Low Complexity Adaptive ML Approaches for End-to-End Latency Prediction
Machine Learning for Networking: 5th International Conference, MLN 2022, Paris, France, November 28–30, 2022, Revised Selected Papers(2023)
摘要
Software Defined Networks have opened the door to statistical and AI-based techniques to improve efficiency of networking. Especially to ensure a certain Quality of Service (QoS) for specific applications by routing packets with awareness on content nature (VoIP, video, files, etc.) and its needs (latency, bandwidth, etc.) to use efficiently resources of a network. Monitoring and predicting various Key Performance Indicators (KPIs) at any level may handle such problems while preserving network bandwidth. The question addressed in this work is the design of efficient, low-cost adaptive algorithms for KPI estimation, monitoring and prediction. We focus on end-to-end latency prediction, for which we illustrate our approaches and results on data obtained from a public generator provided after the recent international challenge on GNN [ 12 ]. In this paper, we improve our previously proposed low-cost estimators [ 6 ] by adding the adaptive dimension, and show that the performances are minimally modified while gaining the ability to track varying networks.
更多查看译文
关键词
latency,prediction,complexity,end-to-end
AI 理解论文
溯源树
样例
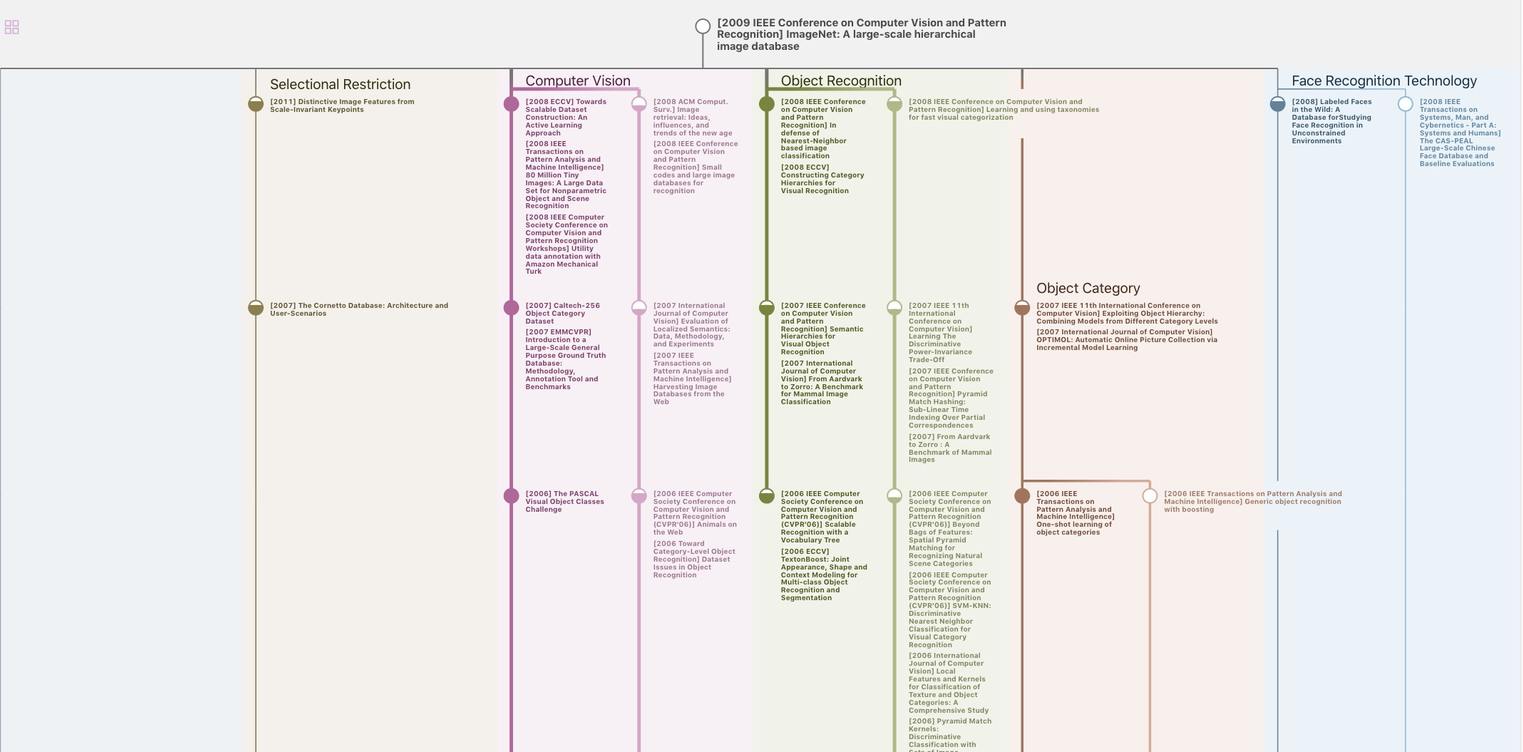
生成溯源树,研究论文发展脉络
Chat Paper
正在生成论文摘要