Low-Frequency Features Optimization for Transferability Enhancement in Radar Target Adversarial Attack
ARTIFICIAL NEURAL NETWORKS AND MACHINE LEARNING, ICANN 2023, PT V(2023)
摘要
Ensuring the transferability of adversarial examples between different recognition models is one of the keys to radar target adversarial attack technology. Analyzing the common transfer principles of different perturbations in various radar target recognition models is an important method to improve the transferability of adversarial examples. The features of radar targets can be divided in frequency domain. The high-frequency features are affected by the flicker phenomenon and have low stability, while the low-frequency features reflect the main structure of the target and have strong generalizations. Given the characteristic, this paper proposes a semantic-inspired synthetic aperture radar target adversarial attack algorithm based on low-frequency optimization and feature bias. By introducing low-frequency optimization in the process of perturbation generation, the generated adversarial examples focus on the low-frequency features of attacked targets, which are more generalized. The adversarial examples are guided to attack the high-level semantic features of the target, and the transferability of adversarial examples is improved. Experimental results on moving and stationary target acquisition and recognition (MSTAR) dataset show the effectiveness of the proposed method.
更多查看译文
关键词
Radar target recognition,Adversarial attack,Feature selection
AI 理解论文
溯源树
样例
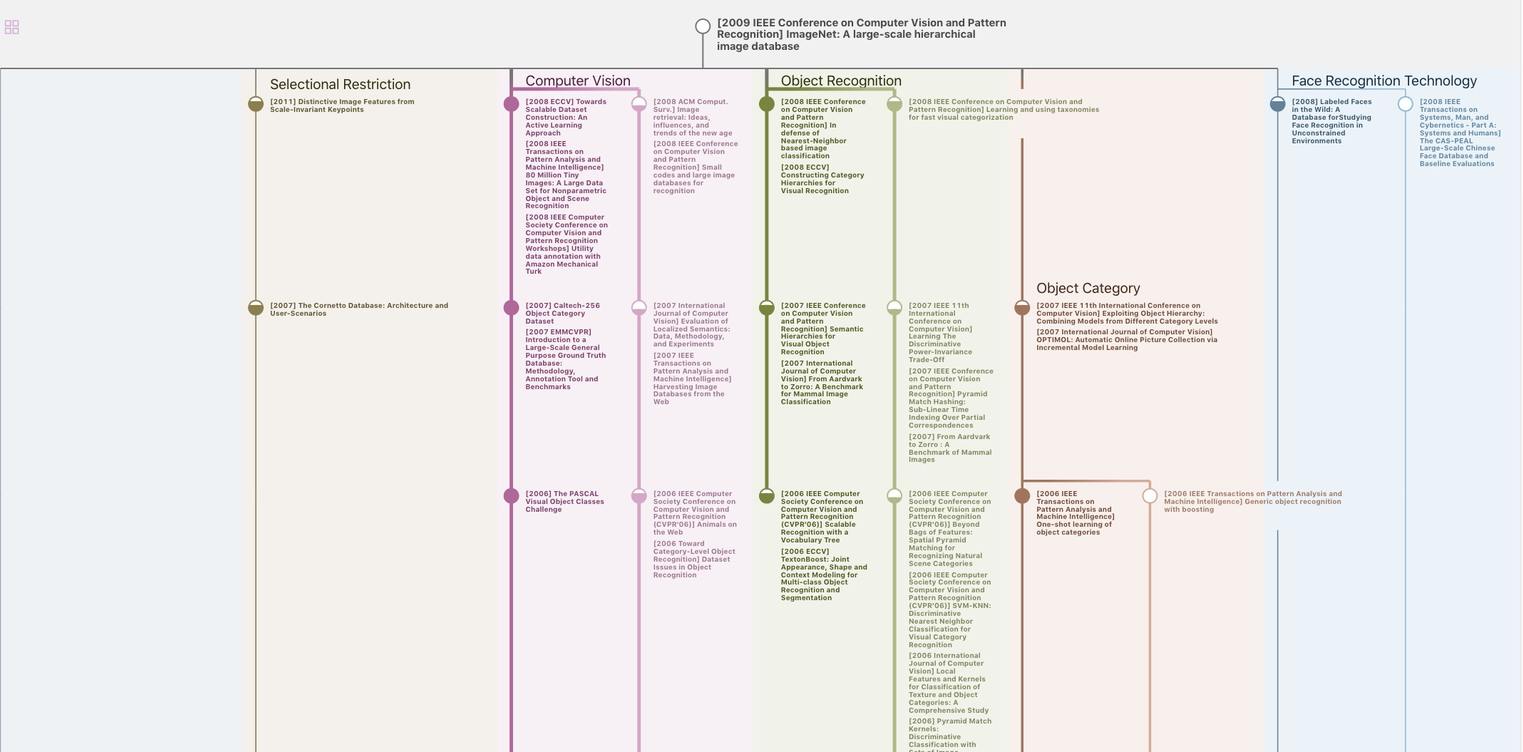
生成溯源树,研究论文发展脉络
Chat Paper
正在生成论文摘要