Decoupled and Reparameterized Compound Attention-Based Light Field Depth Estimation Network
IEEE ACCESS(2023)
摘要
A light field (LF) camera captures both spatial and angular information of the real world, and the intertwined nature of these dimensions presents a pressing challenge in effectively disentangling meaningful LF information for depth estimation. This paper introduces a feature extraction network based on LF decoupling, which ingeniously separates the LF. Furthermore, given the extensive volume of input data inherent in LF images, a novel reparameterizable Residual-Densely Branched Leaky-ReLU Block(Res-DBLB) architecture was developed to replace conventional residual structures and multibranch architectures and enhance inference efficiency. Incorporating an attention mechanism further refines the network, effectively addressing the computational intensity and time-consuming nature of LF depth estimation, thus furthering the advancement of this technology. Our model was applied to widely used datasets as well as the latest LF datasets, namely HCI and UrbanLF, showing superior performance over six other popular models across four evaluation metrics.
更多查看译文
关键词
Estimation,Feature extraction,Computer architecture,Cameras,Light fields,Spatial resolution,Data mining,Parameter estimation,Light field depth estimation,decoupling,reparameterization,attention mechanism
AI 理解论文
溯源树
样例
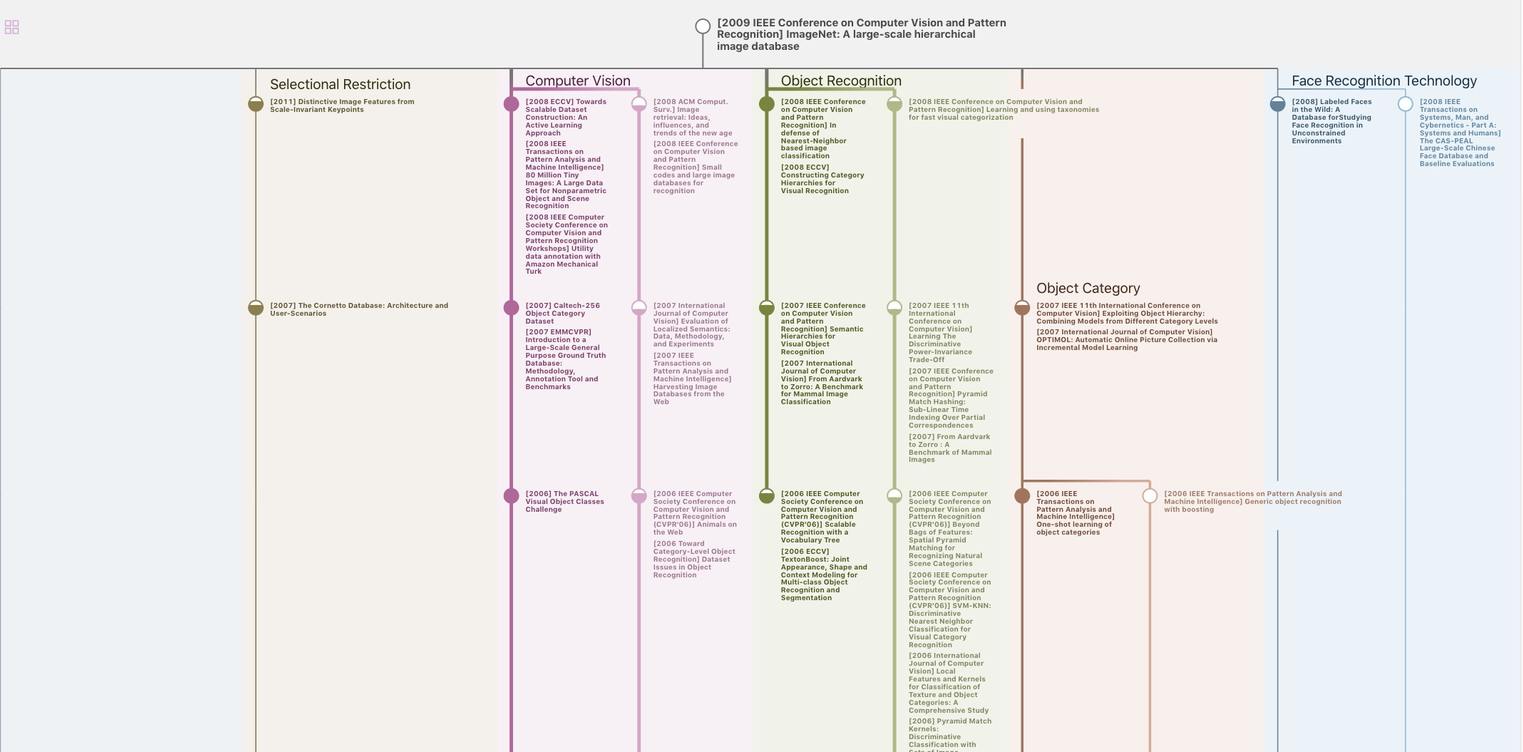
生成溯源树,研究论文发展脉络
Chat Paper
正在生成论文摘要