An End-to-End Solution for Spatial Inference in Smart Buildings
PROCEEDINGS OF THE 10TH ACM INTERNATIONAL CONFERENCE ON SYSTEMS FOR ENERGY-EFFICIENT BUILDINGS, CITIES, AND TRANSPORTATION, BUILDSYS 2023(2023)
摘要
Smart building technology is an aspiring application of the Internet of Things (IoT) that utilizes various IoT sensors to serve the purposes of facility management, building automation, and improving sustainability. The collection and analysis of sensor data support informed and precise building operations and hold great potential to improve human health, productivity, comfort, as well as energy efficiency. However, these benefits are hindered by the manual labor required during sensor relationship inference, e.g., how sensors are connected and what sensors are deployed in which room. This manual process of relation inference is costly and prone to error, and thus automated solutions to this problem are called for. This paper focuses on spatial relationship inference that concerns which equipment/sensors are co-located in the same physical space. The difficulties of this problem are due to it being a combination of two complex tasks: time series representation learning and combinatorial optimization. While recent works have made notable progress on these two tasks separately, the merit of a fully automated pipeline is overlooked. In this work, we propose an end-to-end solution to spatial relationship inference that combines: 1. a metric learning module that generates sensor representations from collected time series data and 2. a reinforcement learning agent that solves the downstream combinatorial optimization problem and further improves the learned similarity metrics. Experiments on synthetic and real-world datasets demonstrate the accuracy and robustness of our approach compared to existing baselines.
更多查看译文
关键词
Combinatorial optimization,similarity learning in time series,reinforcement learning,smart building management
AI 理解论文
溯源树
样例
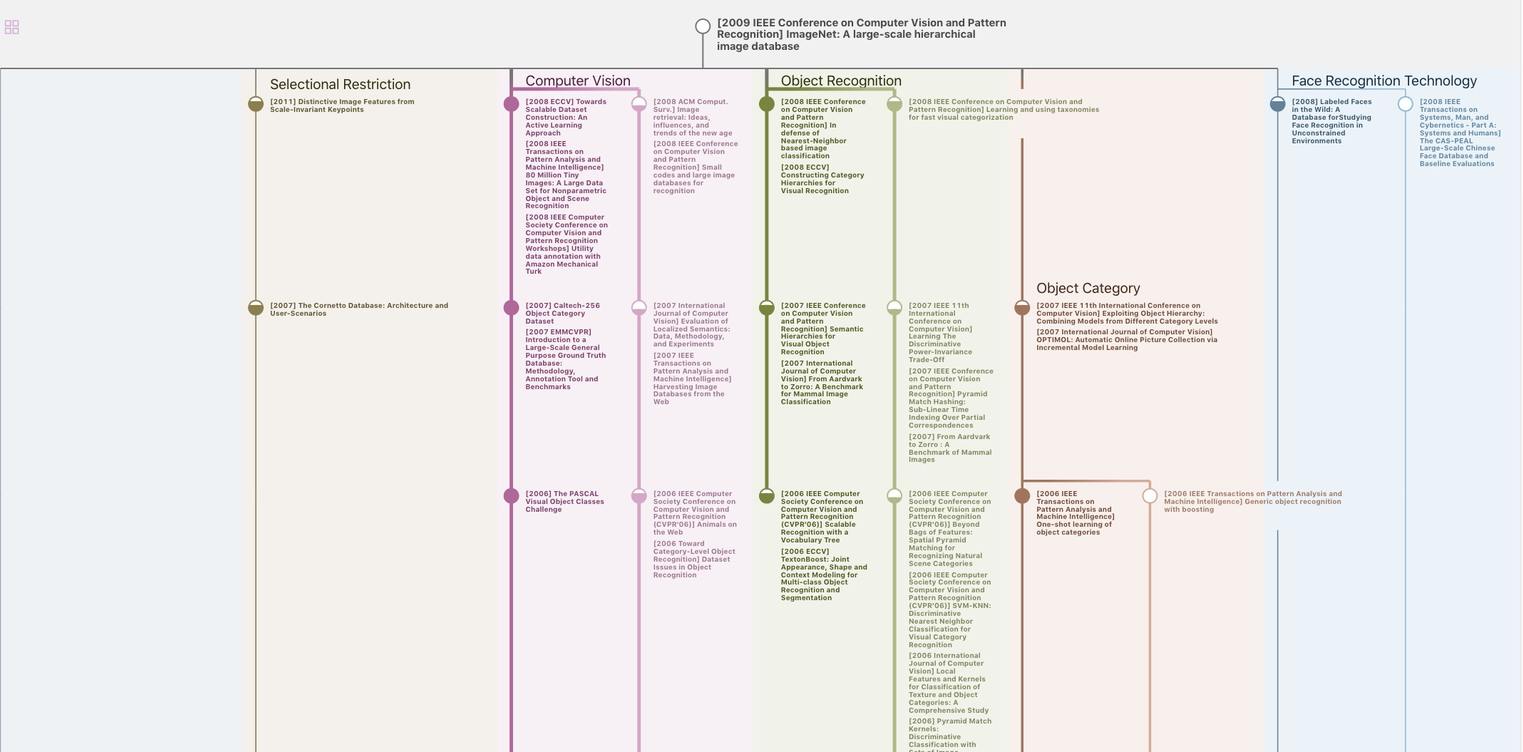
生成溯源树,研究论文发展脉络
Chat Paper
正在生成论文摘要