E-Patcher: A Patch-Based Efficient Network for Fast Whole Slide Images Segmentation
ARTIFICIAL NEURAL NETWORKS AND MACHINE LEARNING, ICANN 2023, PT II(2023)
摘要
UNeXt is a leading medical image segmentation method that employs convolutional and multi-layer perceptron (MLP) structure for its segmentation network. It outperforms other image recognition algorithms, such as MedT and TransUNet, in terms of faster computation speed and has shown great potential for clinical applications. However, its reliance on limited pixel neighborhood information for pixel-level segmentation of large pathological imagesmay lead to inaccurate segmentation of the entire image and overlook important features, resulting in suboptimal segmentation results. To this end, we designed a slight and universal plug-and-play block based patch-level for fully considering local features and global features simultaneously, named "Digging" and "Filling" ViT (DF-ViT) block. Specifically, a "Digging" operation is introduced to randomly select sub-blocks from each sub-patch. Multi-Head Attention (MHA) is then applied to integrate global information into these sub-blocks. The resulting sub-blocks with global semantic features are reassembled into the original feature map, and feature fusion is performed to combine the local and global features. This approach achieves global representation while maintaining a low computational complexity of the model at 0.1424G. Compared to UNeXt, it improves mIoU by 1.07%. Moreover, it reduces the parameter count by 58.50% and the computational workload by 68.09%. Extensive experiments on PAIP 2019 WSI dataset demonstrate that the DF-ViT block significantly enhances computation efficiency while maintaining a high level of accuracy.
更多查看译文
关键词
Efficient Transformer,Medical Image Segmentation,Whole Slide Images
AI 理解论文
溯源树
样例
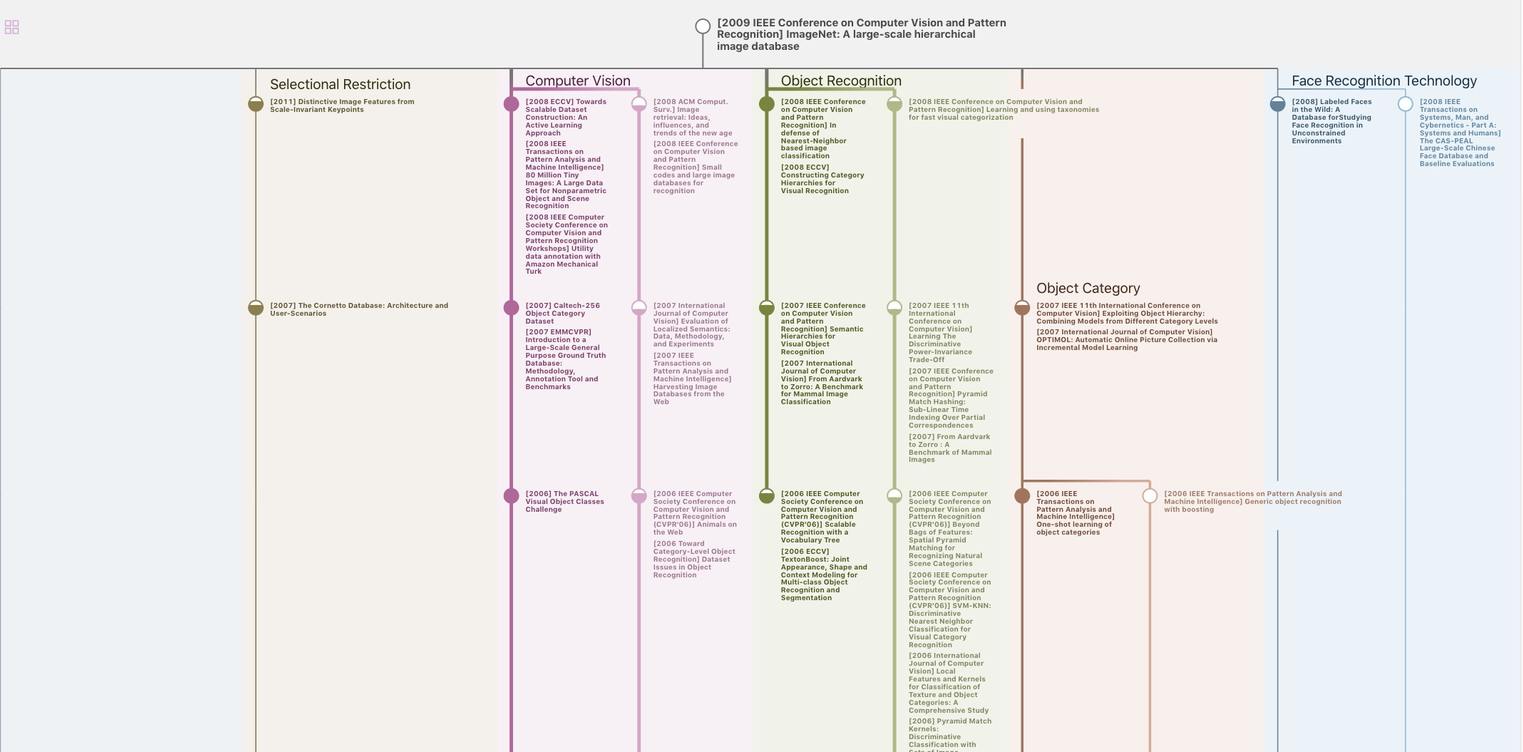
生成溯源树,研究论文发展脉络
Chat Paper
正在生成论文摘要