordinary Kriging interpolation method (OK), with Helan County as the study area. The prediction accuracies of the four different models were compared in conjunction with multiple sources of auxiliary variables. The prediction accuracies for the four models were BPNN (MRE = 0.066, RMSE = 0.257) > RF (MRE = 0.186, RMSE = 3.320) > GWR (MRE = 0.193, RMSE = 3.595) > OK (MRE = 0.198, RMSE = 4.248). Moreover, the spatial distribution trends for the SOC content predicted with the four different models were similar: high in the western area and low in the eastern area of the study region. The BPNN model better handled the nonlinear relationship between the SOC content and multisource auxiliary variables and presented finer information for spatial differentiation. These results provide an important theoretical basis and data support to explore the spatial distribution trend for SOC content.
更多查看译文
关键词
environmental auxiliary variables,machine learning,spatial distribution,soil organic carbon
AI 理解论文
溯源树
样例
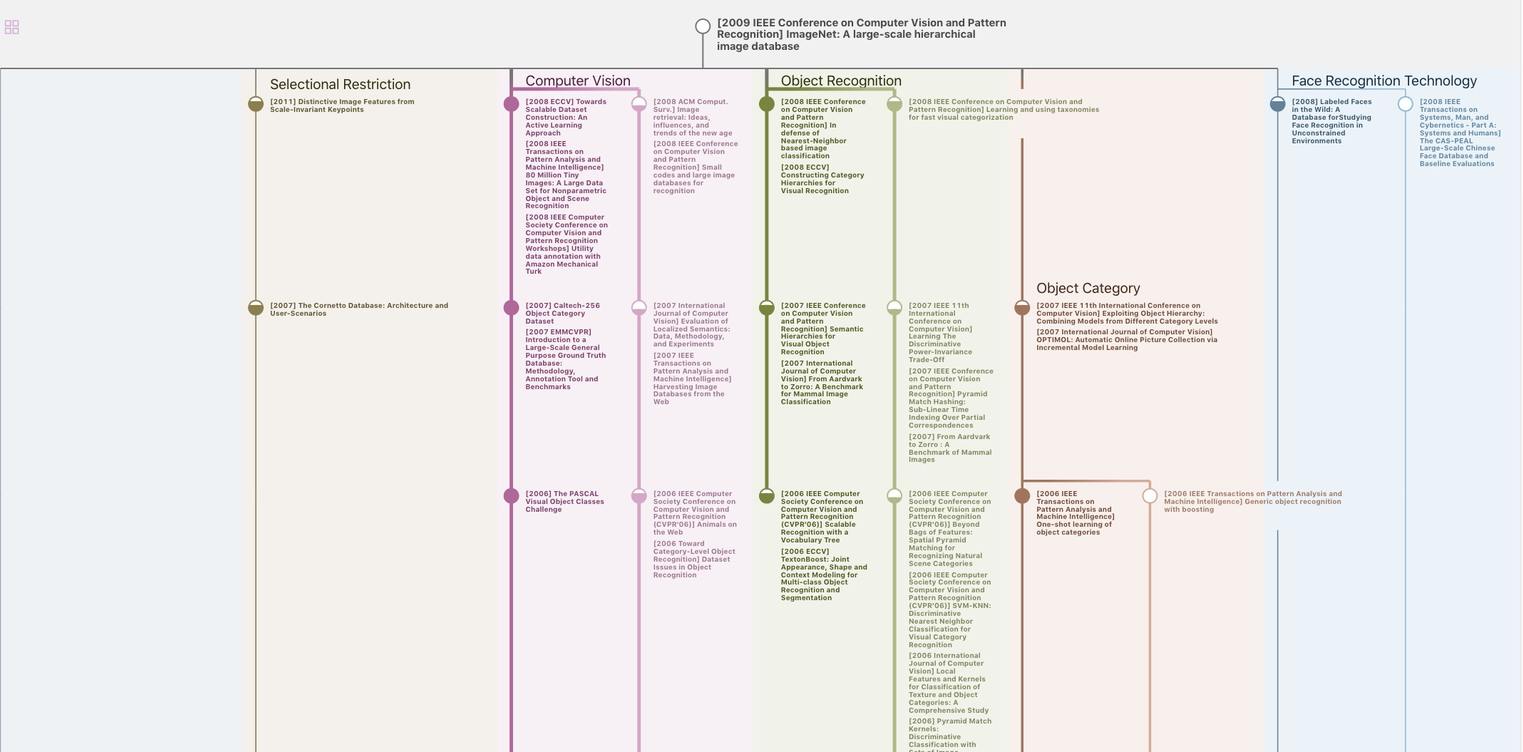
生成溯源树,研究论文发展脉络
Chat Paper
正在生成论文摘要