A Novel State Space Exploration Method for the Sparse-Reward Reinforcement Learning Environment
ARTIFICIAL INTELLIGENCE XL, AI 2023(2023)
摘要
Sparse-reward reinforcement learning environments pose a particular challenge because the agent receives infrequent rewards, making it difficult to learn an optimal policy. In this paper, we propose NSSE, a novel approach that combines that stratified state space exploration with prioritised sweeping to enhance the informativeness of learning, thus enabling fast learning convergence. We evaluate NSSE on three typical Atari sparse reward environments. The results demonstrate that our state space exploration method exhibits strong performance compared to two baseline algorithms: Deep Q-Network (DQN) and noisy Deep Q-Network (Noisy DQN).
更多查看译文
关键词
Sparse-reward,Replay Sub-buffers,DQN,Exploration,Reinforcement Learning
AI 理解论文
溯源树
样例
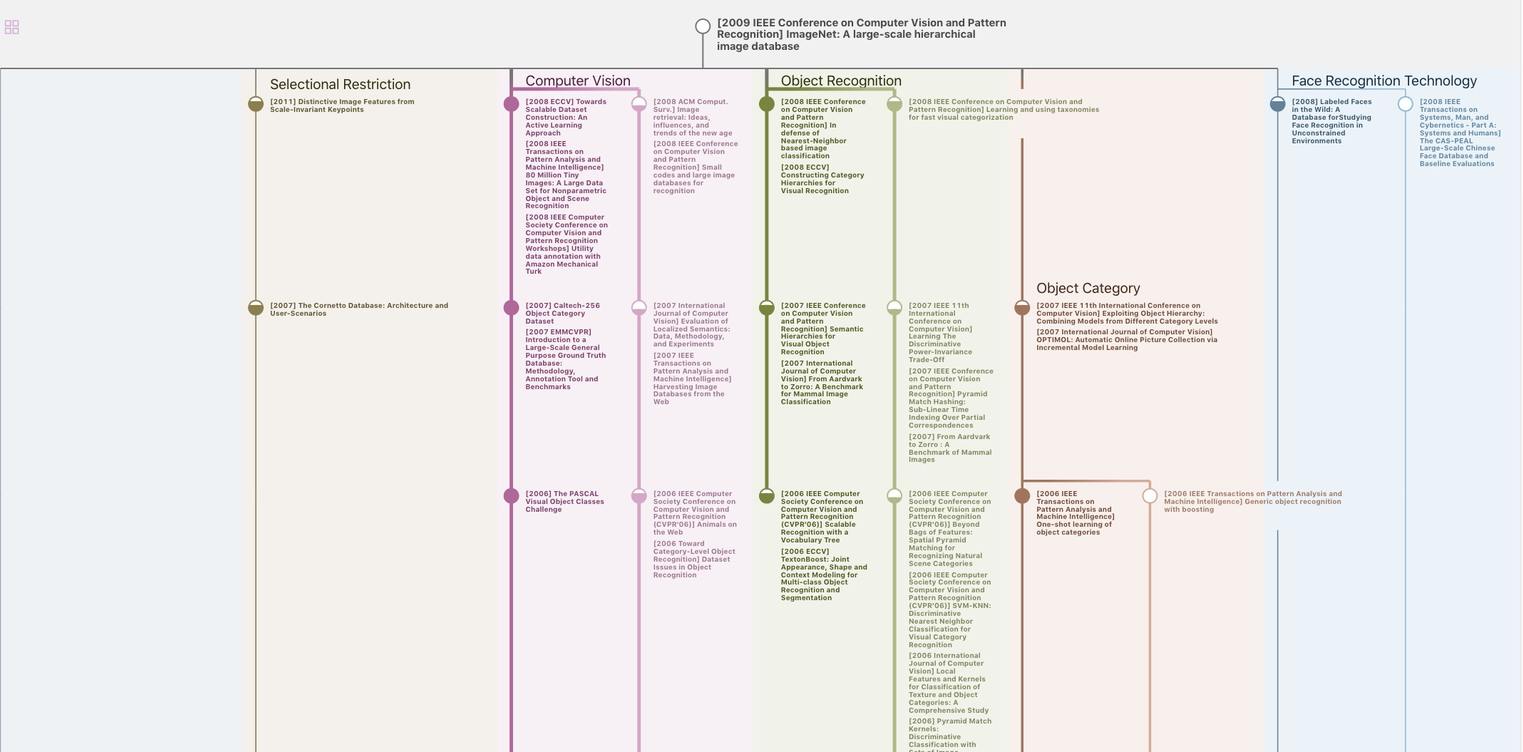
生成溯源树,研究论文发展脉络
Chat Paper
正在生成论文摘要