Convolutional Channel Attentional Facial Expression Recognition Network and Its Application in Human-Computer Interaction
IEEE ACCESS(2023)
Abstract
Currently, the use of robots has altered the way people live and their lifestyles. To realize a human-computer interaction system based on robots' comprehension of human emotions, this study chooses facial expressions as the research object and constructs a facial expression recognition model based on convolutional neural networks and channel attention. To deploy the recognition model to portable devices, a depth-separable convolution filter pruning algorithm based on principal component analysis is constructed. This model applies principal component analysis to reduce the dimensionality of similar filter matrices obtained by calculating geometric medians to prevent gradient explosion. The proposed algorithm for facial expression recognition in this study achieves a 99% recognition accuracy with an average of 80.39%, while using the least number of parameters among the compared algorithms. The verification experiment results of lightweight network show that when the model depth is 56 and the pruning rate is 40%, the correct rate of facial expression recognition of the network model based on the pruning strategy proposed in the study is 93.24%. When dimension is 0.85, the accuracy of model classification is the highest. The algorithm presented in this study exhibits excellent performance when recognizing facial expressions, demonstrating notable robustness and efficiency. The pruning strategy proposed in this study has a good model acceleration effect. It can not only reduce the memory occupied by about 41% of parameters, but also improve the classification accuracy, running time and calculation cost after pruning to a certain extent. The study applied deep separable convolution and PCA techniques to improve and reduce the dimensionality of the convolutional layer in the ResNet network structure, and improved the comparable filter matrix generated during the filter pruning process.
MoreTranslated text
Key words
Face recognition,Feature extraction,Convolution,Convolutional neural networks,Tensors,Principal component analysis,Human computer interaction,Channel estimation,Filters,Channel attention,depth-separable convolution,facial expression recognition,human-computer interaction,filter pruning,principal component analysis
AI Read Science
Must-Reading Tree
Example
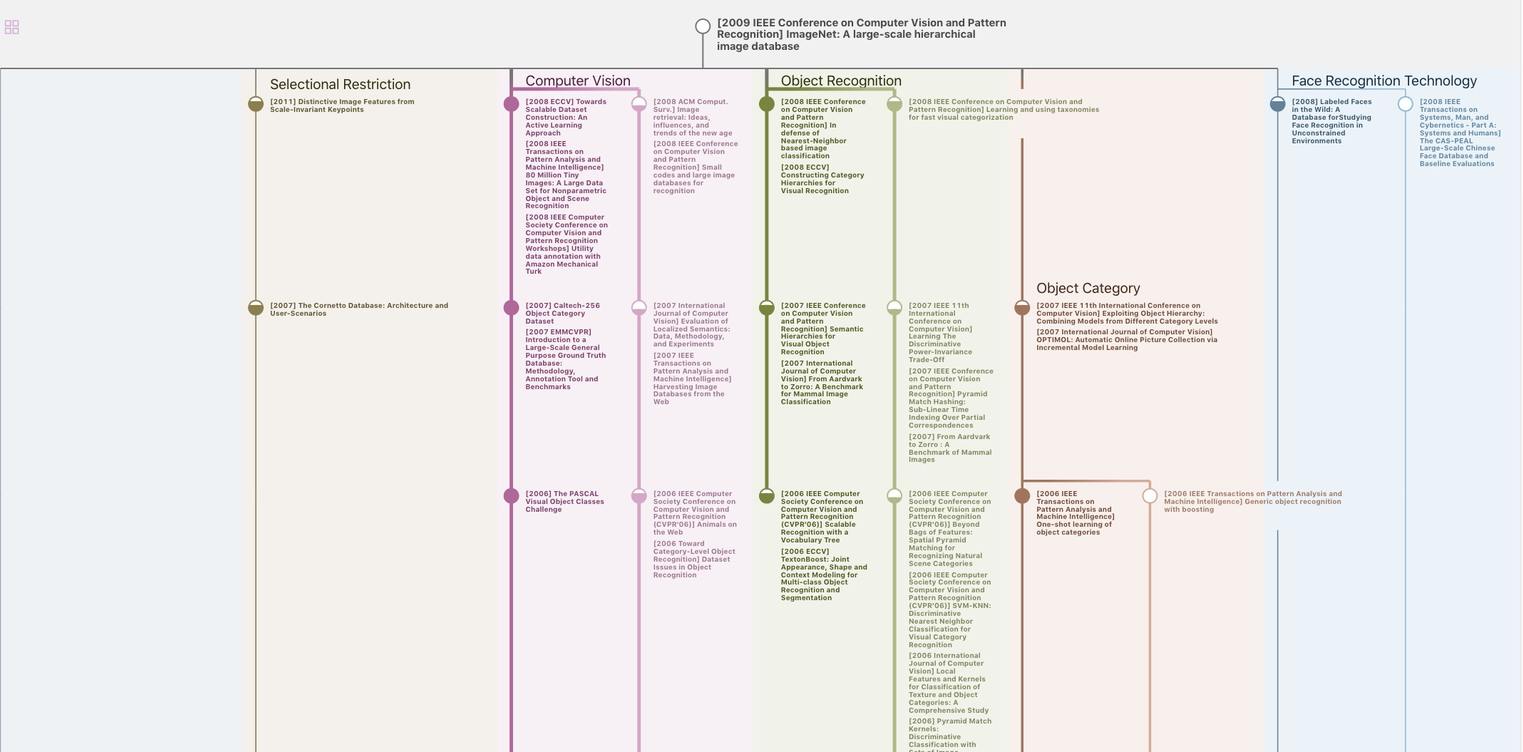
Generate MRT to find the research sequence of this paper
Chat Paper
Summary is being generated by the instructions you defined