Dosimetric Evaluation of U-Net Deep Learning-Based Synthetic Computed Tomography for Adaptive Radiation Treatment Planning
2023 15th Biomedical Engineering International Conference (BMEiCON)(2023)
摘要
In cancer treatment, radiation therapy plays a vital role, employing advanced techniques to precisely target cancer cells while minimizing harm to healthy tissues. However, these techniques are sensitive to uncertainties in delivering radiation doses. To address the challenges posed by treatment complexity and anatomical changes, adaptive radiotherapy (ART) modifies treatment plans based on patient anatomical changes, utilizing kilovoltage cone-beam computed tomography (kV-CBCT) images. However, directly using kV-CBCT for patient alignment is not feasible due to limitations in CT number inconsistencies, image quality, and limited field of view, necessitating kV-CBCT correction. Thus, this study aims to integrate the 2D U-Net algorithm for generating synthetic CT (sCT) images for radiation therapy planning in head and neck cancer, with a focus on dosimetric comparison. The results demonstrate that sCT images effectively reduce artifacts and closely resemble planning CT (pCT) images in CT number (Hounsfield units). In the high-dose region, sCT outperforms kV-CBCT images. For density-based structures, sCT performs comparably to pCT for bone regions, with some variations observed in a kV-CBCT-based plan. Regarding clinical targets and organs at risk, sCT exhibits comparable dosimetric performance to pCT, while differences are observed with kV-CBCT. The study highlights the potential of the 2D U-Net algorithm for generating sCT images in adaptive radiation therapy for head and neck cancer, encouraging further research to optimize and validate its use in clinical practice.
更多查看译文
关键词
Synthetic CT,Deep learning,Dosimetric comparison,Cone beam computed tomography
AI 理解论文
溯源树
样例
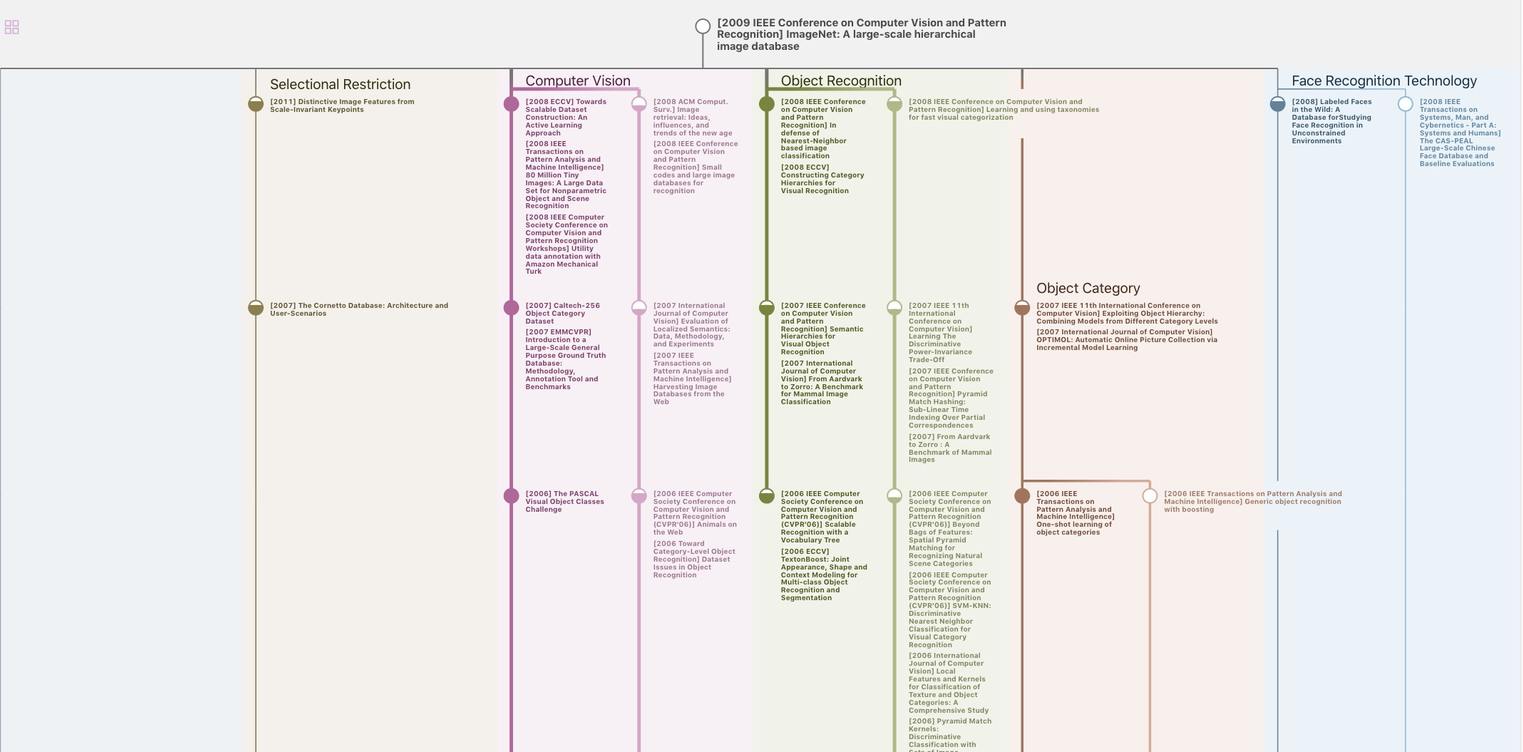
生成溯源树,研究论文发展脉络
Chat Paper
正在生成论文摘要