A Novel Graph Neural Network for Zone-Level Urban-Scale Building Energy Use Estimation
PROCEEDINGS OF THE 10TH ACM INTERNATIONAL CONFERENCE ON SYSTEMS FOR ENERGY-EFFICIENT BUILDINGS, CITIES, AND TRANSPORTATION, BUILDSYS 2023(2023)
摘要
Buildings are highly responsible for total energy consumption in cities; therefore, accurate estimation of building energy consumption is essential for developing energy-efficient strategies on an urban scale. Data-driven urban building energy models can predict energy use with high precision and low computational cost. In recent years, machine learning, especially neural networks, emerged as the prominent method for predicting energy load for buildings. These models typically use different input features on building form, occupancy and operation. However, they remain inadequate in capturing the complex inter-dependencies (i.e., heat transfer) between units in multi-zone buildings, as they do not have an explicit representation of the neighborhood relations between zones. The precision of data-driven models can be improved using Graph neural networks (GNN) that can capture the underlying relationships and dependencies between different building elements. In this paper we propose a novel GNN model that surpasses the current state-of-art methods for the prediction of zone-level heating energy use. We applied the methodology in a residential neighborhood consisting of 5866 buildings and 64462 zones. We use zone-level features regarding their geometry, material thermal characteristics, internal loads as node features, inter-zone parameters as edge features (total area and U value of the adjacent surfaces) and weather parameters. The results showed that our proposed model provides improvements over alternative approaches for precise prediction of urban building energy consumption.
更多查看译文
关键词
Urban Building Energy Modeling,Graph Neural Network,Urbanscale Building Energy Consumption
AI 理解论文
溯源树
样例
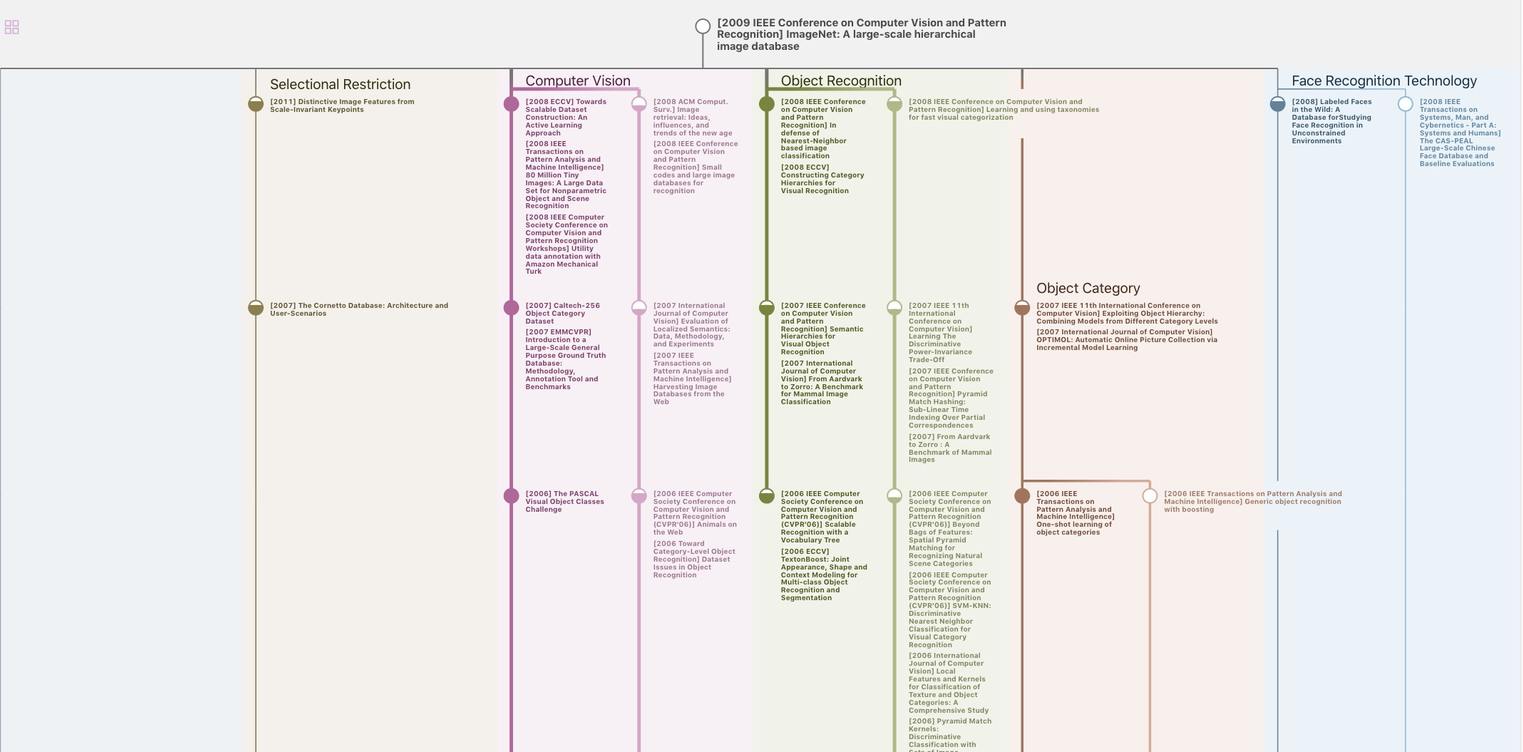
生成溯源树,研究论文发展脉络
Chat Paper
正在生成论文摘要