Driving Environment Inference from POI of Navigation Map: Fuzzy Logic and Machine Learning Approaches
SENSORS(2023)
摘要
To adapt vehicle control and plan strategies in a predictive manner, it is usually desired to know the context of a driving environment. This paper aims at efficiently inferring the following five driving environments around vehicle's vicinity: shopping zone, tourist zone, public station, motor service area, and security zone, whose existences are not necessarily mutually exclusive. To achieve that, we utilize the Point of Interest (POI) data from a navigation map as the semantic clue, and solve the inference task as a multilabel classification problem. Specifically, we first extract all relevant POI objects from a map, then transform these discrete POI objects into numerical POI features. Based on these POI features, we finally predict the occurrence of each driving environment via an inference engine. To calculate representative POI features, a statistical approach is introduced. To composite an inference engine, three inference systems are investigated: fuzzy inference system (FIS), support vector machine (SVM), and multilayer perceptron (MLP). In total, we implement 11 variants of inference engine following two inference strategies: independent and unified inference strategies, and conduct comprehensive evaluation on a manually collected dataset. The result shows that the proposed inference framework generalizes well on different inference systems, where the best overall F1 score 0.8699 is achieved by the MLP-based inference engine following the unified inference strategy, along with the fastest inference time of 0.0002 millisecond per sample. Hence, the generalization ability and efficiency of the proposed inference framework are proved.
更多查看译文
关键词
driving environment inference,point of interest (POI),multilabel classification,fuzzy inference system,support vector machine,multilayer perceptron,navigation map
AI 理解论文
溯源树
样例
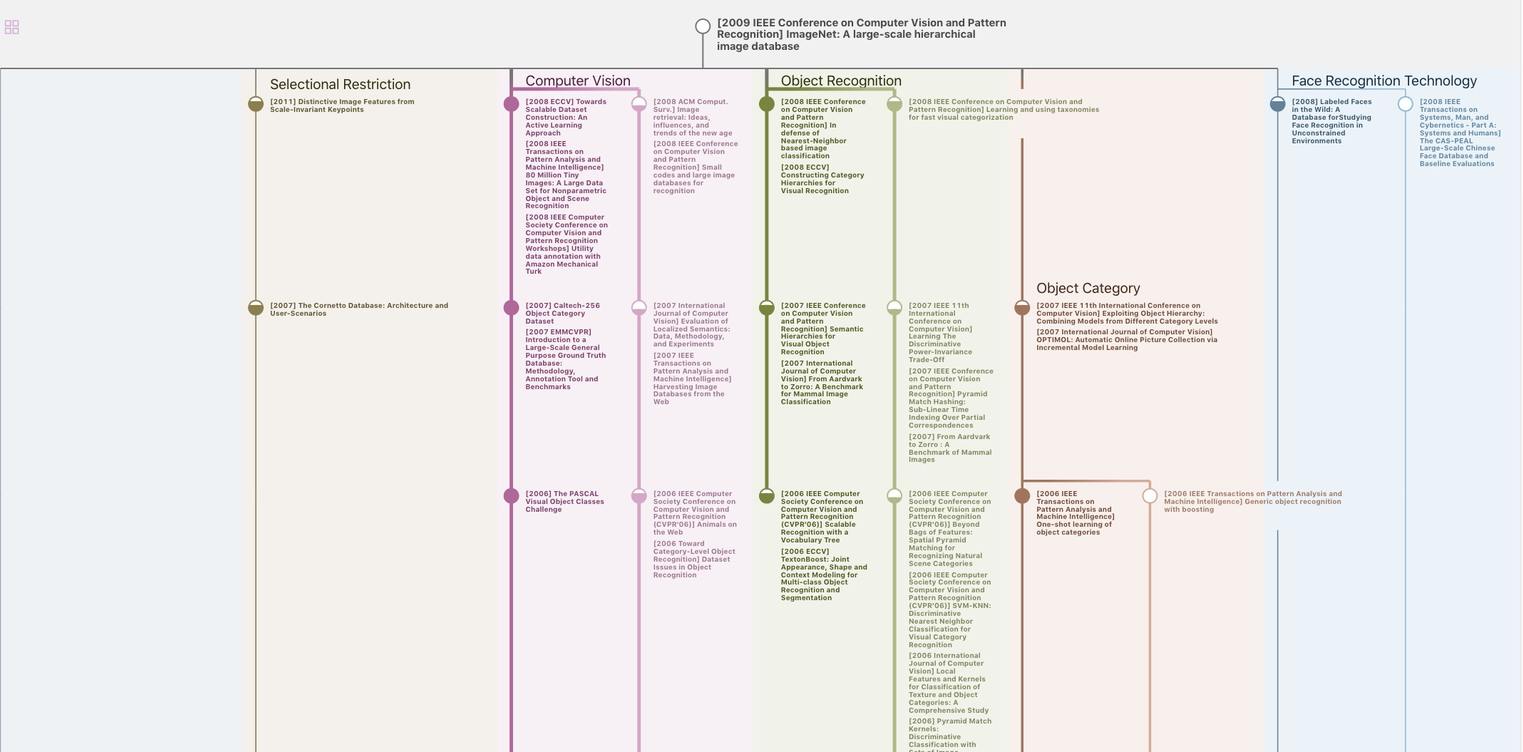
生成溯源树,研究论文发展脉络
Chat Paper
正在生成论文摘要