Tensor-Train Decomposition-Based Hybrid Beamforming for Millimeter-Wave Massive Multiple-Input Multiple-Output/Free-Space Optics in Unmanned Aerial Vehicles with Reconfigurable Intelligent Surface Networks
Photonics(2023)
摘要
Unmanned aerial vehicles (UAVs) can support low-cost, highly mobile communications while making it possible to establish dedicated terrestrial networks. To overcome the pointing error (PE) and beam misalignment of millimeter-wave large-scale multiple-input multiple-output/free-space optics (MIMO/FSO) caused by UAV jitter, a millimeter-wave massive MIMO/FSO hybrid beamforming method based on tensor train decomposition is proposed. This approach is used for reconfigurable intelligent surface (RIS) network-assisted UAV millimeter-wave massive MIMO/FSO to improve system spectral efficiency. Firstly, the high-dimensional channel of RIS-assisted millimeter-wave massive MIMO/FSO in UAV is represented as a low-dimensional channel by tensor training decomposition. Secondly, the two-way gated recursive unit attention neural network model can effectively solve the FSO PE caused by UAV jitter, and the fast fading channel and Doppler frequency shift are estimated by the Fast Cyclic Tensor Power Method (FCTPM) based on tensor training decomposition. Finally, the RIS phase shift matrix is optimized by singular value decomposition. The hybrid beamforming and RIS phase-shift matrices were estimated by using the low-complexity phase extraction alternating minimization method to solve the beam misalignment problem. Simulation results show that by using the proposed method, the spectrum utilization rate is improved by 23.6% compared with other methods.
更多查看译文
关键词
UAV communication,massive MIMO,tensor,FSO,hybrid beamforming
AI 理解论文
溯源树
样例
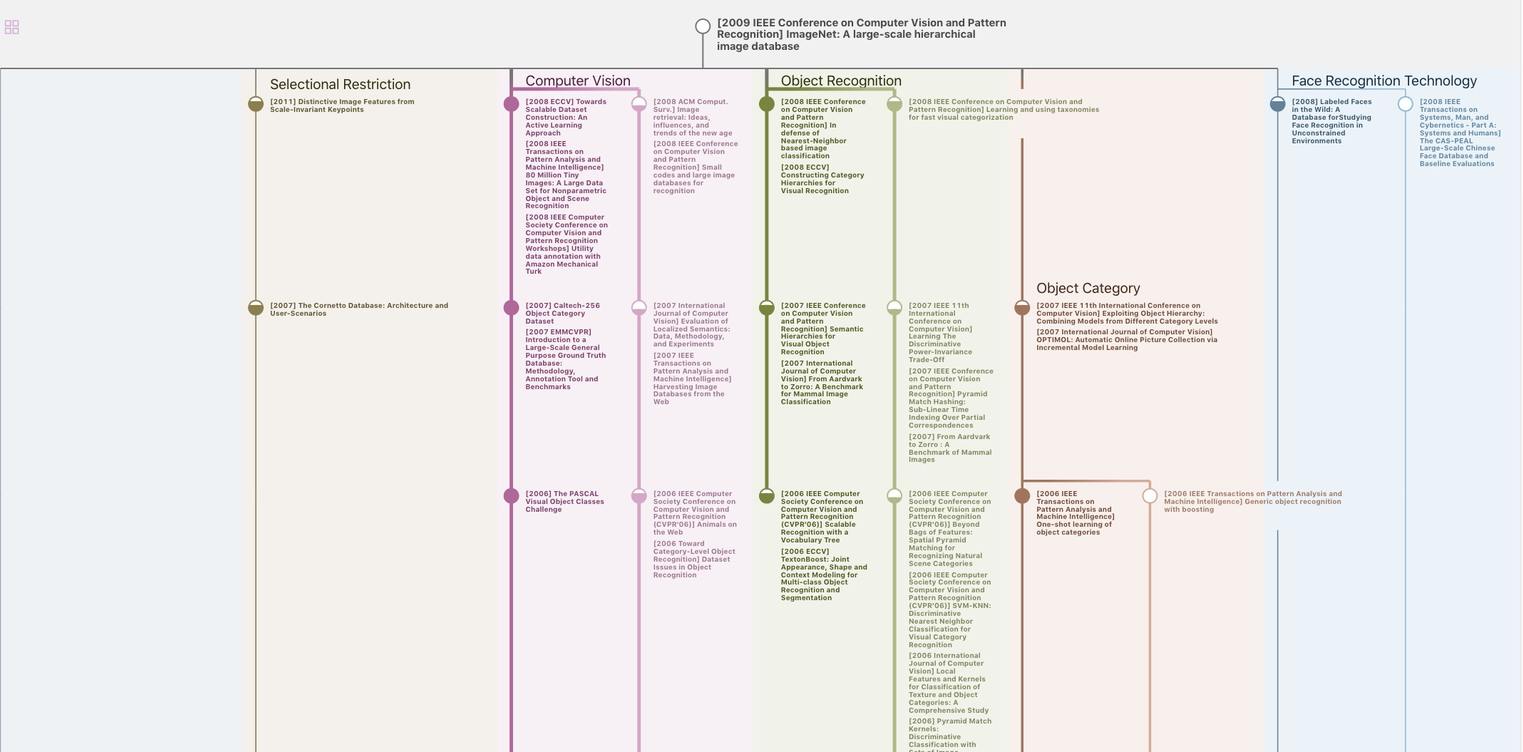
生成溯源树,研究论文发展脉络
Chat Paper
正在生成论文摘要