Graph Neural Network-Based Representation Learning for Medical Time Series
ARTIFICIAL NEURAL NETWORKS AND MACHINE LEARNING, ICANN 2023, PT VI(2023)
摘要
The ability to analyze and predict medical time series data is crucial for enhancing healthcare decision-making and improving patient outcomes. Currently, the algorithms used for classification and prediction of medical time series data are limited in their capabilities and may not be reliable enough to meet the demands of practical applications. The purpose of this paper is to promote the representation learning of complex data primarily comprised of medical time series, in order to facilitate various downstream tasks. Under the framework of graph neural networks (GNN), we present indegree regularized neural message passing to reflect the dependencies between different sequences. Our approach also leverages representation learning to convert multivariate time series (MTS) and static features into nodes of GNN. Moreover, we propose a dynamic loss function to encourage the consistent learning of sensor dependency graphs across models. Based on these proposals, our method can effectively capture not only the temporal dependencies among variables, but also the multidimensional dependencies among MTS and static features. We classify time series on two medical challenge and a human activity datasets. The results show that our approach can significantly improve downstream task performance across various metrics. Code is available at https://github.com/Zzzoptimus/GICG.
更多查看译文
关键词
Graph neural network,Medical time series,Regularized neural message passing,Time series classification
AI 理解论文
溯源树
样例
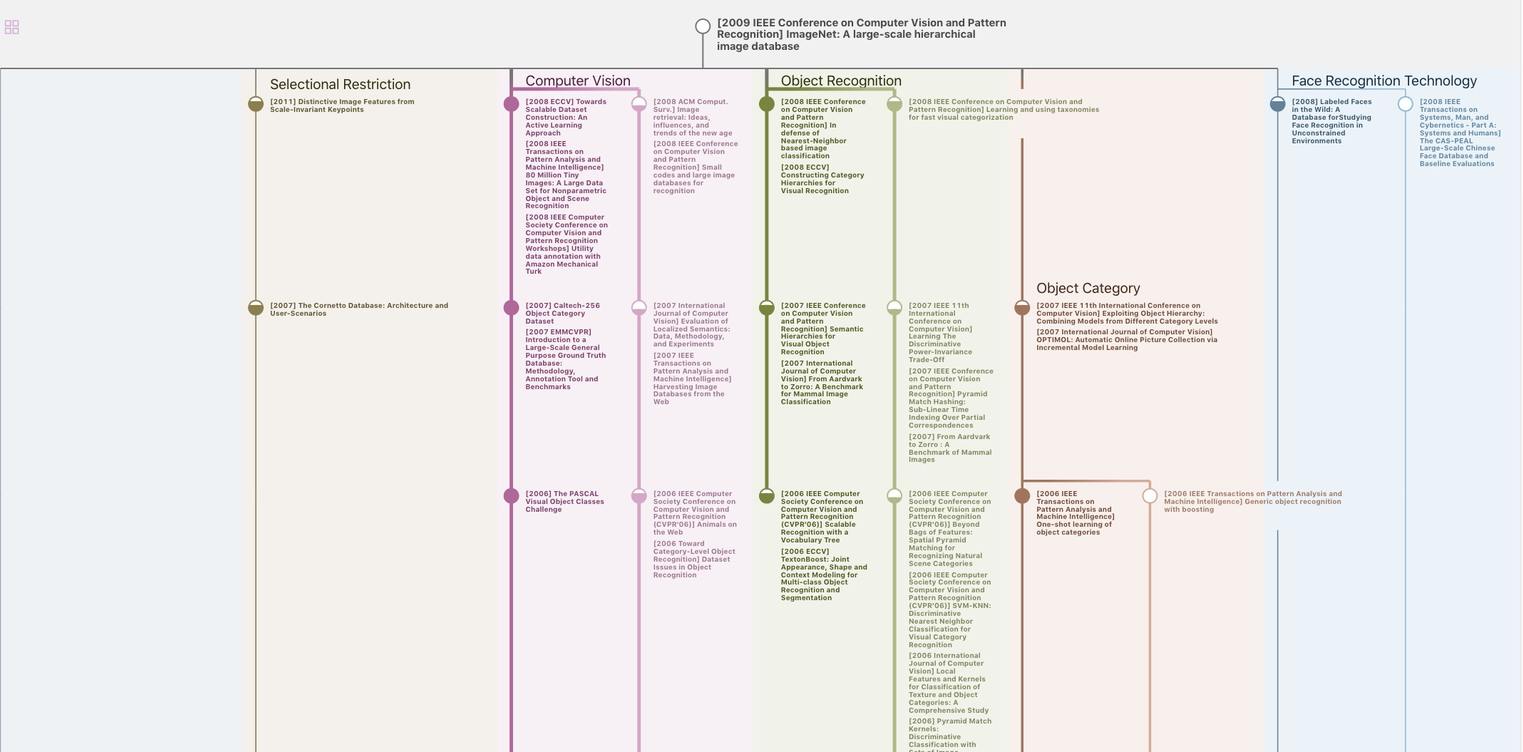
生成溯源树,研究论文发展脉络
Chat Paper
正在生成论文摘要