Multi-label Image Deep Hashing with Hybrid Loss of Global Center and Local Alignment
ARTIFICIAL NEURAL NETWORKS AND MACHINE LEARNING, ICANN 2023, PART X(2023)
摘要
Deep hashing algorithms are widely used in large-scale image retrieval tasks. Based on deep neural network as the backbone, combined with the design of loss function, deep hashing can transform high-dimensional image inputs into binary hash codes, which better improves the efficiency of image retrieval and reduces the storage space. Most of the existing methods use image pairs or triplets for local similarity constraints, and recently hashing methods based on the global hash centers have been proposed. In this paper, we propose a novel deep hashing method that combines the global and local constraints in order to further improve the effect of deep hashing in image retrieval task. For multi-label images, we extend the global hash center generation method so that each image has multiple hash centers, represented by binary hash codes, with the same number of image categories. Then, multiple global hash central binary codes corresponding to the images are used as anchors, and dissimilar image pairs are selected to construct the triplet loss constraint linking global and local features. Moreover, we construct a partially similar loss function for the images where only part of the classification labels are similar, making more use of multiple labels. Furthermore, we combine the global and local loss functions and propose a novel hybrid loss function for multi-label image deep hashing. Extensive experiments on four multi-label image datasets for image retrieval demonstrate that the proposed method achieves substantial improvement over state-of-the-art hashing methods.
更多查看译文
关键词
Deep hashing,Multi-label image,Hybrid loss,Global center,Local alignment
AI 理解论文
溯源树
样例
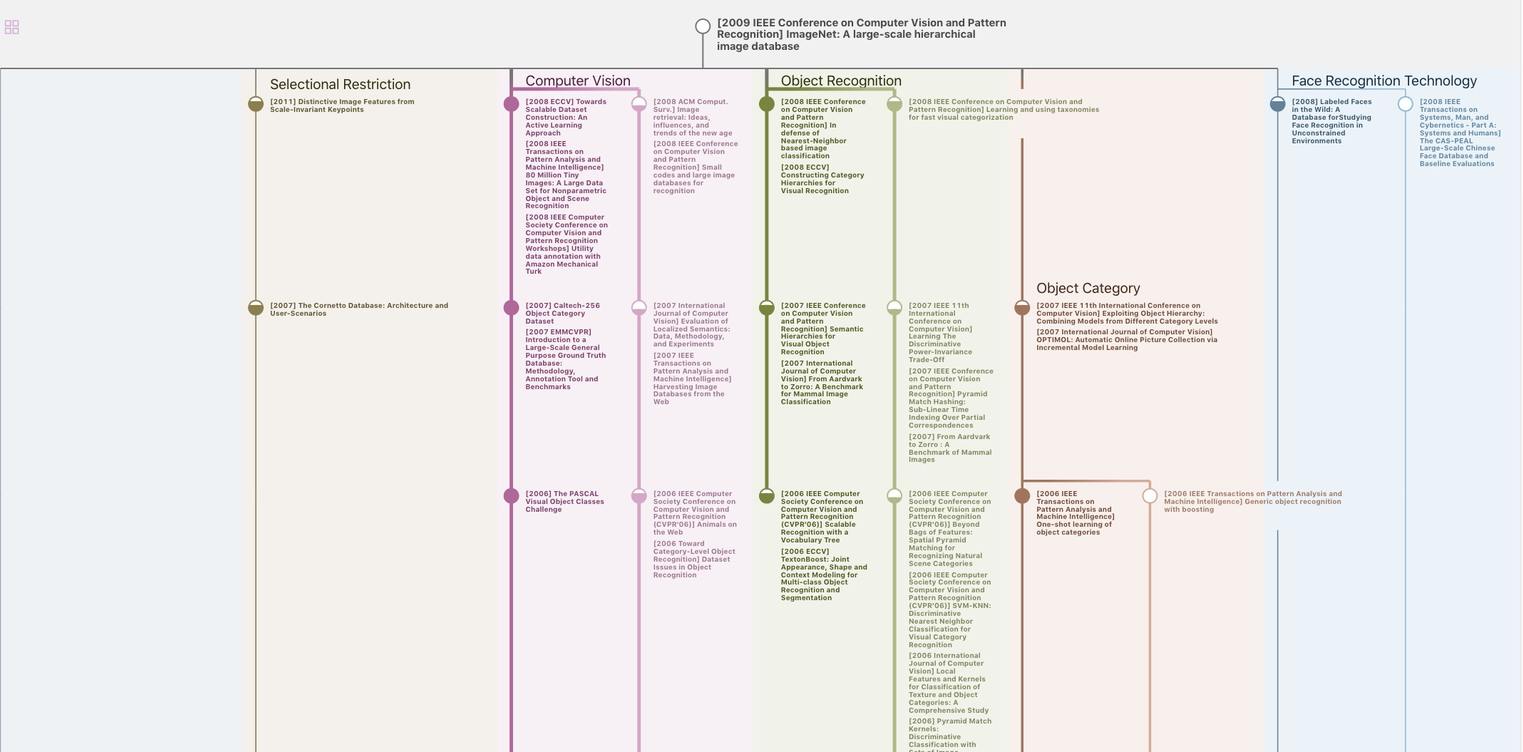
生成溯源树,研究论文发展脉络
Chat Paper
正在生成论文摘要