Reveal flocking phase transition of self-propelled active particles by machine learning regression uncertainty
ACTA PHYSICA SINICA(2023)
摘要
We develop the neural network based "learning from regression uncertainty" approach for the automatic detection of phases of matter in nonequilibrium active systems. Taking the flocking phase transition of selfpropelled active particles described by the Vicsek model for example, we find that after training a neural network for solving the inverse statistical problem, i.e. for performing the regression task of reconstructing the noise level from given samples of such a nonequilibrium many-body complex system's steady state configurations, the uncertainty of regression results obtained by the well-trained network can actually be utilized to reveal possible phase transitions in the system under study. The noise level dependence of regression uncertainty is assumed to be in a non-trivial M-shape, and its valley appears at the critical point of the flocking phase transition. By directly comparing this regression-based approach with the widely-used classification-based "learning by confusion" and "learning with blanking" approaches, we show that our approach has practical effectiveness, efficiency, good generality for various physical systems across interdisciplinary fields, and a greater possibility of being interpretable via conventional notions of physics. These approaches can complement each other to serve as a promising generic toolbox for investigating rich critical phenomena and providing data driven evidence on the existence of various phase transitions, especially for those complex scenarios associated with first-order phase transitions or nonequilibrium active systems where traditional research methods in physics could face difficulties.
更多查看译文
关键词
machine learning,phase transition,nonequilibrium many-body system,inverse statistical problem
AI 理解论文
溯源树
样例
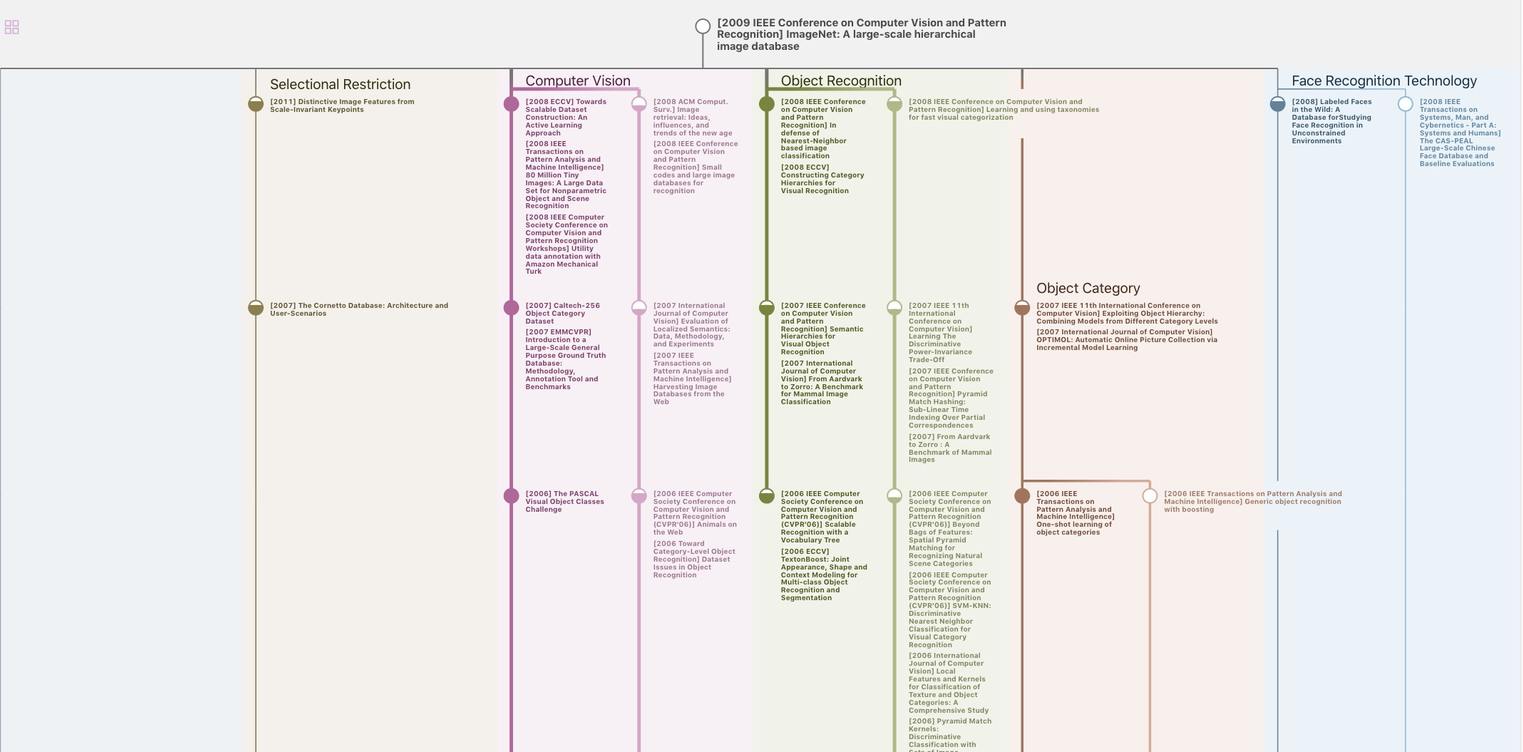
生成溯源树,研究论文发展脉络
Chat Paper
正在生成论文摘要