FeatEMD: Better Patch Sampling and Distance Metric for Few-Shot Image Classification
ARTIFICIAL NEURAL NETWORKS AND MACHINE LEARNING, ICANN 2023, PT I(2023)
摘要
Few-shot image classification (FSIC) studies the problem of classifying images when given only a few training samples, which presents a challenge for deep learning models to generalize well on unseen image categories. To learn FSIC tasks effectively, recent metric-based methods leverage the similarity measures of deep feature representations with minimum matching costs, introducing a new paradigm in addressing the FSIC challenge. Recent metric-learning techniques, e.g., DeepEMD, measure the distance between features with the earth mover's distance (EMD), and it is currently the state-of-the-art (SOTA) approach for FSIC. In this paper, we however identify two fundamental limitations in DeepEMD. First, it brings high computational cost, as it randomly samples image patches to extract features. This process is often wasteful due to suboptimal sampling strategies. Second, its accuracy is also limited by the use of optimal-transport costs based on cosine similarity, which only measures directional discrepancies. To mitigate the above shortcomings, we propose an improved method, which we call FeatEMD. First, it introduces a feature saliency-based cropping (FeatCrop) to construct image patches that concentrates computations on object-salient regions. Second, it proposes a Direction-Distance Similarity (DDS) a more effective distance criterion in capturing subtle differences in latent space features. We conduct comprehensive experiments and ablations to validate our method. Experimental results show FeatEMD establishes new SOTA on two mainstream benchmark datasets. Remarkably, when compared with DeepEMD, FeatEMD reduces up to 36% computational costs. Our code is available at https://github.com/SethDeng/FeatEMD.
更多查看译文
关键词
Few-shot image classification,metric learning,saliency-based cropping
AI 理解论文
溯源树
样例
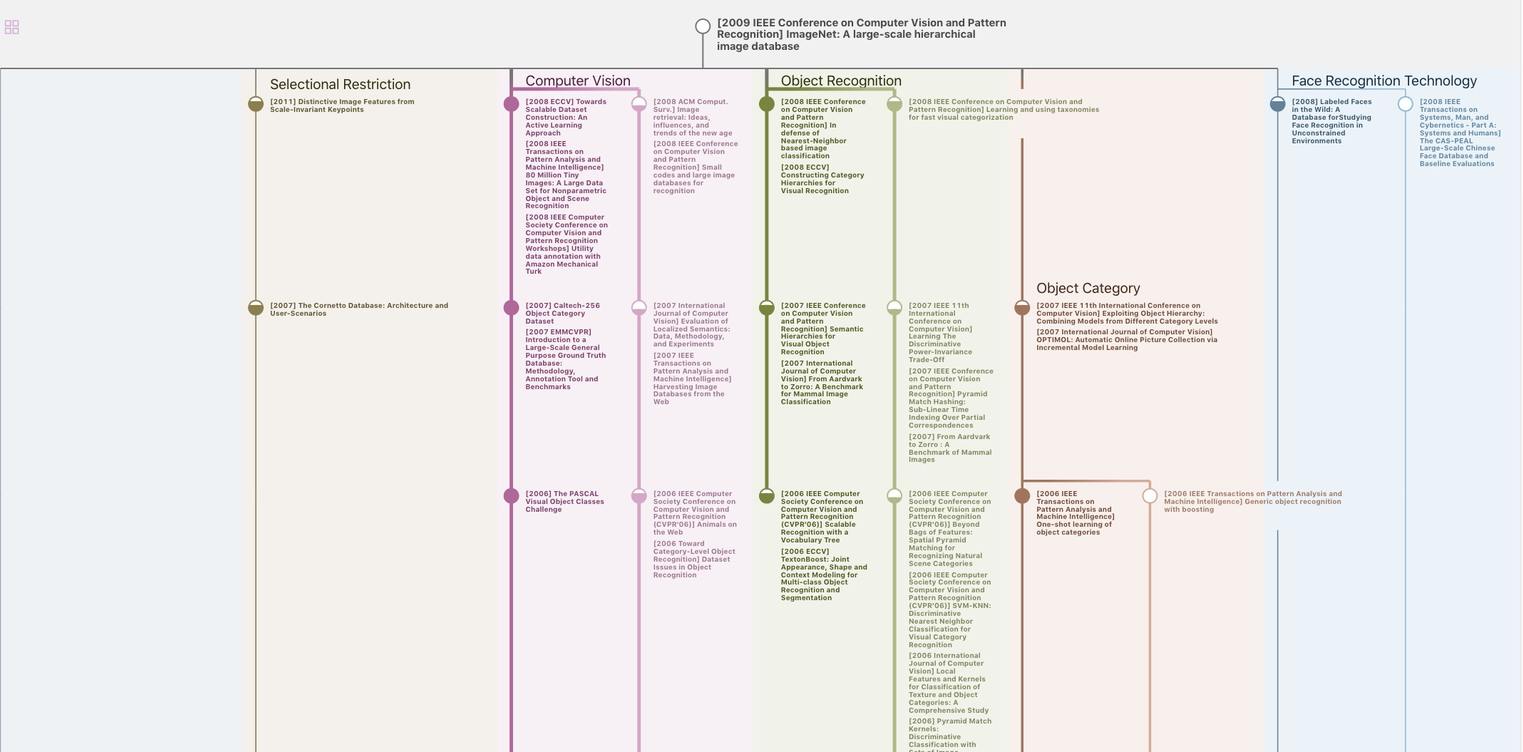
生成溯源树,研究论文发展脉络
Chat Paper
正在生成论文摘要