PEB-DDI: A Task-Specific Dual-View Substructural Learning Framework for Drug-Drug Interaction Prediction
IEEE JOURNAL OF BIOMEDICAL AND HEALTH INFORMATICS(2024)
摘要
Adverse drug-drug interactions (DDIs) pose potential risks in polypharmacy due to unknown physicochemical incompatibilities between co-administered drugs. Recent studies have utilized multi-layer graph neural network architectures to model hierarchical molecular substructures of drugs, achieving excellent DDI prediction performance. While extant substructural frameworks effectively encode interactions from atom-level features, they overlook valuable chemical bond representations within molecular graphs. More critically, given the multifaceted nature of DDI prediction tasks involving both known and novel drug combinations, previous methods lack tailored strategies to address these distinct scenarios. The resulting lack of adaptability impedes further improvements to model performance. To tackle these challenges, we propose PEB-DDI, a DDI prediction learning framework with enhanced substructure extraction. First, the information of chemical bonds is integrated and synchronously updated with the atomic nodes. Then, different dual-view strategies are selected based on whether novel drugs are present in the prediction task. Particularly, we constructed Molecular fingerprint-Molecular graph view for transductive task, and Bipartite graph-Molecular graph view for inductive task. Rigorous evaluations on benchmark datasets underscore PEB-DDI's superior performance. Notably, on DrugBank, it achieves an outstanding accuracy rate of 98.18% when predicting previously unknown interactions among approved drugs. Even when faced with novel drugs, PEB-DDI consistently exhibits outstanding generalization capabilities with an accuracy rate of 88.06%, attributing to the proper migrating of molecular basic structure learning.
更多查看译文
关键词
Drug-drug interaction,substructure learning,fingerprints,bipartite graphs,graph neural network
AI 理解论文
溯源树
样例
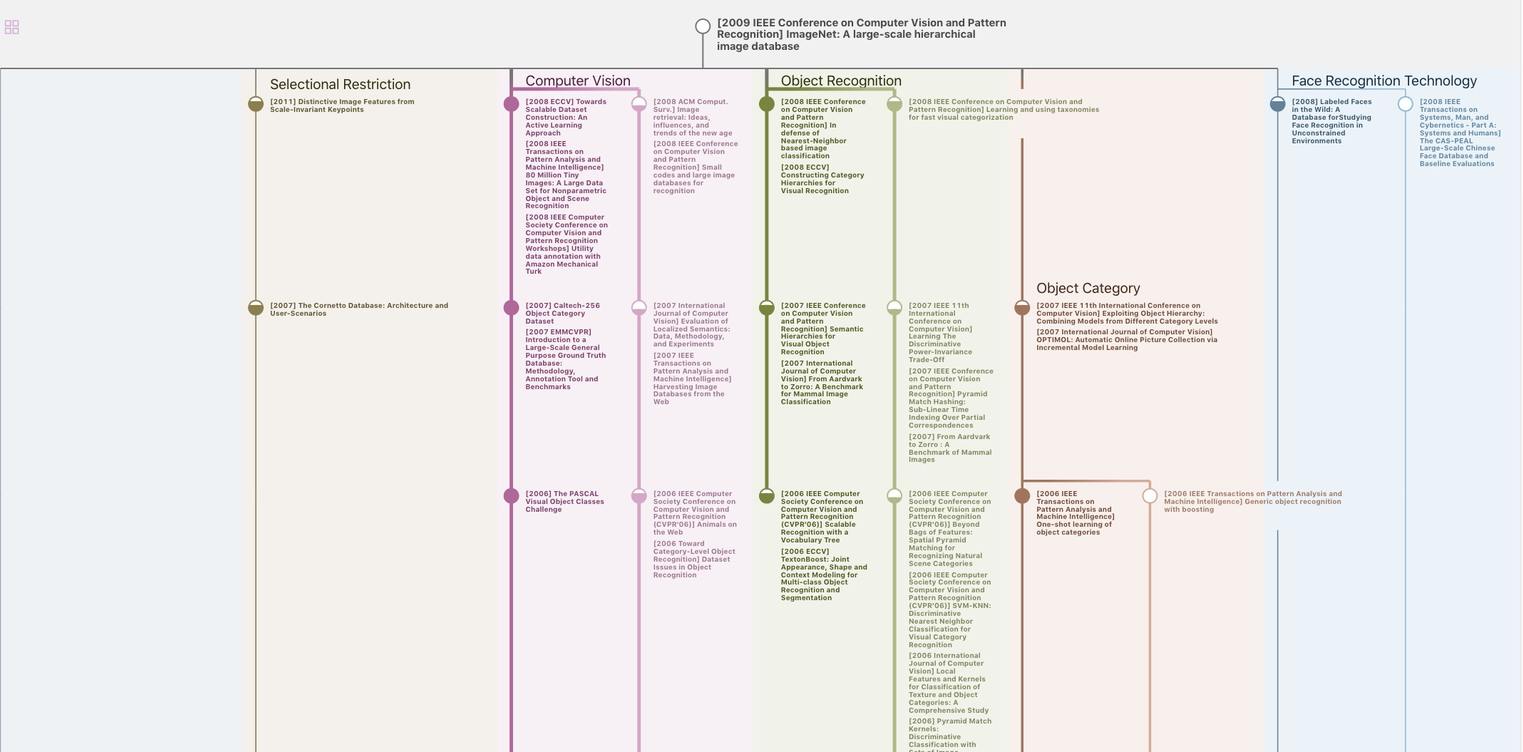
生成溯源树,研究论文发展脉络
Chat Paper
正在生成论文摘要