Contour-Augmented Concept Prediction Network for Image Captioning
ARTIFICIAL NEURAL NETWORKS AND MACHINE LEARNING, ICANN 2023, PT II(2023)
Abstract
Semantic information in images is essential for image captioning. However, previous works leverage the pre-trained object detector to mine semantics in an image, making the model unable to accurately capture visual semantics, and further making the generated descriptions irrelevant to the content of the given image. Thus, in this paper, we propose a Contour-augmented Concept Prediction Network (CCP-Net), which leverages two additional aspects of visual information, including high-level features (concepts) and low-level features (contours) in an end-to-end manner, to encourage the contribution of visual content in description generation. Furthermore, we propose a contour-augmented visual feature extraction module and equip it with elegantly designed feature fusion. Utilizing homogeneous contour features can better enhance visual feature extraction and further promote visual concept prediction. Extensive experimental results on MS COCO dataset demonstrate the effectiveness of our method and each proposed module, which can obtain 40.6 BLEU-4 and 135.6 CIDEr scores. Code will be released in the final version of the paper.
MoreTranslated text
Key words
Contour-augmented Feature Extraction,Joint Prediction,Concept Prediction,Image Captioning
AI Read Science
Must-Reading Tree
Example
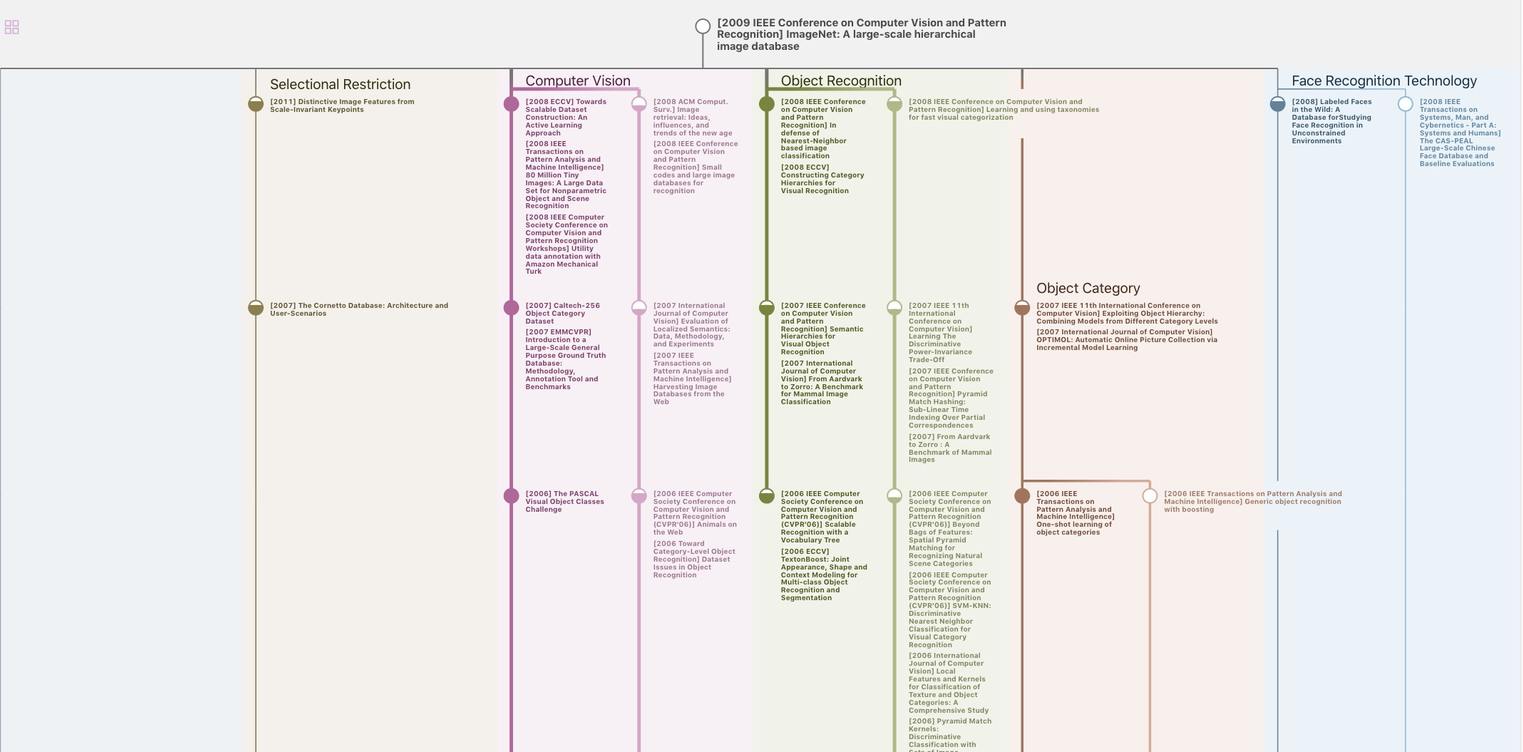
Generate MRT to find the research sequence of this paper
Chat Paper
Summary is being generated by the instructions you defined