Enhancing Cross-Lingual Few-Shot Named Entity Recognition by Prompt-Guiding
ARTIFICIAL NEURAL NETWORKS AND MACHINE LEARNING, ICANN 2023, PT I(2023)
摘要
The cross-lingual named entity recognition task has attracted significant attention from researchers. Previous work has demonstrated that incorporating unlabeled data with potential entities in the target language can enhance cross-lingual model performance. However, unlabeled data for the target language is not always available, and the entity types may not share the same label space as the source language. To address this issue, we introduce a new NER task called cross-lingual few-shot NER. Distance metric learning has emerged as a popular solution for low-resource scenarios without semantic information for the target language. Inspired by few-shot metric learning and prompt learning, we propose a novel method called Cross-lingual Prompt-guiding Named Entity Recognition (CroPoNER) for this task. We use prompts from different languages to serve as 1) supervisory guidance for conveying unseen entity type information to the language model; 2) metric referents for predicting target language entity types; 3) a bridge between different languages that mitigates the language gap. Our experiments on several widely-used cross-lingual NER datasets (CoNLL, WikiAnn) in the few-shot setting demonstrate that our method outperforms state-of-the-art models by a significant margin in most cases for cross-lingual few-shot NER.
更多查看译文
关键词
Named Entity Recognition,Few-shot Learning,Cross-lingual,Metric Referent
AI 理解论文
溯源树
样例
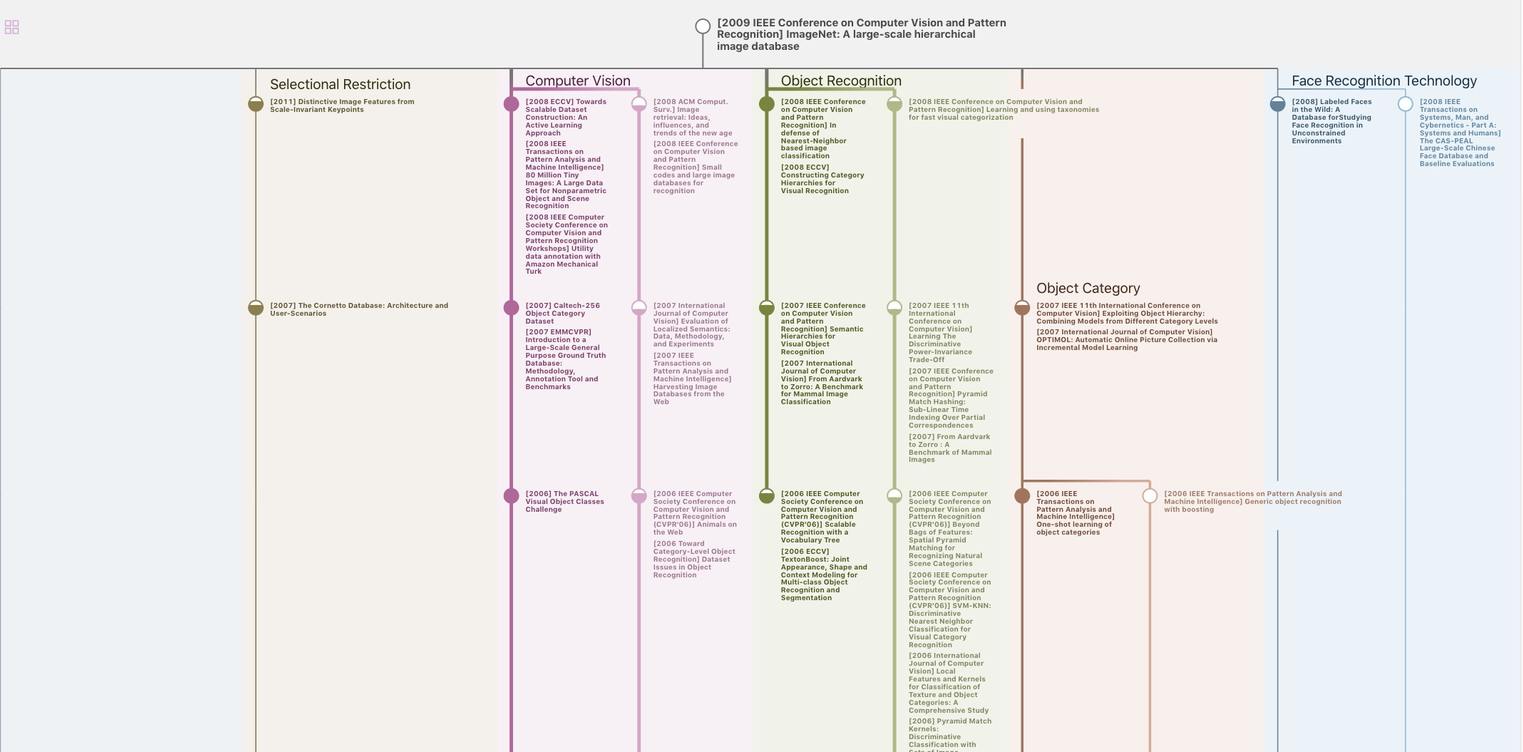
生成溯源树,研究论文发展脉络
Chat Paper
正在生成论文摘要