Decoupled knowledge distillation method based on meta-learning
HIGH-CONFIDENCE COMPUTING(2024)
摘要
With the advancement of deep learning techniques, the number of model parameters has been increasing, leading to significant memory consumption and limits in the deployment of such models in real-time applications. To reduce the number of model parameters and enhance the generalization capability of neural networks, we propose a method called Decoupled MetaDistil, which involves decoupled meta-distillation. This method utilizes meta-learning to guide the teacher model and dynamically adjusts the knowledge transfer strategy based on feedback from the student model, thereby improving the generalization ability. Furthermore, we introduce a decoupled loss method to explicitly transfer positive sample knowledge and explore the potential of negative samples knowledge. Extensive experiments demonstrate the effectiveness of our method. (c) 2023 The Author(s). Published by Elsevier B.V. on behalf of Shandong University. This is an open access article under the CC BY-NC-ND license (http://creativecommons.org/licenses/by-nc-nd/4.0/).
更多查看译文
关键词
Model compression,Knowledge distillation,Meta-learning,Decoupled loss
AI 理解论文
溯源树
样例
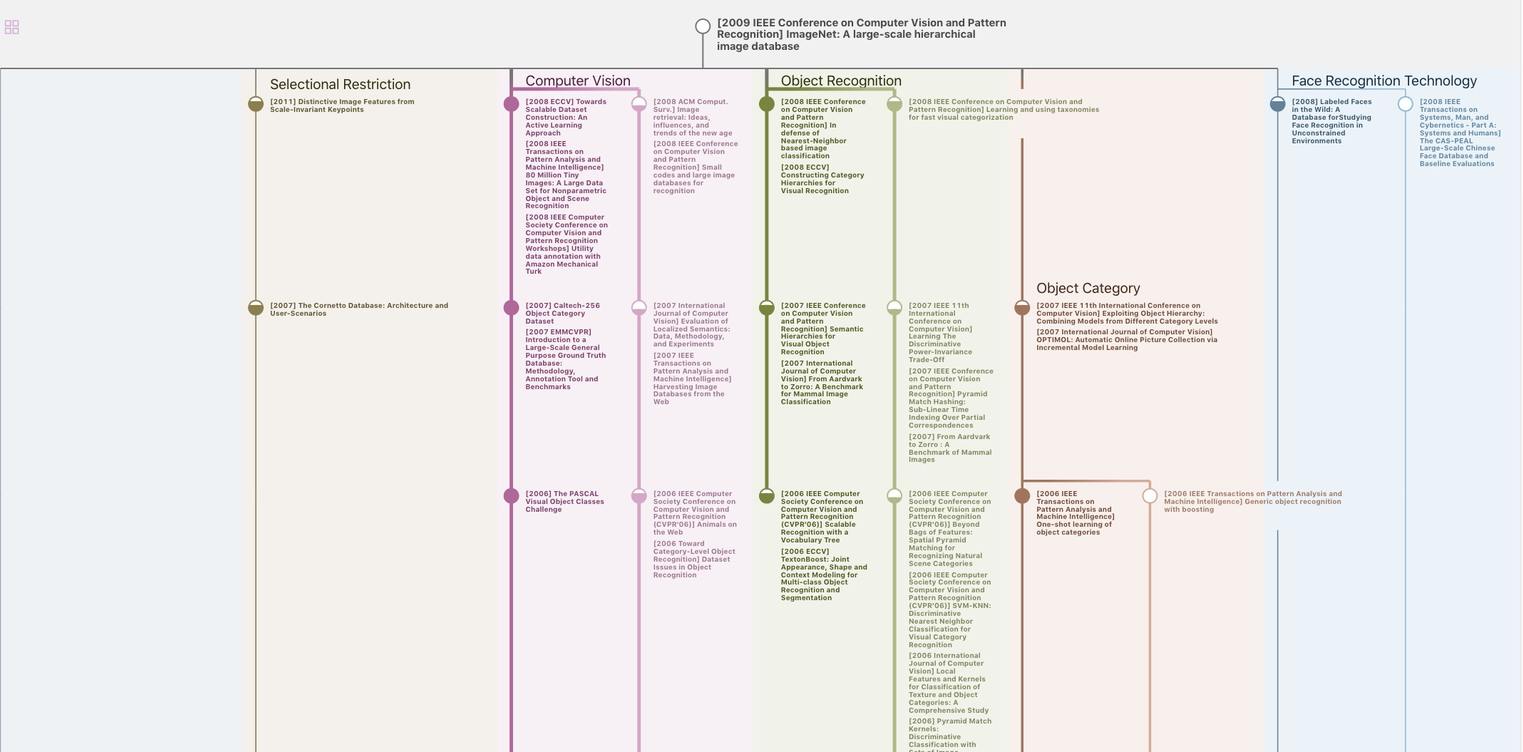
生成溯源树,研究论文发展脉络
Chat Paper
正在生成论文摘要