Chemical Composition Optimization of Biocompatible Non-Equiatomic High-Entropy Alloys Using Machine Learning and First-Principles Calculations
SYMMETRY-BASEL(2023)
摘要
Obtaining a suitable chemical composition for high-entropy alloys (HEAs) with superior mechanical properties and good biocompatibility is still a formidable challenge through conventional trial-and-error methods. Here, based on a large amount of experimental data, a machine learning technique may be used to establish the relationship between the composition and the mechanical properties of the biocompatible HEAs. Subsequently, first-principles calculations are performed to verify the accuracy of the prediction results from the machine learning model. The predicted Young's modulus and yield strength of HEAs performed very well in the previous experiments. In addition, the effect on the mechanical properties of alloying an element is investigated in the selected Ti-Zr-Hf-Nb-Ta HEA with the high crystal symmetry. Finally, the Ti8-Zr20-Hf16-Nb35-Ta21 HEA predicted by the machine learning model exhibits a good combination of biocompatibility and mechanical performance, attributed to a significant electron flow and charge recombination. This work reveals the importance of these strategies, combined with machine learning and first-principles calculations, on the development of advanced biocompatible HEAs.
更多查看译文
关键词
biocompatibility,first-principles calculation,high-entropy alloy,machine learning,mechanical properties
AI 理解论文
溯源树
样例
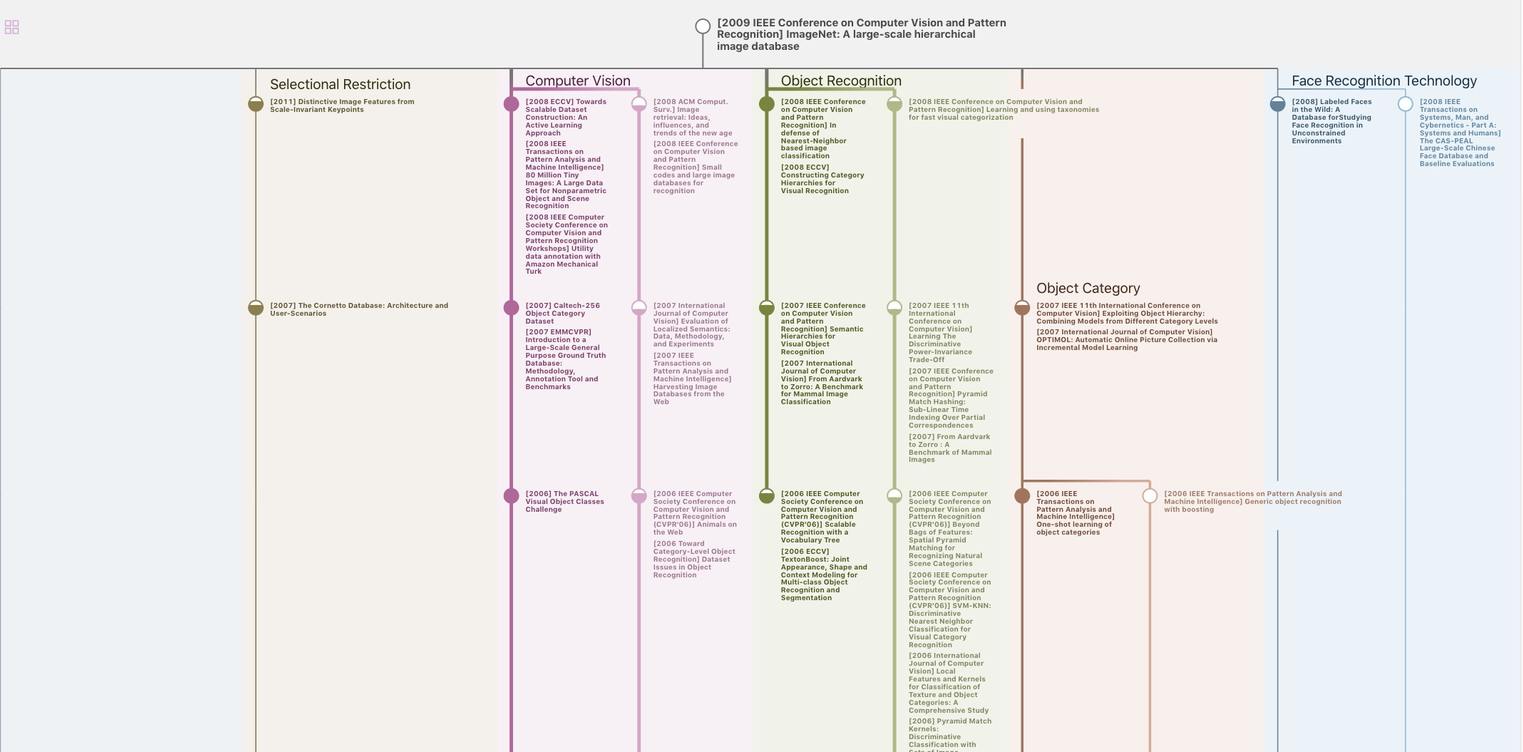
生成溯源树,研究论文发展脉络
Chat Paper
正在生成论文摘要