Attention-Based Global-Local Graph Learning for Dynamic Facial Expression Recognition.
Image and Graphics: 12th International Conference, ICIG 2023, Nanjing, China, September 22–24, 2023, Proceedings, Part I(2023)
摘要
Previous methods for dynamic facial expression recognition cannot jointly capture discriminative global and local features and leverage facial structural information to better understand expressions in videos with occlusions, variant poses and poor illumination. In this paper, we propose a novel global-local graph-learning method based on attention mechanisms to conduct robust recognition for these challenging cases. First, we extract high-level features from each frame by a CNN backbone. Then, to holistically describe dynamic expressions, we construct a global spatial-temporal graph (GSTG) by regarding each patch of the output feature maps as a graph node and initializing spatial and temporal connections. We employ multi-head self-attention to capture the long-range spatiotemporal relations and dynamically optimizes GSTG to generate discriminative descriptions. To capture dominant local information, we construct a local spatial-temporal graph (LSTG) by extracting intermediate CNN features of local regions based on landmark-guided attention and defining their geometric relationships. We utilize topology-learnable ST-GCNs to exploit the local dynamics and implicit relations. Finally, we design a global-local fusion unit at decision level, which adaptively controls the importance of each feature element. The proposed method achieves competitive performance on Oulu-CASIA and AFEW datasets.
更多查看译文
关键词
facial expression recognition,graph,attention-based,global-local
AI 理解论文
溯源树
样例
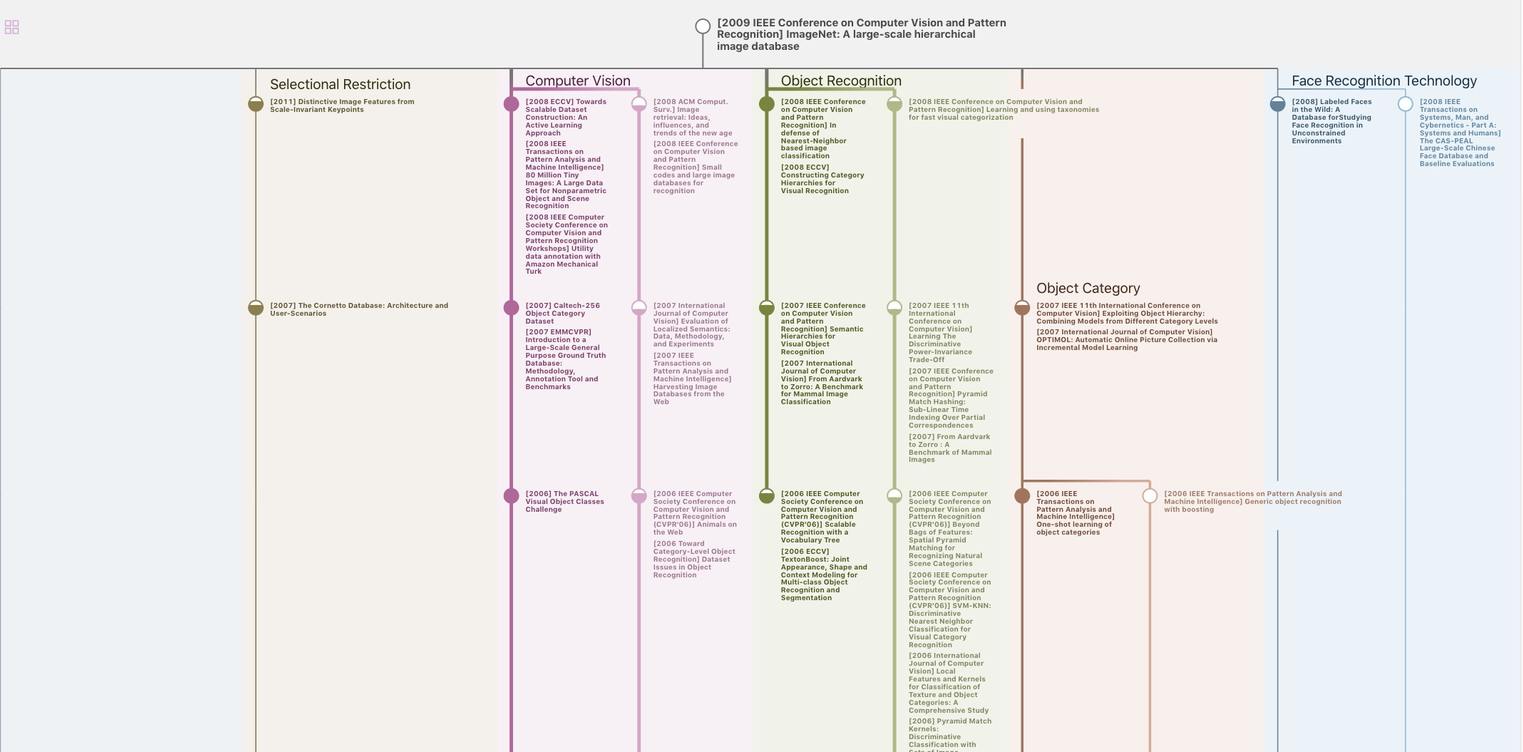
生成溯源树,研究论文发展脉络
Chat Paper
正在生成论文摘要