Automated Multi-class Crater Segmentation in Mars Orbital Images
PROCEEDINGS OF THE 6TH ACM SIGSPATIAL INTERNATIONAL WORKSHOP ON AI FOR GEOGRAPHIC KNOWLEDGE DISCOVERY, GEOAI 2023(2023)
摘要
Crater mapping and counting are critical analyses in many planetary science investigations, as the size-frequency distribution of impact craters can be used to measure the age of a planet's surface and interpret its geologic history. Crater counting is extremely tedious - counting hundreds to thousands of small features in a small region could take days to months for a trained planetary scientist. Previous work has demonstrated the feasibility of using computer vision techniques to automatically map and count craters in Mars orbital images using semantic segmentation. We present an improved approach for binary and multi-class semantic segmentation of craters in THEMIS daytime thermal infrared images using U-2-Net and U-NetFormer with template matching. Our approach is the first method to perform multi-class segmentation of craters using computer vision. Our binary segmentation approach outperforms previous approaches that used semantic segmentation and template matching. A new global high-resolution image mosaic dataset of Mars (CTX, 5 m/px) is now available, but to date, no studies have benchmarked automated crater counting methods for this improved dataset. Toward this goal, we applied the THEMIS-trained model to out-of-domain CTX datasets and evaluated results quantitatively using the DoMars16 benchmark dataset and qualitatively using global mosaic tiles. We show that the THEMIS-trained models effectively segment craters in CTX images without additional fine-tuning. The code can be found here.
更多查看译文
关键词
Multi-class crater classification,semantic segmentation,out-of-distribution evaluation,U-Net,template matching,remote sensing
AI 理解论文
溯源树
样例
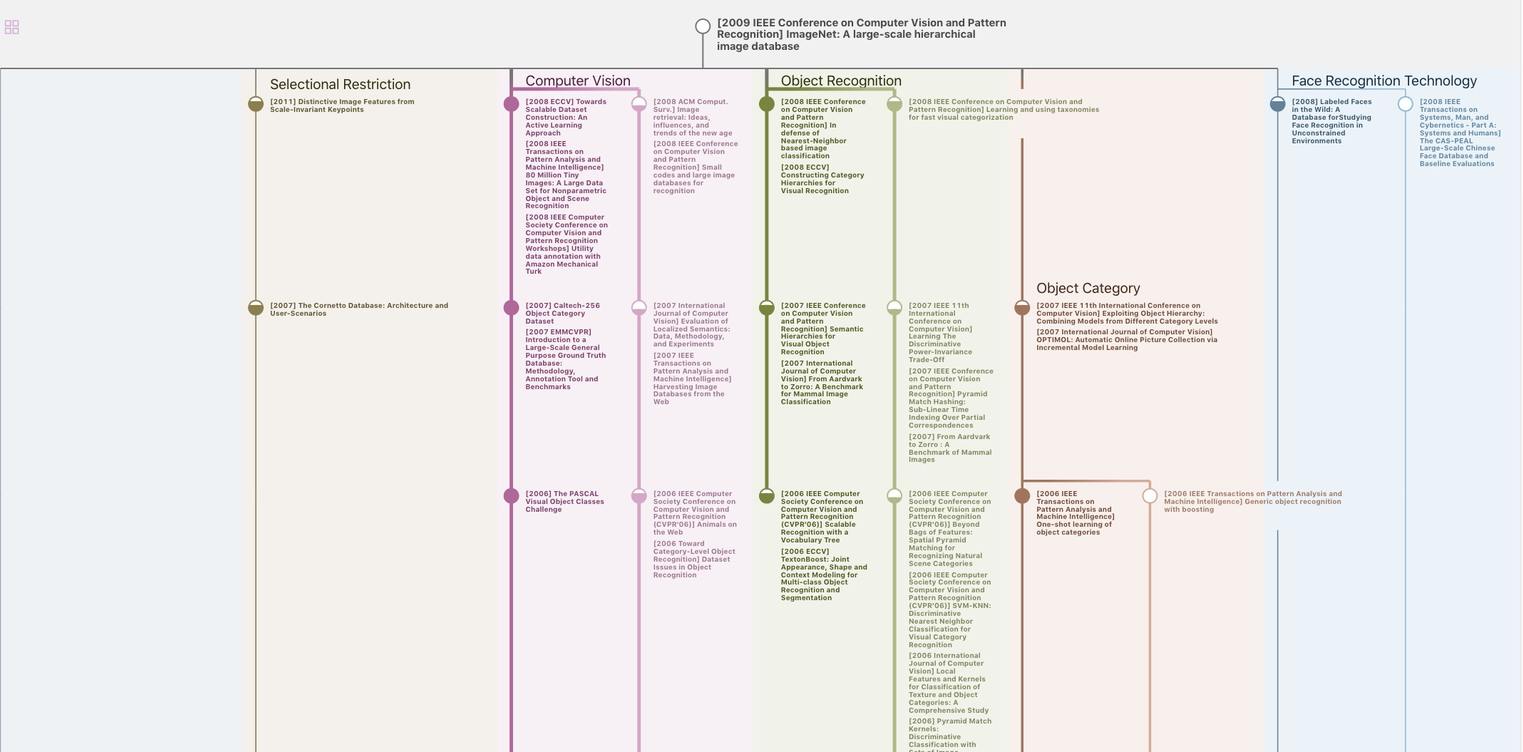
生成溯源树,研究论文发展脉络
Chat Paper
正在生成论文摘要