A Document-Level Relation Extraction Framework with Dynamic Pruning
ARTIFICIAL NEURAL NETWORKS AND MACHINE LEARNING, ICANN 2023, PT VIII(2023)
摘要
Relation extraction (RE) has been a fundamental task in natural language processing (NLP) as it identifies semantic relations among entity pairs in texts. Because sentence-level RE can only capture intra-connections within a sentence rather than inter-connections between or among sentences, researchers shift their attentions to document-level RE to obtain richer and complex relations which may involve logic inference. Prior works on document-level RE suffer from inflexible pruning rules and lack of sentence-level features, which lead to the missing of valuable information. In this paper, we propose a document-level relation extraction framework with both dynamic pruning mechanism and sentence-level attention. Specifically, a weight-based flexible pruning mechanism is applied on the document-level dependency tree to remove non-relational edges dynamically and obtain the weight dependency tree (WDT). Moreover, a graph convolution network (GCN) then is employed to learn syntactic representations of the WDT. Furthermore, the sentence-level attention and gating selection module are applied to capture the intrinsic interactions between sentence-level and document-level features. We evaluate our framework on three benchmark datasets: DocRED, CDR, and GDA. Experiment results demonstrate that our approach outperforms the baselines and achieves the state-of-the-art performance.
更多查看译文
关键词
Document-level Relation Extraction,Dynamic pruning mechanism,sentence-level attention,gating selection
AI 理解论文
溯源树
样例
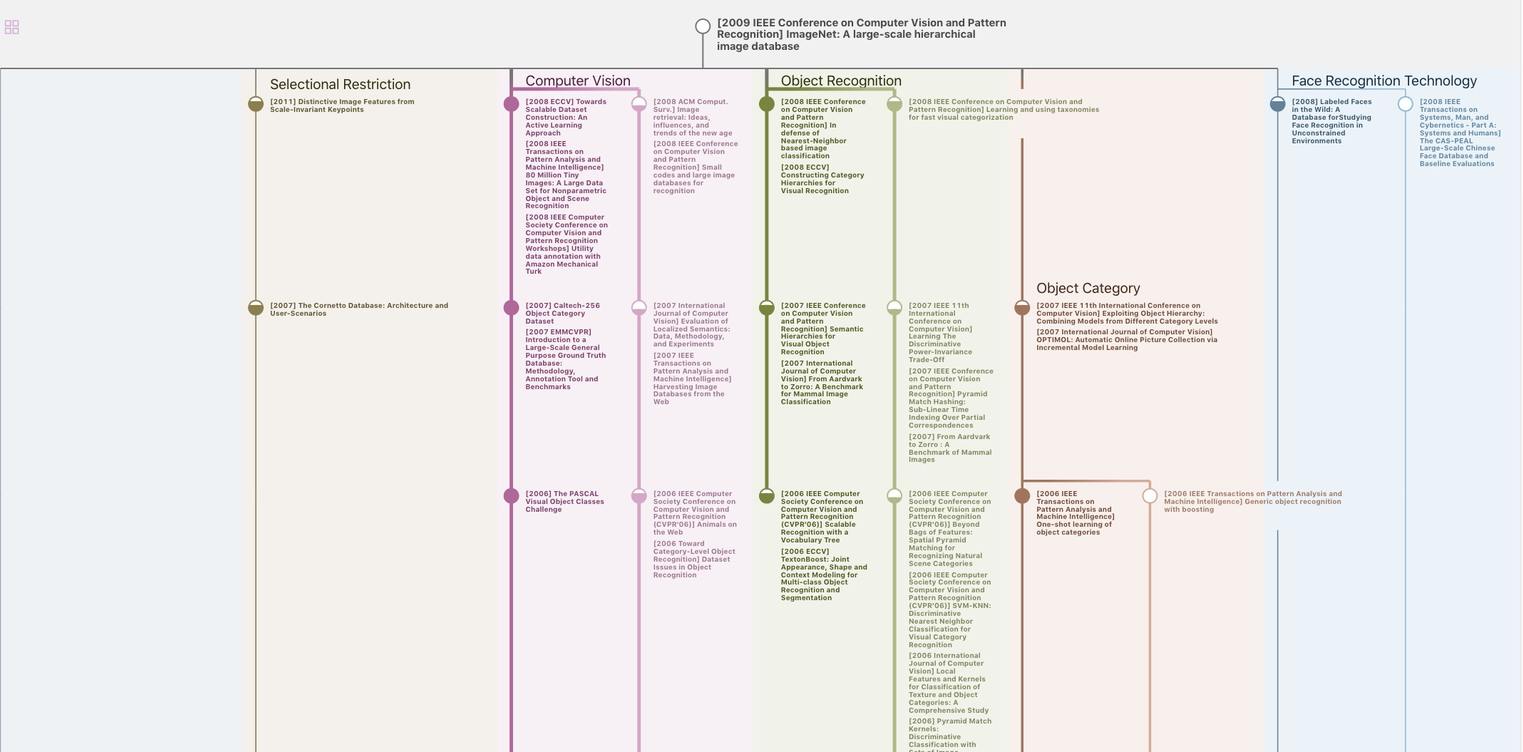
生成溯源树,研究论文发展脉络
Chat Paper
正在生成论文摘要